REST: Drug-Drug Interaction Prediction via Reinforced Student-Teacher Curriculum Learning
PROCEEDINGS OF THE 32ND ACM INTERNATIONAL CONFERENCE ON INFORMATION AND KNOWLEDGE MANAGEMENT, CIKM 2023(2023)
摘要
Accurate prediction of drug-drug interaction (DDI) is crucial to achieving effective decision-making in medical treatment for both doctors and patients. Recently, many deep learning based methods have been proposed to learn from drug-related features and conduct DDI prediction. These works have achieved promising results. However, the extreme imbalance of medical data poses a serious problem to DDI prediction, where a small fraction of DDI types occupy the majority training data. A straightforward way is to develop an appropriate policy to sample the data. Due to the high complexity and speciality of medical science, a dynamic learnable policy is required instead of a heuristic, uniform or static one. Therefore, we propose a REinforced Student-Teacher curriculum learning model (REST) for effective sampling to tackle this imbalance problem. Specifically, REST consists of two interactive parts, which are a heterogeneous graph neural network as the student and a reinforced sampler as the teacher. In each interaction, the teacher model takes action to sample an appropriate batch to train the student model according to the student model state while the cumulated improvement in performance of the student model is treated as the reward for policy gradient of the teacher model. The experimental results on two benchmarking datasets have demonstrated the significant effectiveness of our proposed model in DDI prediction, especially for the DDI types with low frequency.
更多查看译文
关键词
drug-drug interaction,curriculum learning,reinforcement learning,heterogeneous graph neural network
AI 理解论文
溯源树
样例
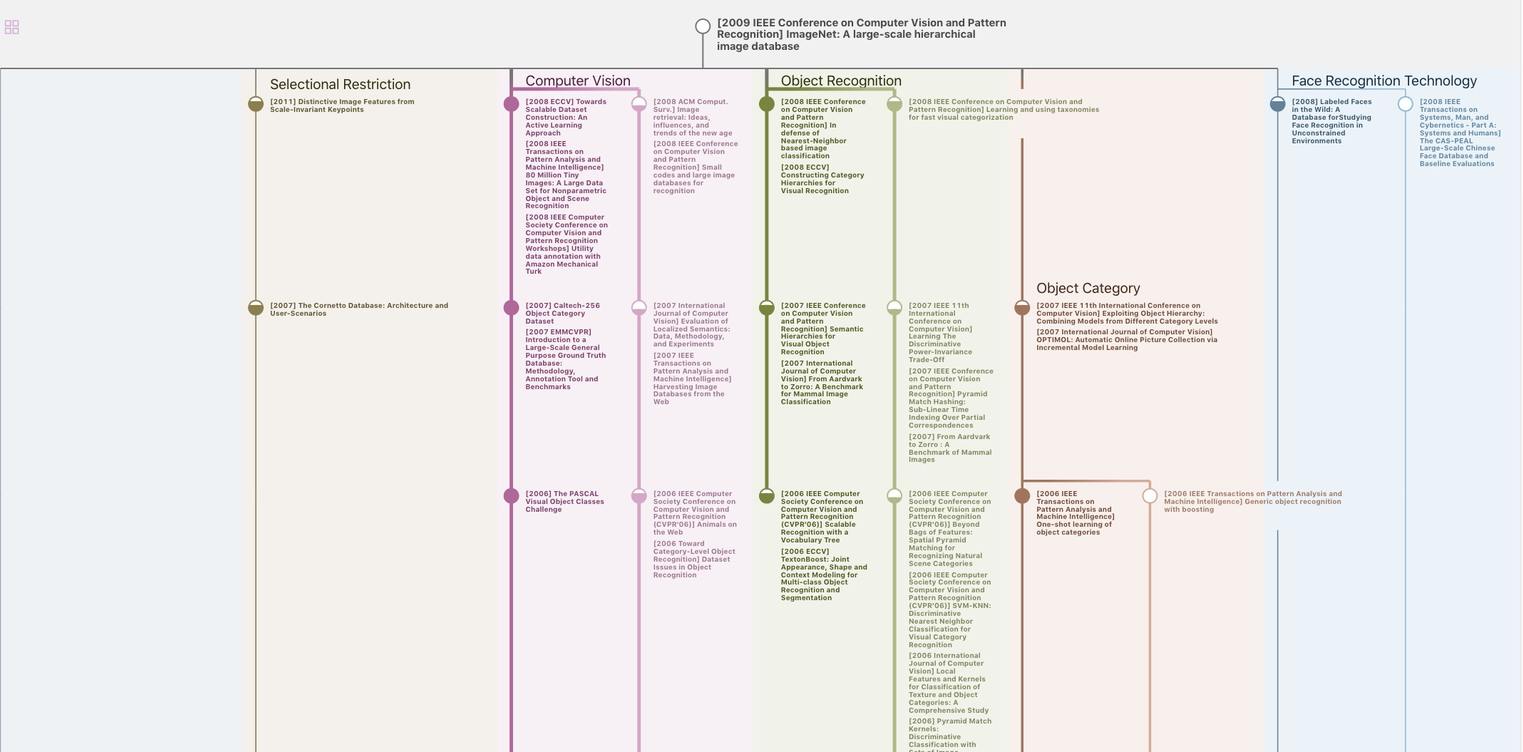
生成溯源树,研究论文发展脉络
Chat Paper
正在生成论文摘要