THGNN: An Embedding-based Model for Anomaly Detection in Dynamic Heterogeneous Social Networks
PROCEEDINGS OF THE 32ND ACM INTERNATIONAL CONFERENCE ON INFORMATION AND KNOWLEDGE MANAGEMENT, CIKM 2023(2023)
摘要
Anomaly detection, particularly the detection of anomalous behaviors in dynamic and heterogeneous social networks, is becoming more and more crucial in real life. Traditional rule-based and feature-based methods cannot well capture the structural and temporal patterns of ever-changing user behaviors. Moreover, most of the existing works based on network embedding either rely on discretized snapshots, which have ignored accurate temporal relations among user behaviors and weakened the impact of new edges, or fail to utilize dynamic and heterogeneous information simultaneously to distinguish varying effects of new edges on existing nodes. In this paper, we propose an end-to-end continuous-time model, named Temporal Heterogeneous Graph Neural Network (THGNN), to detect anomalous behaviors (edges) in dynamic heterogeneous social networks. Specifically, the model constantly updates node embeddings by propagating the information of a new edge to its source and target nodes as well as their neighbors. In this process, heterogeneous encoders are employed to handle different types of nodes and edges. What is more, a novel dual-level distributive attention mechanism is designed to allocate the influence degree of a currently interacting node to its multiple neighbors, considering the combined effect of edge type and time interval information. That can be regarded as an extension of the classical aggregative attention mechanism in the opposite direction. Extensive experiments on four real-world datasets demonstrate that THGNN outperforms all the baselines on the task of anomalous edge detection, achieving an average AUC gain of 6% across all datasets.
更多查看译文
关键词
anomaly detection,dynamic heterogeneous social networks,network embedding,dual-level attention,distributive attention
AI 理解论文
溯源树
样例
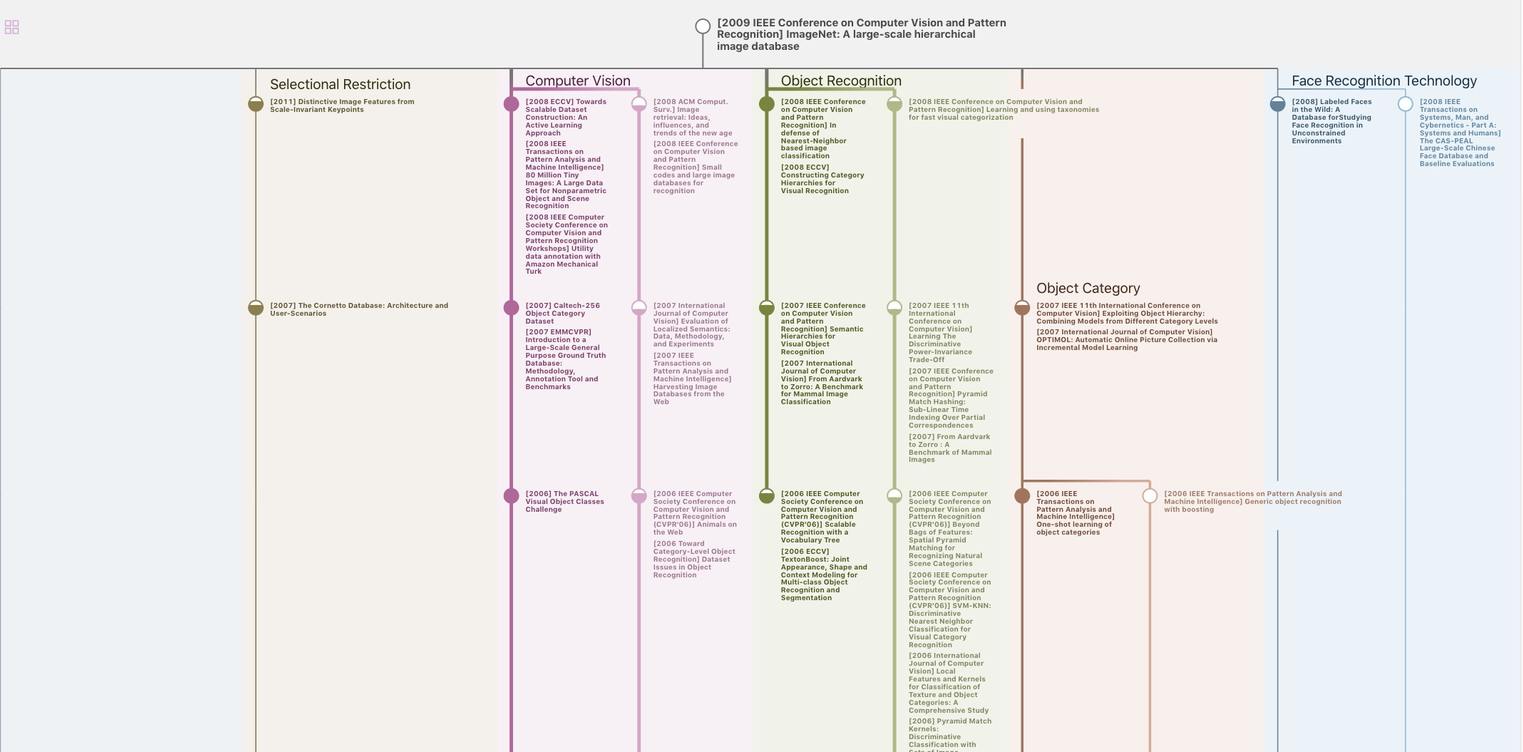
生成溯源树,研究论文发展脉络
Chat Paper
正在生成论文摘要