Multi-scale Graph Pooling Approach with Adaptive Key Subgraph for Graph Representations
PROCEEDINGS OF THE 32ND ACM INTERNATIONAL CONFERENCE ON INFORMATION AND KNOWLEDGE MANAGEMENT, CIKM 2023(2023)
摘要
The recent progress in graph representation learning boosts the development of many graph classification tasks, such as protein classification and social network classification. One of the mainstream approaches for graph representation learning is the hierarchical pooling method. It learns the graph representation by gradually reducing the scale of the graph, so it can be easily adapted to large-scale graphs. However, existing graph pooling methods discard the original graph structure during downsizing the graph, resulting in a lack of graph topological structure. In this paper, we propose a multi-scale graph neural network (MSGNN) model that not only retains the topological information of the graph but also maintains the key-subgraph for better interpretability. MSGNN gradually discards the unimportant nodes and retains the important subgraph structure during the iteration. The key subgraphs are first chosen by experience and then adaptively evolved to tailor specific graph structures for downstream tasks. The extensive experiments on seven datasets show that MSGNN improves the SOTA performance on graph classification and better retains key subgraphs.
更多查看译文
关键词
Graph representation learning,Graph classification,Graph pooling,Graph neural network,Key-subgraph
AI 理解论文
溯源树
样例
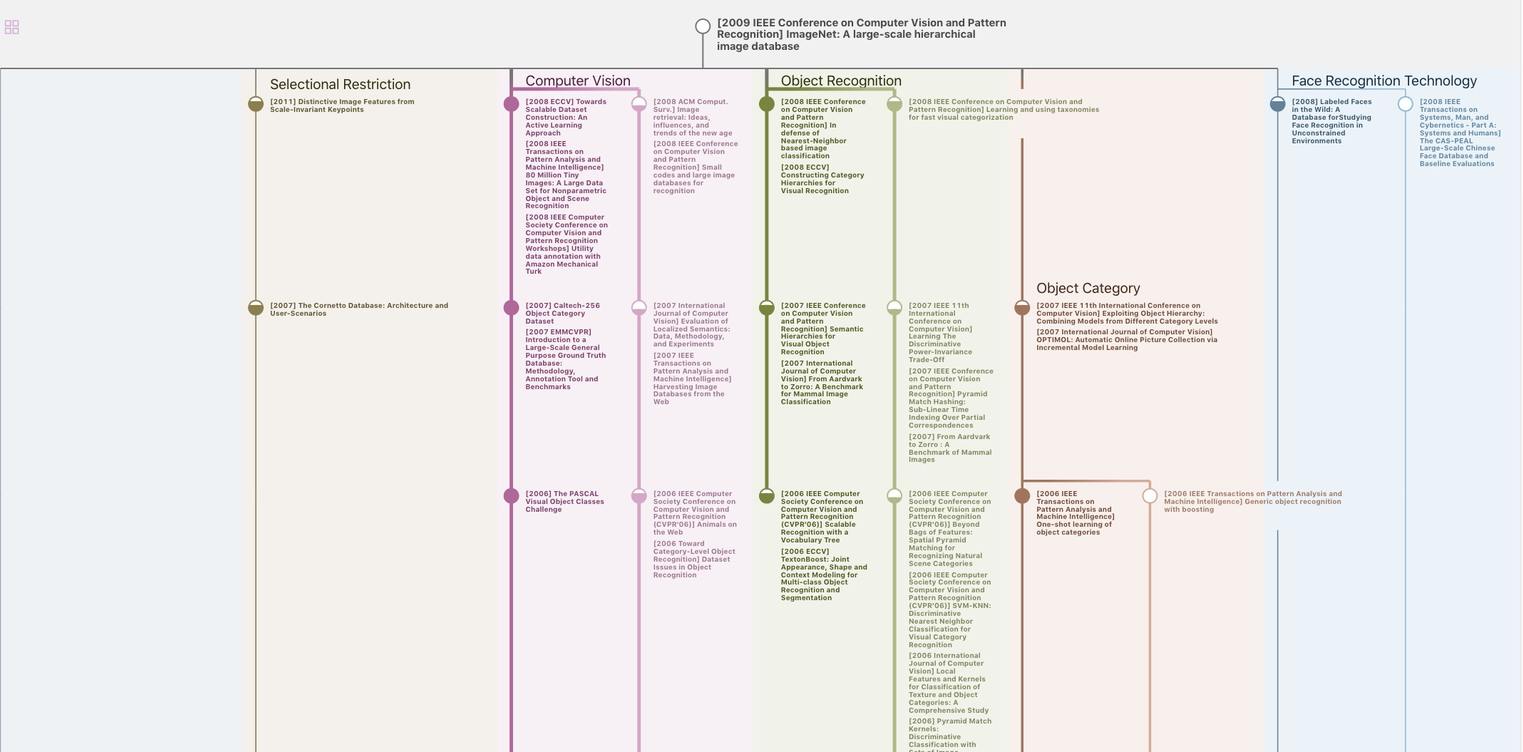
生成溯源树,研究论文发展脉络
Chat Paper
正在生成论文摘要