LambdaRank Gradients are Incoherent
PROCEEDINGS OF THE 32ND ACM INTERNATIONAL CONFERENCE ON INFORMATION AND KNOWLEDGE MANAGEMENT, CIKM 2023(2023)
摘要
In Information Retrieval (IR), the Learning-to-Rank (LTR) task requires building a ranking model that optimises a specific IR metric. One of the most effective approaches to do so is the well-known LambdaRank algorithm. LambdaRank uses gradient descent optimisation, and at its core, it defines approximate gradients, the so-called lambdas, for a non-differentiable IR metric. Intuitively, each lambda describes how much a document's score should be "pushed" up/down to reduce the ranking error. In this work, we show that lambdas may be incoherent w.r.t. the metric being optimised: e.g., a document with high relevance in the ground truth may receive a smaller gradient push than a document with lower relevance. This behaviour goes far beyond the expected degree of approximation. We analyse such behaviour of LambdaRank gradients and we introduce some strategies to reduce their incoherencies. We demonstrate through extensive experiments, conducted using publicly available datasets, that the proposed approach reduces the frequency of the incoherencies in LambdaRank and derivatives, and leads to models that achieve statistically significant improvements in the NDCG metric, without compromising the training efficiency.
更多查看译文
关键词
Information Retrieval,Learning to Rank,LambdaRank
AI 理解论文
溯源树
样例
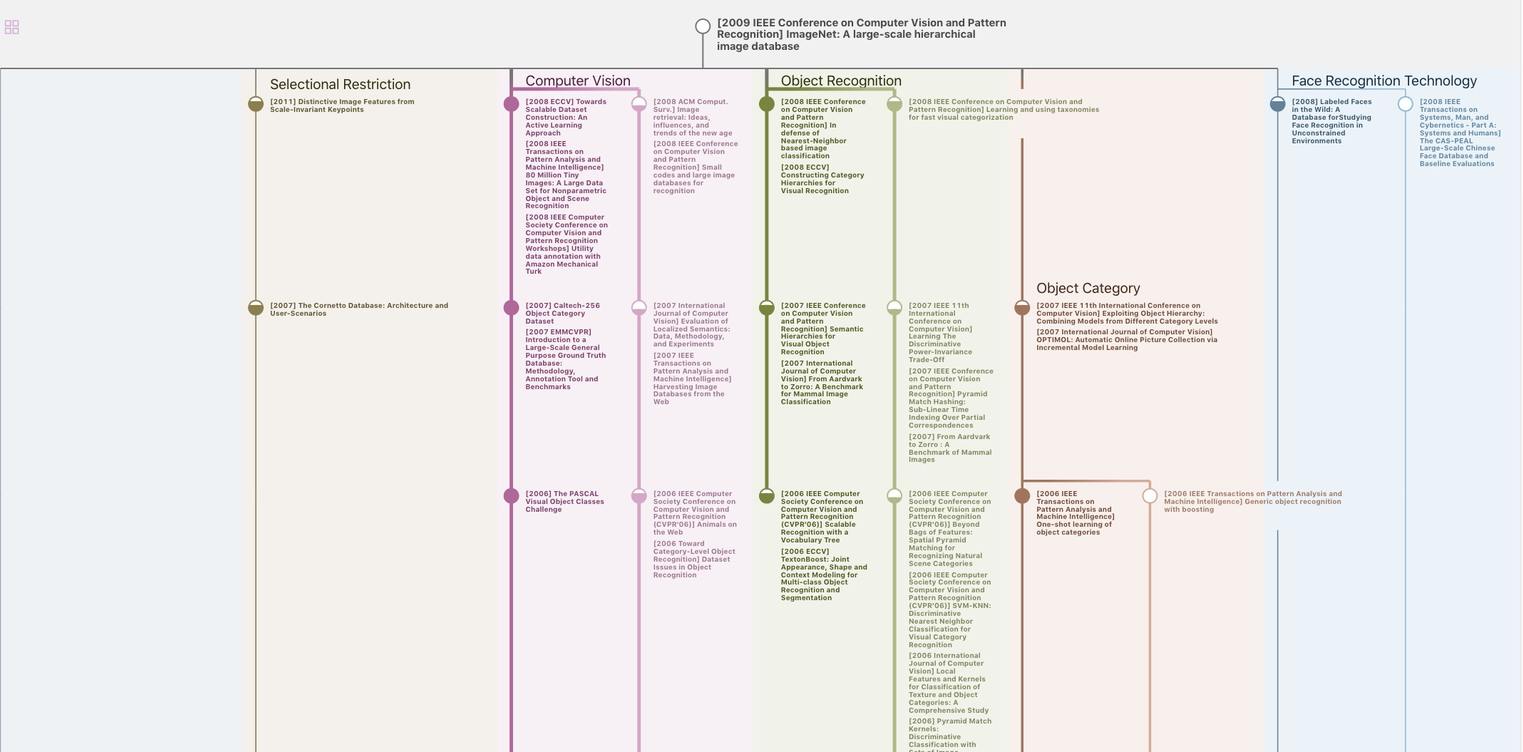
生成溯源树,研究论文发展脉络
Chat Paper
正在生成论文摘要