Automatic and Precise Data Validation for Machine Learning
PROCEEDINGS OF THE 32ND ACM INTERNATIONAL CONFERENCE ON INFORMATION AND KNOWLEDGE MANAGEMENT, CIKM 2023(2023)
摘要
Machine learning (ML) models in production pipelines are frequently retrained on the latest partitions of large, continually growing datasets. Due to engineering bugs, partitions in such datasets almost always have some corrupted features; thus, it's critical to find data issues and block retraining before downstream ML accuracy decreases. However, current ML data validation methods are difficult to operationalize: they yield too many false positive alerts, require manual tuning, or are infeasible at scale. In this paper, we present an automatic, precise, and scalable data validation system for ML pipelines, employing a simple idea that we call a Partition Summarization (PS) approach to data validation: each timestamp-based partition of data is summarized with data quality metrics, and summaries are compared to detect corrupted partitions. We demonstrate how to adapt PS for any data validation method in a robust manner and evaluate several adaptations-which by themselves provide limited precision. Finally, we present gate, our data validation method that leverages these adaptations, giving a 2.1x average improvement in precision over the baseline from prior work on a case study within our large tech company.
更多查看译文
关键词
machine learning,data validation
AI 理解论文
溯源树
样例
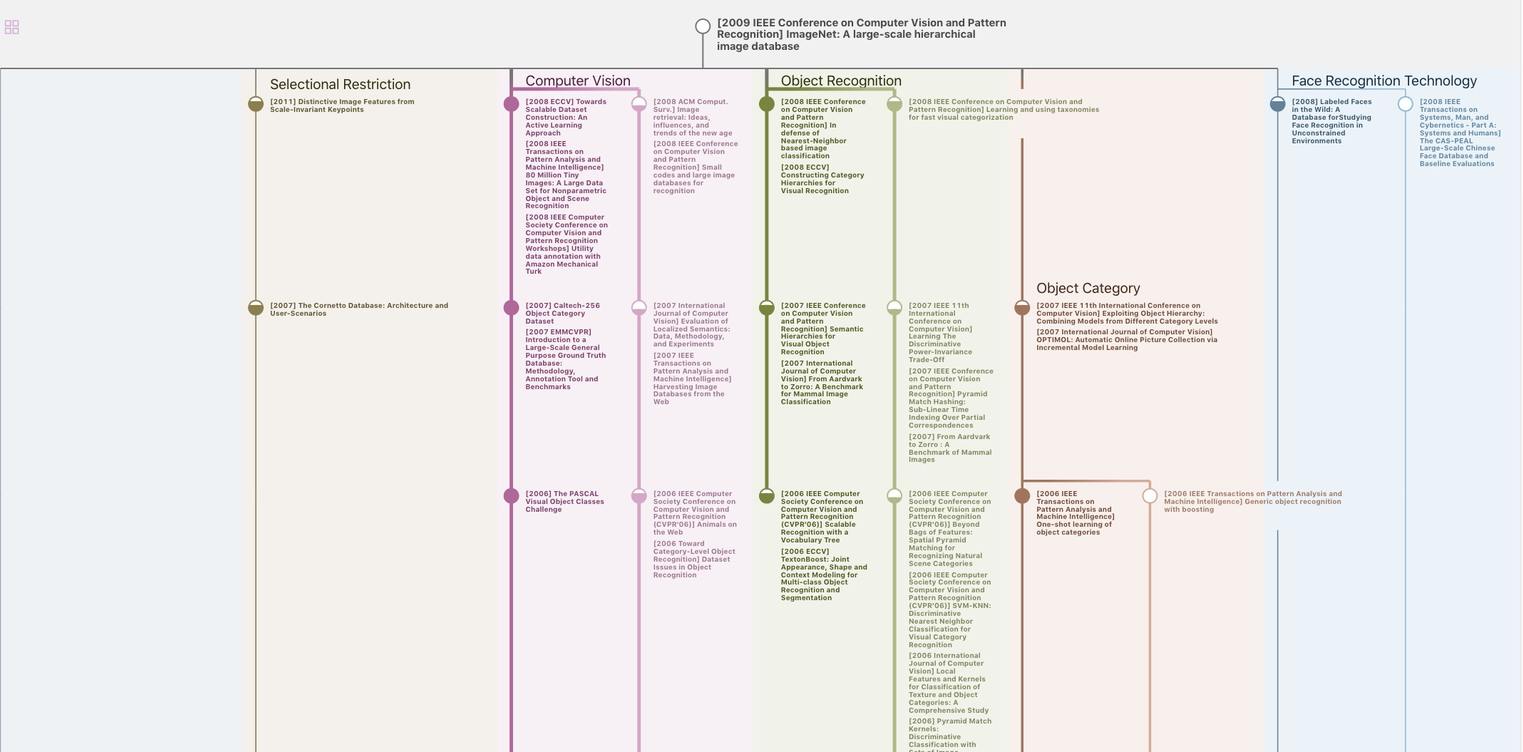
生成溯源树,研究论文发展脉络
Chat Paper
正在生成论文摘要