Topic-Aware Contrastive Learning and K-Nearest Neighbor Mechanism for Stance Detection
PROCEEDINGS OF THE 32ND ACM INTERNATIONAL CONFERENCE ON INFORMATION AND KNOWLEDGE MANAGEMENT, CIKM 2023(2023)
摘要
The goal of stance detection is to automatically recognize the author's expressed attitude in text towards a given target. However, social media users often express themselves briefly and implicitly, which leads to a significant number of comments lacking explicit reference information to the target, posing a challenge for stance detection. To address the missing relationship between text and target, existing studies primarily focus on incorporating external knowledge, which inevitably introduces noise information. In contrast to their work, we are dedicated to mining implicit relational information within data. Typically, users tend to emphasize their attitudes towards a relevant topic or aspect of the target while concealing others when expressing opinions. Motivated by this phenomenon, we suggest that the potential correlation between text and target can be learned from instances with similar topics. Therefore, we design a pretext task to mine the topic associations between samples and model this topic association as a dynamic weight introduced into contrastive learning. In this way, we can selectively cluster samples that have similar topics and consistent stances, while enlarging the gap between samples with different stances in the feature space. Additionally, we propose a nearest-neighbor prediction mechanism for stance classification to better utilize the features we constructed. Our experiments on two datasets demonstrate the advanced and generalization ability of our method, yielding the state-of-the-art results.
更多查看译文
关键词
stance detection,topic association,contrastive learning,pretext task,nearest neighbor mechanism
AI 理解论文
溯源树
样例
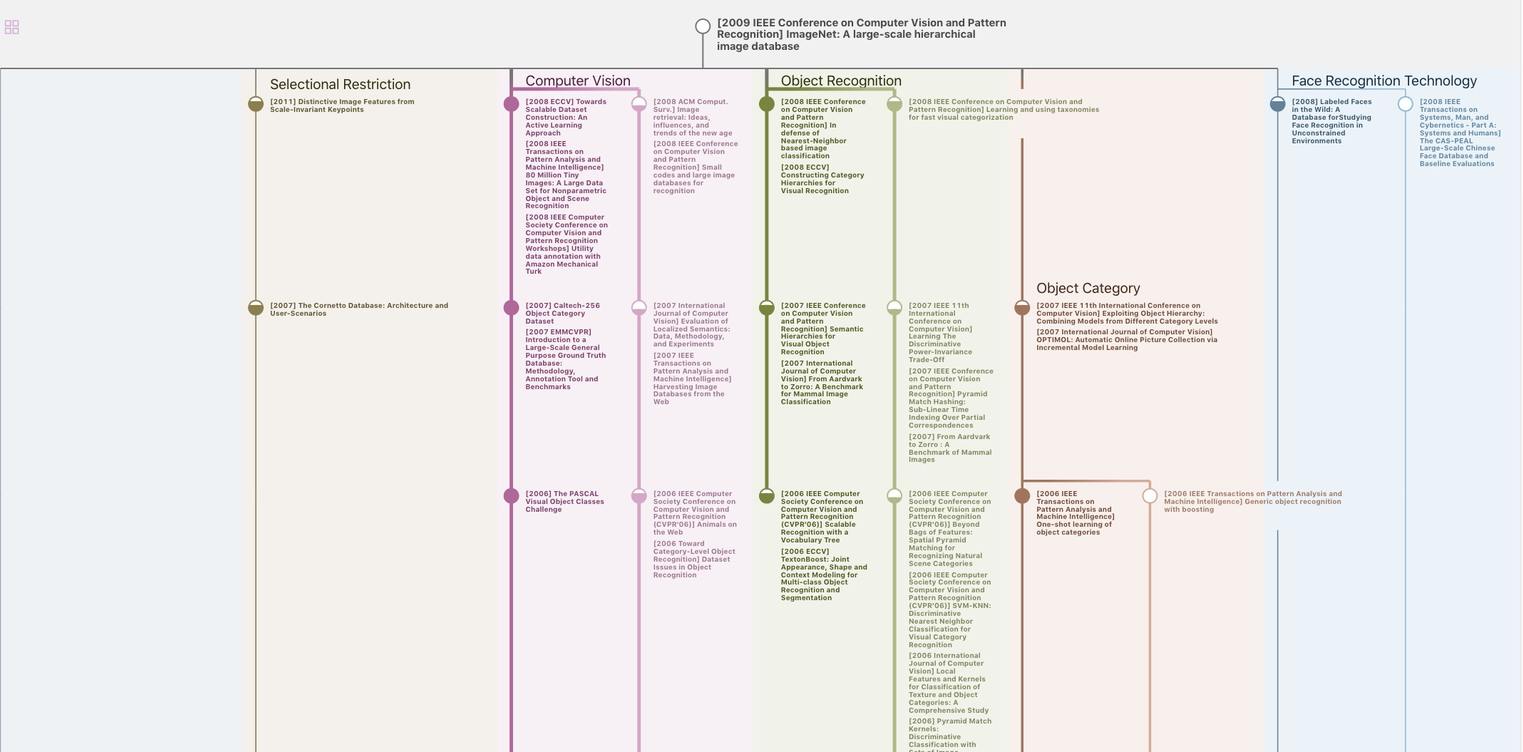
生成溯源树,研究论文发展脉络
Chat Paper
正在生成论文摘要