Periodicity May Be Emanative: Hierarchical Contrastive Learning for Sequential Recommendation
PROCEEDINGS OF THE 32ND ACM INTERNATIONAL CONFERENCE ON INFORMATION AND KNOWLEDGE MANAGEMENT, CIKM 2023(2023)
摘要
Nowadays, contrastive self-supervised learning has been widely incorporated into sequential recommender systems. However, most existing contrastive sequential recommender systems simply emphasize the overall information of interaction sequences, thereby neglecting the special periodic patterns of user behavior. In this study, we propose that users exhibit emanative periodicity towards a group of correlated items, i.e., user behavior follow a certain periodic pattern while their interests may shift from one item to other related items over time. In light of this observation, we present a hierarchical contrastive learning framework to model EmAnative periodicity for SEquential Recommendation (referred to as EASE). Specifically, we design dual-channel contrastive strategy from the perspective of correlation and periodicity to capture emanative periodic patterns. Furthermore, we extend the traditional binary contrastive loss with hierarchical constraint to handle hierarchical contrastive samples, thus preserving the inherent hierarchical information of correlation and periodicity. Comprehensive experiments conducted on five datasets substantiate the effectiveness of our proposed EASE in improving sequential recommendation.
更多查看译文
关键词
Sequential Recommendation,Contrastive Learning,Periodicity
AI 理解论文
溯源树
样例
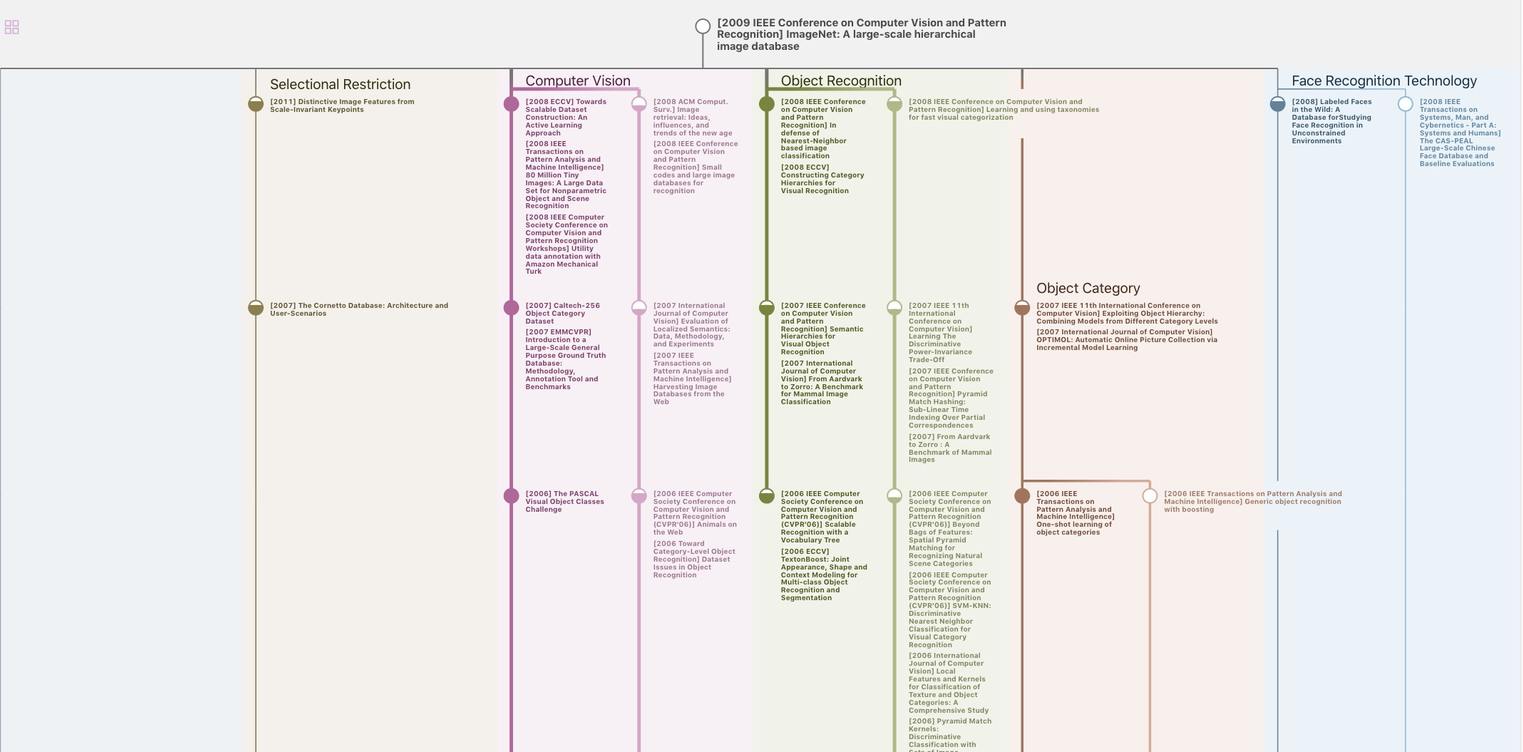
生成溯源树,研究论文发展脉络
Chat Paper
正在生成论文摘要