PSLF: Defending Against Label Leakage in Split Learning
PROCEEDINGS OF THE 32ND ACM INTERNATIONAL CONFERENCE ON INFORMATION AND KNOWLEDGE MANAGEMENT, CIKM 2023(2023)
摘要
With increasing concern over data privacy, split learning has become a widely used distributed machine learning paradigm in practice, where two participants (namely the non-label party and the label party) own raw features and raw labels respectively, and jointly train a model. Although no raw data is communicated between the two parties during model training, several works have demonstrated that data privacy, especially label privacy, is still vulnerable in split learning, and have proposed several defense algorithms against label attacks. However, the theoretical guarantee on the privacy preservation of these algorithms is limited. In this work, we propose a novel Private Split Learning Framework (PSLF). In PSLF, the label party shares only the gradients computed by flipped labels with the non-label party, which improves privacy preservation on raw labels, and meanwhile, we further design an extra sub-model from true labels to improve prediction accuracy. We also design a Flipped Multi-Label Generation mechanism (FMLG) based on randomized response for the label party to generate flipped labels. FMLG is proven differentially private and the label party could make a trade-off between privacy and utility by setting the DP budget. In addition, we design an upsampling method to further protect the labels against some existing attacks. We have evaluated PSLF over real-world datasets to demonstrate its effectiveness in protecting label privacy and achieving promising prediction accuracy.
更多查看译文
关键词
privacy preservation,label leakage,split learning
AI 理解论文
溯源树
样例
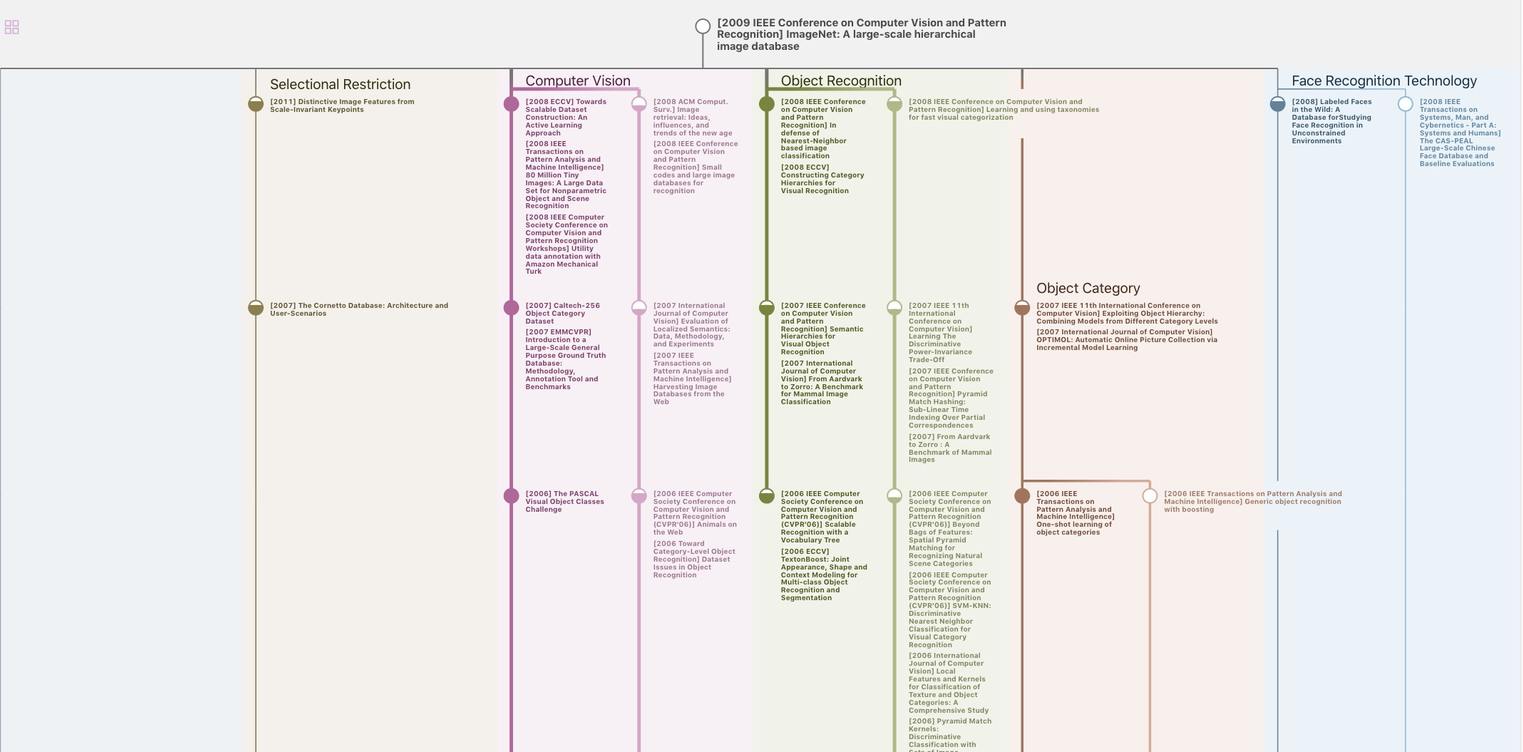
生成溯源树,研究论文发展脉络
Chat Paper
正在生成论文摘要