Closed-form Machine Unlearning for Matrix Factorization
PROCEEDINGS OF THE 32ND ACM INTERNATIONAL CONFERENCE ON INFORMATION AND KNOWLEDGE MANAGEMENT, CIKM 2023(2023)
摘要
Matrix factorization (MF) is a fundamental model in data mining and machine learning, which finds wide applications in diverse application areas, including recommendation systems with user-item rating matrices, phenotype extraction from electronic health records, and spatial-temporal data analysis for check-in records. The "right to be forgotten" has become an indispensable privacy consideration due to the widely enforced data protection regulations, which allow personal users having contributed their data for model training to revoke their data through a data deletion request. Consequently, it gives rise to the emerging task of machine unlearning for the MF model, which removes the influence of the matrix rows/columns from the trained MF factors upon receiving the deletion requests from the data owners of these rows/columns. The central goal is to effectively remove the influence of the rows/columns to be forgotten, while avoiding the computationally prohibitive baseline approach of retraining from scratch. Existing machine unlearning methods are either designed for single-variable models and not compatible with MF that has two factors as coupled model variables, or require alternative updates that are not efficient enough. In this paper, we propose a closed-form machine unlearning method. In particular, we explicitly capture the implicit dependency between the two factors, which yields the total Hessian-based Newton step as the closed-form unlearning update. In addition, we further introduce a series of efficiency-enhancement strategies by exploiting the structural properties of the total Hessian. Extensive experiments on five real-world datasets from three application areas as well as synthetic datasets validate the efficiency, effectiveness, and utility of the proposed method.
更多查看译文
关键词
Machine Unlearning,Matrix Factorization,Privacy-Preserving
AI 理解论文
溯源树
样例
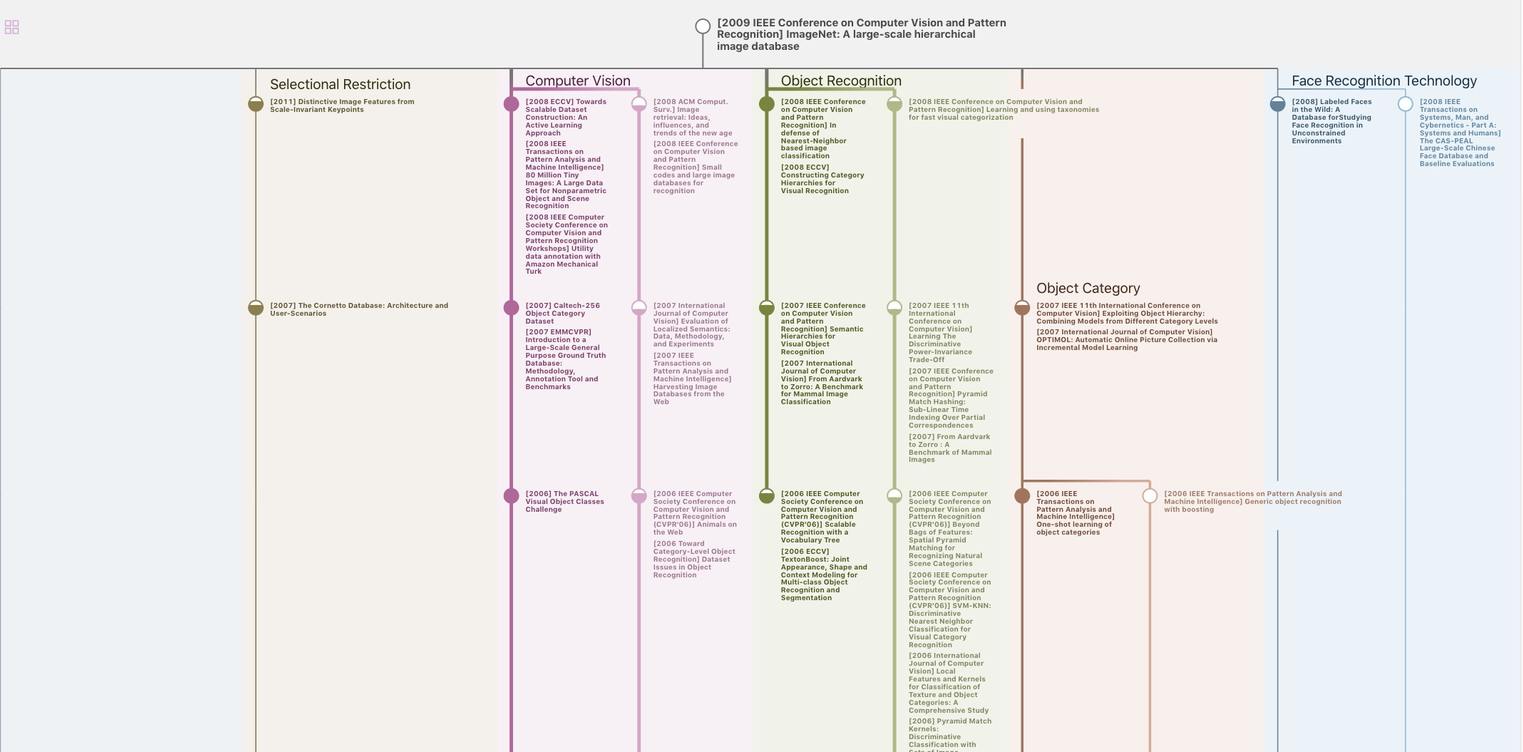
生成溯源树,研究论文发展脉络
Chat Paper
正在生成论文摘要