A Voronoi Diagram and Q-Learning based Relay Node Placement Method Subject to Radio Irregularity
ACM TRANSACTIONS ON SENSOR NETWORKS(2024)
摘要
Industrial Wireless Sensor Networks (IWSNs) have been widely used in industrial applications that require highly reliable and real-time wireless transmission. A lot of works have been done to optimize the Relay Node Placement (RNP), which determines the underlying topology of IWSNs and hence impacts the network performance. However, existing RNP algorithms use a fixed communication radius to compute the deployment result at once offline, while ignoring that the radio environment may vary drastically across different locations, also known as radio irregularity. To address this limitation, we propose a Voronoi diagram and Q-learning based RNP (VQRNP) method in this article. Instead of using a fixed communication radius, VQRNP employs the Q-learning algorithm to dynamically update the radio environment of measured areas, uses a Voronoi diagram basedmethod to estimate the radio environment of unmeasured areas, and proposes a coverage extension location selection algorithm to place RNs so as to extend the coverage of the deployed network based on the results estimated by Voronoi diagram based Graph Generating (VGG). In this way, the VQRPN method can adapt itself well to the variation of radio environment and largely speed up the deployment process. Extensive simulations verify that VQRNP significantly outperforms existing RNP algorithms in terms of reliability.
更多查看译文
关键词
Relay node placement,wireless sensor networks,Q-learning,Voronoi diagram,QoS
AI 理解论文
溯源树
样例
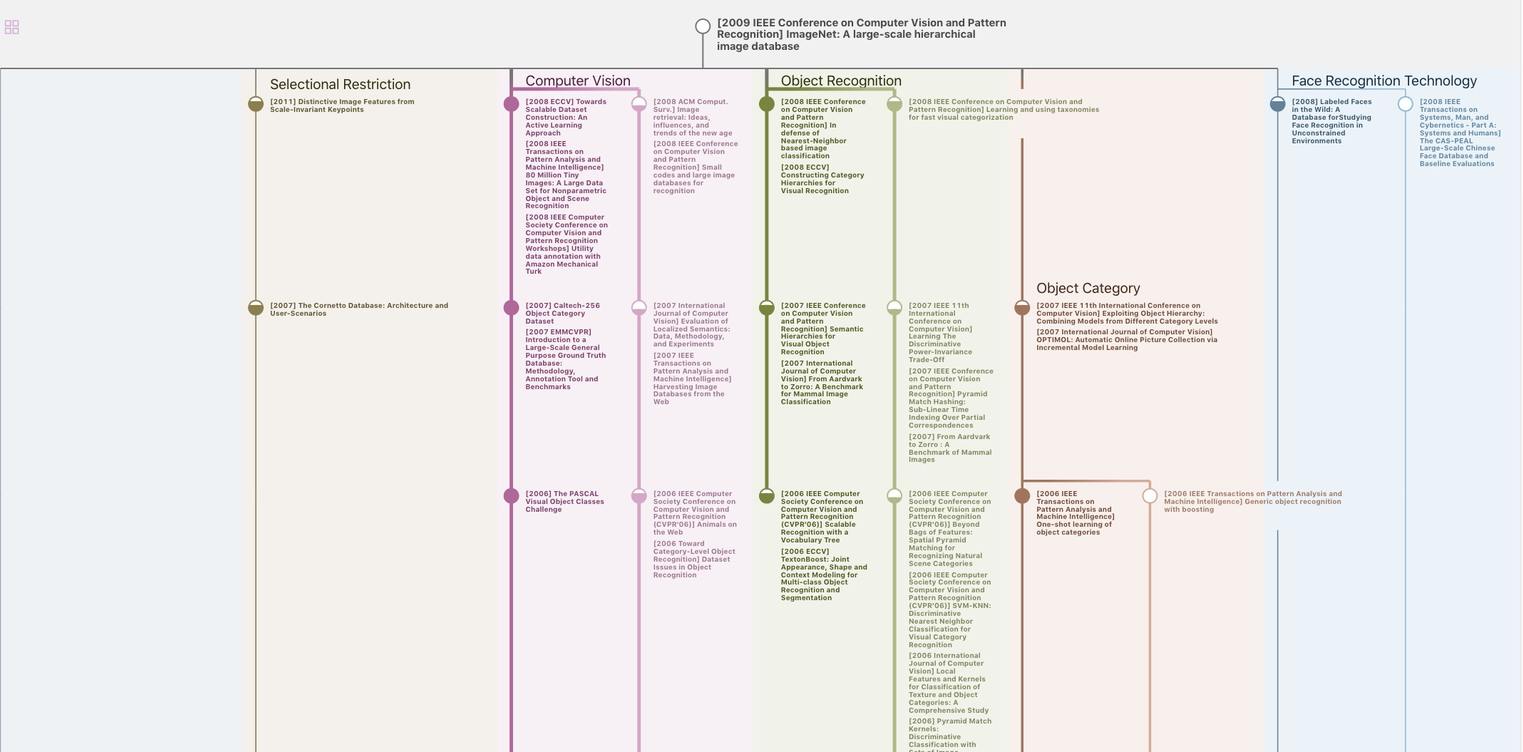
生成溯源树,研究论文发展脉络
Chat Paper
正在生成论文摘要