Analyzing the contribution of different passively collected data to predict Stress and Depression
2023 11TH INTERNATIONAL CONFERENCE ON AFFECTIVE COMPUTING AND INTELLIGENT INTERACTION WORKSHOPS AND DEMOS, ACIIW(2023)
摘要
The possibility of recognizing diverse aspects of human behavior and environmental context from passively captured data motivates its use for mental health assessment. In this paper, we analyze the contribution of different passively collected sensor data types (WiFi, GPS, Social interaction, Phone Log, Physical Activity, Audio, and Academic features) to predict daily self-report stress and PHQ-9 depression score. First, we compute 125 mid-level features from the original raw data. These 125 features include groups of features from the different sensor data types. Then, we evaluate the contribution of each feature type by comparing the performance of Neural Network models trained with all features against Neural Network models trained with specific feature groups. Our results show that WiFi features (which encode mobility patterns) and Phone Log features (which encode information correlated with sleep patterns), provide significative information for stress and depression prediction.
更多查看译文
关键词
depression prediction,stress prediction,Digital Phenotyping,feature importance
AI 理解论文
溯源树
样例
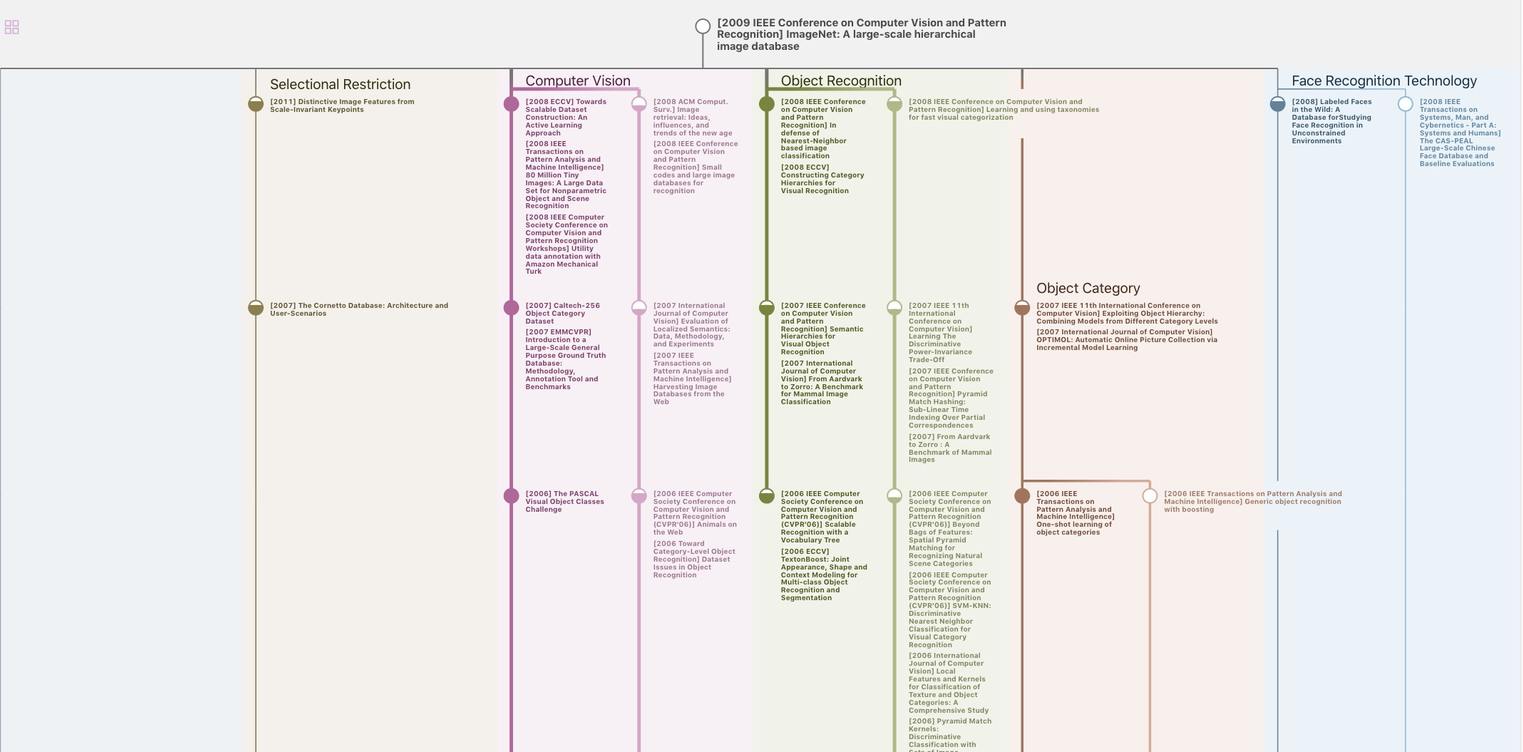
生成溯源树,研究论文发展脉络
Chat Paper
正在生成论文摘要