On Data-Driven Surrogate Modeling for Nonlinear Optimal Control
CoRR(2023)
摘要
In this paper, we study the use of state-of-the-art nonlinear system identification techniques for the optimal control of nonlinear systems. We show that the nonlinear systems identification problem is equivalent to estimating the generalized moments of an underlying sampling distribution and is bound to suffer from ill-conditioning and variance when approximating a system to high order, requiring samples combinatorial-exponential in the order of the approximation, i.e., the global nature of the approximation. We show that the iterative identification of "local" linear time varying (LTV) models around the current estimate of the optimal trajectory, coupled with a suitable optimal control algorithm such as iterative LQR (ILQR), is necessary as well as sufficient, to accurately solve the underlying optimal control problem.
更多查看译文
关键词
surrogate modeling,nonlinear optimal control,optimal control,data-driven
AI 理解论文
溯源树
样例
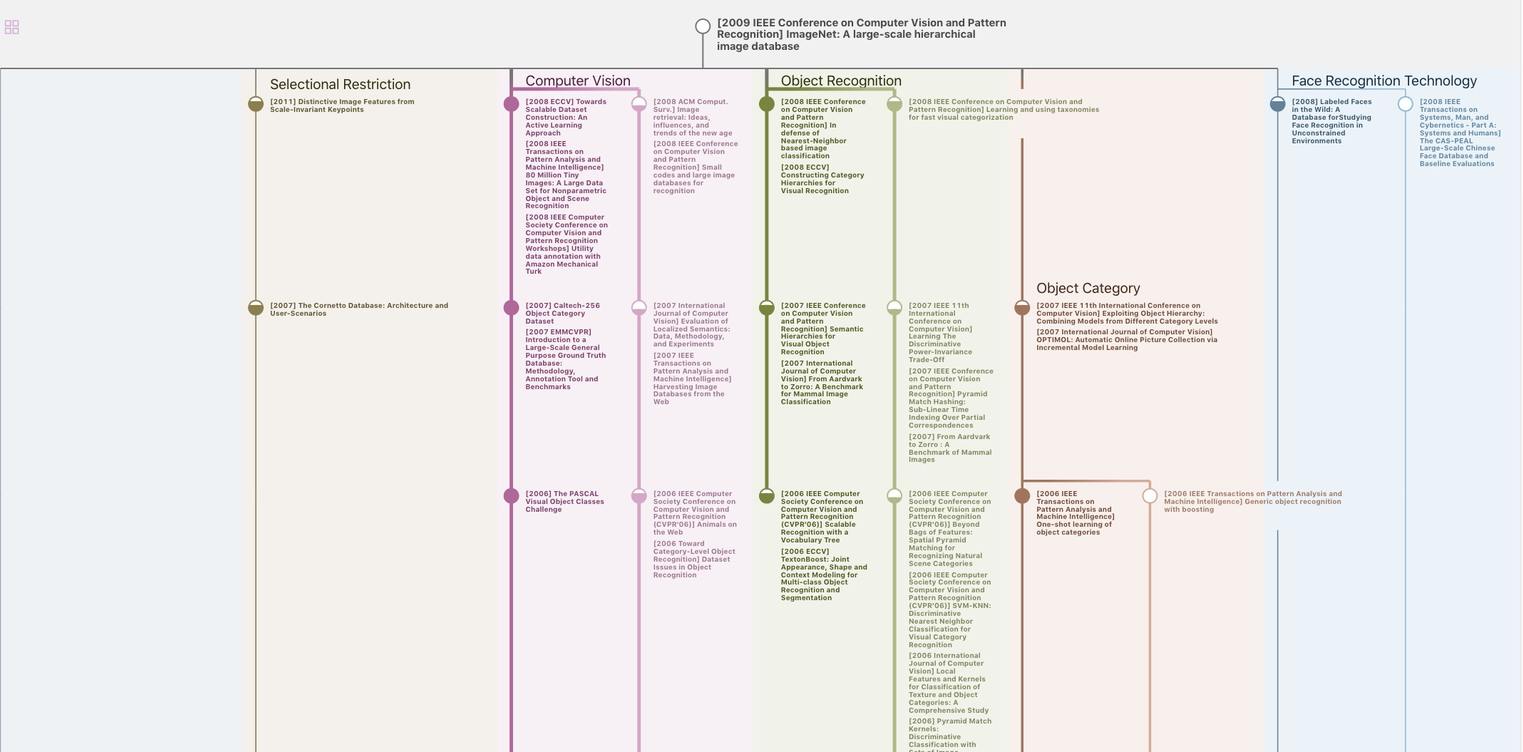
生成溯源树,研究论文发展脉络
Chat Paper
正在生成论文摘要