Auto-Instruct: Automatic Instruction Generation and Ranking for Black-Box Language Models.
CoRR(2023)
摘要
Large language models (LLMs) can perform a wide range of tasks by following natural language instructions, without the necessity of task-specific fine-tuning. Unfortunately, the performance of LLMs is greatly influenced by the quality of these instructions, and manually writing effective instructions for each task is a laborious and subjective process. In this paper, we introduce Auto-Instruct, a novel method to automatically improve the quality of instructions provided to LLMs. Our method leverages the inherent generative ability of LLMs to produce diverse candidate instructions for a given task, and then ranks them using a scoring model trained on a variety of 575 existing NLP tasks. In experiments on 118 out-of-domain tasks, Auto-Instruct surpasses both human-written instructions and existing baselines of LLM-generated instructions. Furthermore, our method exhibits notable generalizability even with other LLMs that are not incorporated into its training process.
更多查看译文
关键词
automatic instruction generation,language models,auto-instruct,black-box
AI 理解论文
溯源树
样例
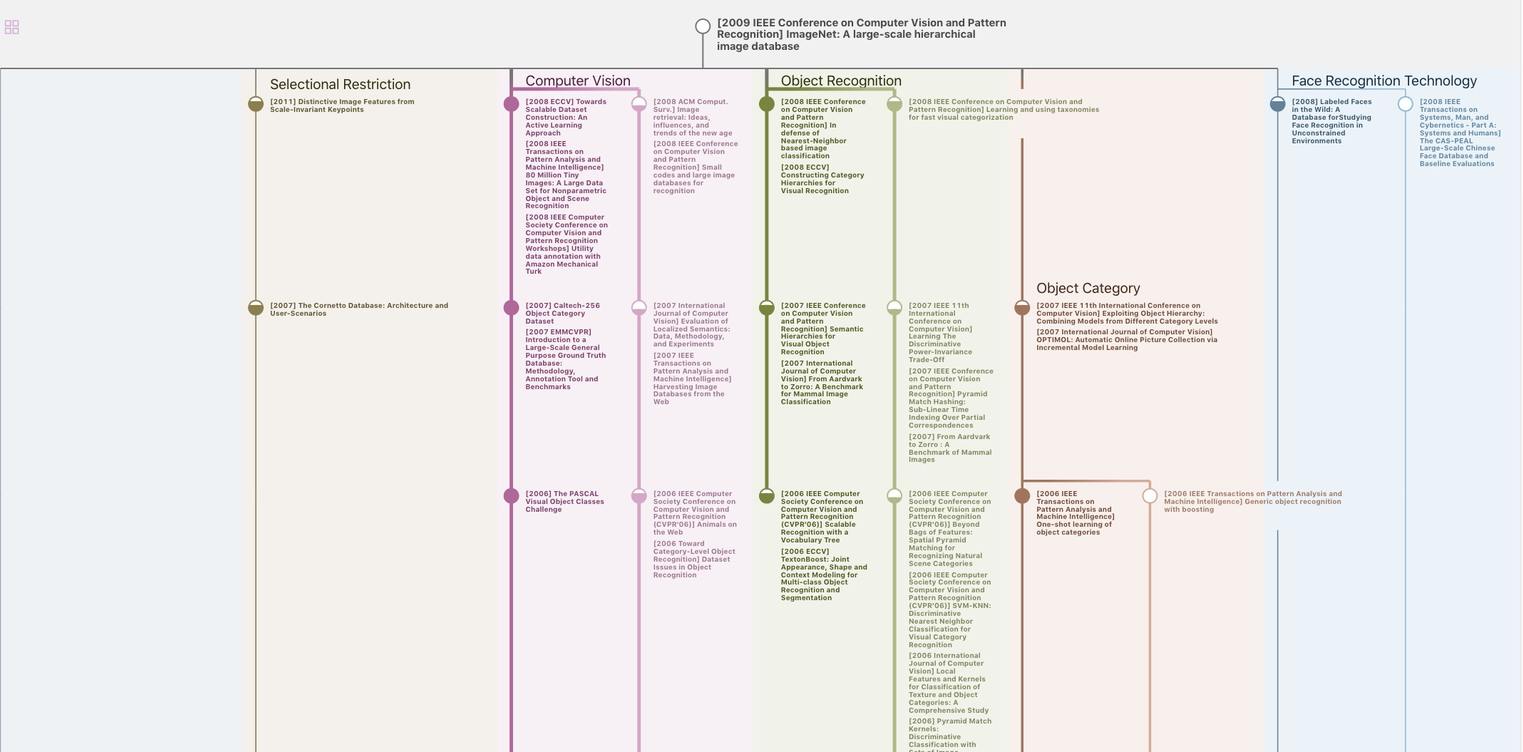
生成溯源树,研究论文发展脉络
Chat Paper
正在生成论文摘要