Electrocardiogram-based deep learning model to screen peripartum cardiomyopathy
ULTRASOUND IN OBSTETRICS & GYNECOLOGY(2023)
摘要
BACKGROUND: Peripartum cardiomyopathy, one of the most fatal conditions during delivery, results in heart failure secondary to left ventricu-lar systolic dysfunction. Left ventricular dysfunction can result in abnormali-ties in electrocardiography. However, the usefulness of electrocardiography in the identification of peripartum cardiomyopathy in pregnant women remains unclear.OBJECTIVE: This study aimed to evaluate the effectiveness of a 12-lead electrocardiography-based artificial intelligence/machine learning-based software as a medical device for screening peripartum cardiomyopathy.STUDY DESIGN: This retrospective cohort study included pregnant women who underwent transthoracic echocardiography between a month before and 5 months after delivery and underwent 12-lead electrocardiog-raphy within 30 days of echocardiography between December 2011 and May 2022 at Seoul National University Hospital. The performance of 12-lead electrocardiography-based artificial intelligence/machine learn-ing analysis (AiTiALVSD software; version 1.00.00, which was developed to screen for left ventricular systolic dysfunction in the general population) was evaluated for the identification of peripartum cardiomyopathy. In addi-tion, the performance of another artificial intelligence/machine learning algorithm using only 1-lead electrocardiography to detect left ventricular systolic dysfunction was evaluated in identifying peripartum cardiomyopa-thy. The results were obtained under a 95% confidence interval and considered significant when P<.05.RESULTS: Among the 14,557 women who delivered during the study period, 204 (1.4%) underwent transthoracic echocardiography a month before and 5 months after delivery. Among them, 12 (5.8%) were diagnosed with peripartum cardiomyopathy. The results showed that AiTiALVSD for 12-lead electrocardiography was highly effective in detect-ing peripartum cardiomyopathy, with an area under the receiver operating characteristic of 0.979 (95% confidence interval, 0.953-1.000), an area under the precision-recall curve of 0.715 (95% confidence interval, 0.499-0.951), a sensitivity of 0.917 (95% confidence interval, 0.760-1.000), a specificity of 0.927 (95% confidence interval, 0.890-0.964), a positive predictive value of 0.440 (95% confidence interval, 0.245-0.635), and a negative predictive value of 0.994 (95% confidence interval, 0.983-1.000). In addition, a 1-lead (lead I) artificial intelligence/machine learning algorithm showed excellent performance; the area under the receiver operating characteristic, area under the preci-sion-recall curve, sensitivity, specificity, positive predictive value, and neg-ative predictive value were 0.944 (95% confidence interval, 0.895 -0.993), 0.520 (95% confidence interval, 0.319-0.801), 0.833 (95% confidence interval, 0.622-1.000), 0.880 (95% confidence interval, 0.834-0.926), 0.303 (95% confidence interval, 0.146-0.460), and 0.988 (95% confidence interval, 0.972-1.000), respectively.CONCLUSION: The 12-lead electrocardiography-based artificial intelligence/machine learning-based software as a medical device (AiTiALVSD) and 1-lead algorithm are noninvasive and effective ways of identifying cardiomyopathies occurring during the peripartum period, and they could potentially be used as highly sensitive screening tools for peripartum cardiomyopathy.
更多查看译文
关键词
artificial intelligence/machine learning model,electrocardi-ography,heart disease,left ventricular systolic dysfunction,peripartum cardiomyopathy
AI 理解论文
溯源树
样例
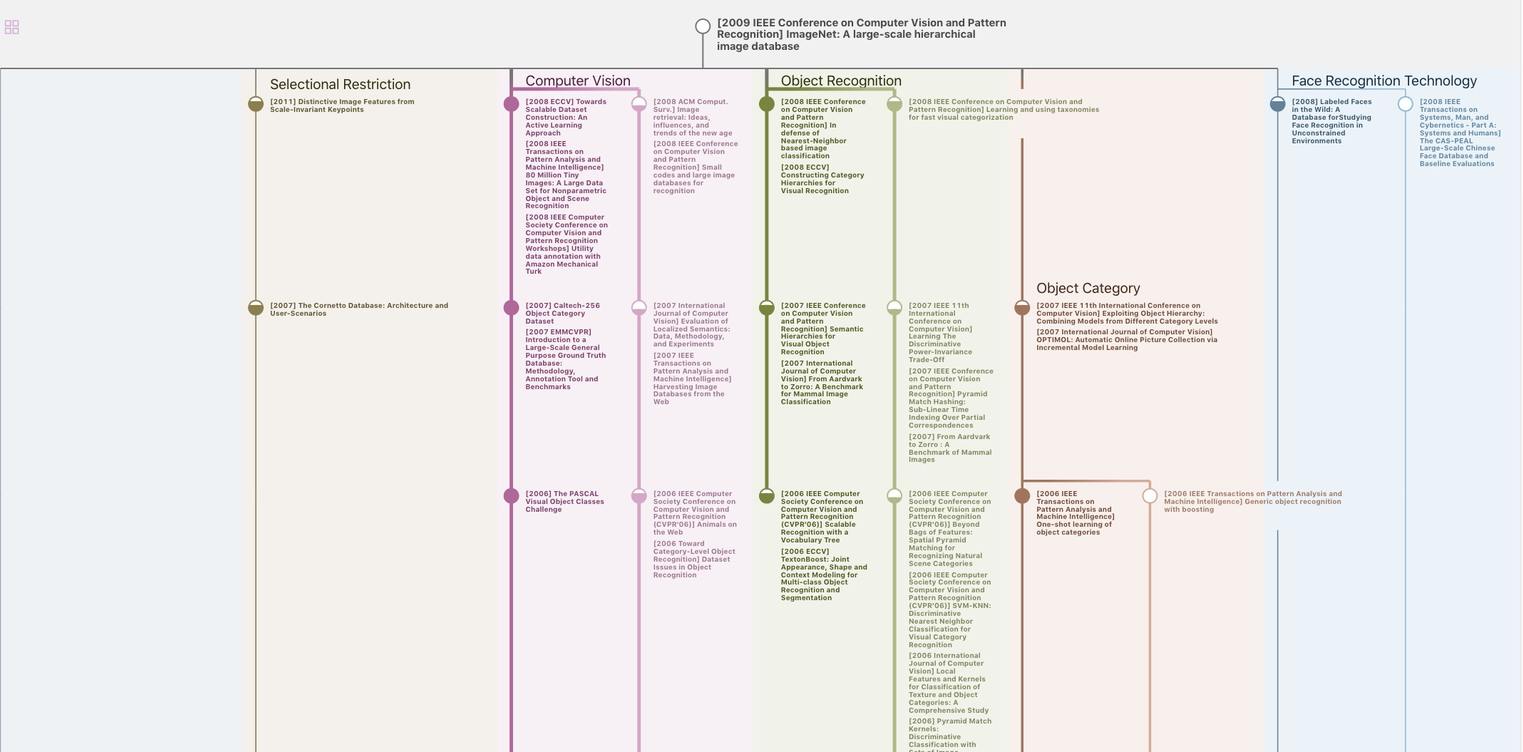
生成溯源树,研究论文发展脉络
Chat Paper
正在生成论文摘要