Slim UNETR: Scale Hybrid Transformers to Efficient 3D Medical Image Segmentation Under Limited Computational Resources
IEEE TRANSACTIONS ON MEDICAL IMAGING(2024)
摘要
Hybrid transformer-based segmentation approaches have shown great promise in medical image analysis. However, they typically require considerable computational power and resources during both training and inference stages, posing a challenge for resource-limited medical applications common in the field. To address this issue, we present an innovative framework called Slim UNETR, designed to achieve a balance between accuracy and efficiency by leveraging the advantages of both convolutional neural networks and transformers. Our method features the Slim UNETR Block as a core component, which effectively enables information exchange through self-attention mechanism decomposition and cost-effective representation aggregation. Additionally, we utilize the throughput metric as an efficiency indicator to provide feedback on model resource consumption. Our experiments demonstrate that Slim UNETR outperforms state-of-the-art models in terms of accuracy, model size, and efficiency when deployed on resource-constrained devices. Remarkably, Slim UNETR achieves 92.44% dice accuracy on BraTS2021 while being 34.6x smaller and 13.4x faster during inference compared to Swin UNETR.
更多查看译文
关键词
Biomedical imaging,Transformers,Image segmentation,Task analysis,Computational modeling,Three-dimensional displays,Solid modeling,3D medical segmentation,lightweight,medical image analysis,resource-limited application
AI 理解论文
溯源树
样例
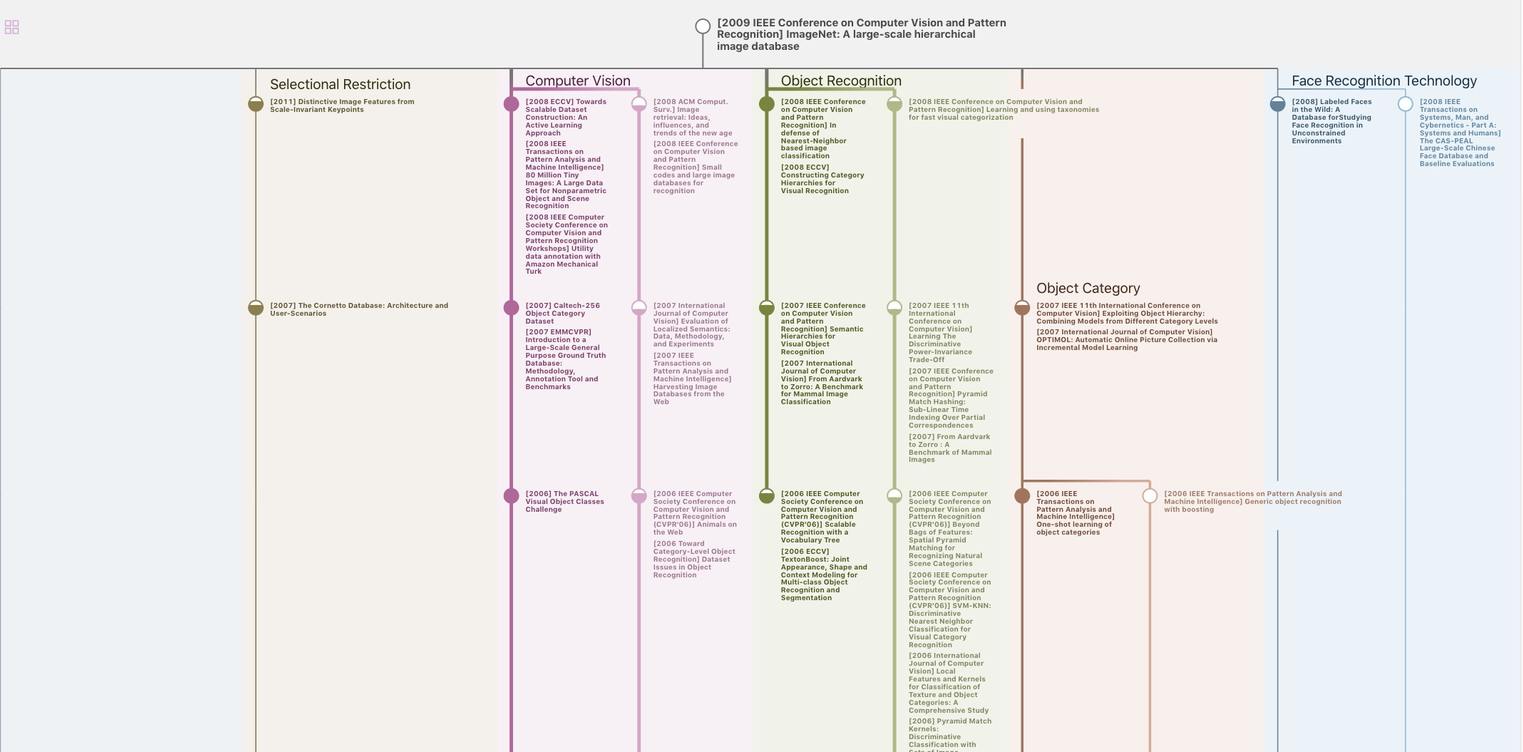
生成溯源树,研究论文发展脉络
Chat Paper
正在生成论文摘要