An Easy Data Augmentation Approach for Application Reviews Event Inference
IEEE TRANSACTIONS ON SOFTWARE ENGINEERING(2023)
摘要
Application review event inference aims to assess the effectiveness of application problems in response to user actions, which enables application developers to promptly discover and address potential issues in various applications, thereby improving their development and maintenance efficiency. Despite the development of event inference models for app reviews, which extract them as user action and app problem events and establish a relationship model between events and inference labels, the accuracy of these models is constrained due to limitations in labeling and characterizing noise and the lack of robustness and generalization. To address this challenge, we propose a model called Easy Data Augmentation for Application Reviews Event Inference (short for EDA-AREI), which comprises a denoising component, data augmentation component, and event inference prediction component. Specifically, the denoising component identifies labels and characterizes noisy data to enhance dataset quality, the data augmentation component replaces non-stop words with synonyms to increase textual diversity, and the event inference and prediction component reconstructs the classifier using denoised and augmented data. Experimental results on six datasets of one-star app reviews in the Apple App Store demonstrate that the EDA-AREI method achieves an Accuracy of 71.19%, 79.14%, 69.05%, 69.02%, 68.24% and 68.48%, respectively, representing an improvement of 0.83%-2.09% compared to state-of-the-art models. Regarding the F1-score, EDA-AREI achieves values of 71.30%, 69.93%, and 68.76% on the threshold_0.5, k-means_2, and random datasets, respectively, outperforming state-of-the-art models by 1.89%-4.02%. Furthermore, EDA-AREI achieves AUC values of 75.66% and 73.37% on the threshold_0.5 and k-means_2 datasets, respectively. As a result, EDA-AREI demonstrates substantial improvements in Accuracy, as well as enhanced F1-score and AUC across most datasets, thereby enhancing the model's accuracy and robustness in identifying related action-problem pairs.
更多查看译文
关键词
Application reviews event inference,data augmentation,Confident Learning
AI 理解论文
溯源树
样例
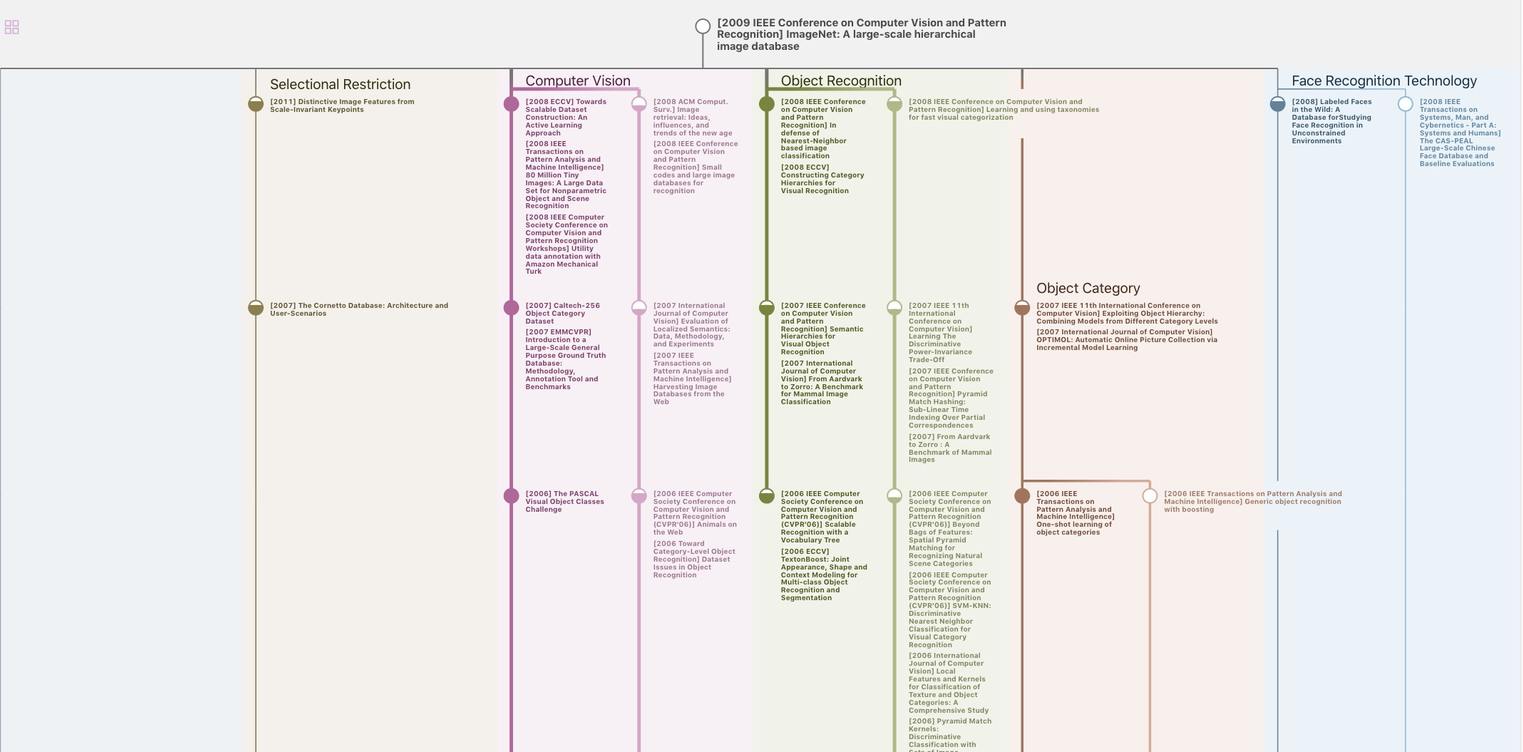
生成溯源树,研究论文发展脉络
Chat Paper
正在生成论文摘要