Graph Deep-Learning-Based Retail Dynamic Pricing for Demand Response
IEEE TRANSACTIONS ON SMART GRID(2023)
摘要
Designing customized dynamic pricing is a promising way to incent consumers to adjust their daily energy consumption behaviors. It helps manage flexible demand response resources on peak load. However, it is insufficiently investigated in previous studies from the individual behavior perspective. To tackle the gap, this paper proposes a graph deep learning-based retail dynamic pricing mechanism. First, a graph attention network-based temporal price elasticity perceptron model is proposed. It explores a novel path to learn price elasticity by using graph deep learning, and can accurately assess consumers' energy consumption behaviors under different prices. Then, to avoid unfair evaluation of demand response, two indexes are proposed as auxiliary measures to assess energy consumption behavior learning models. At last, a customized dynamic pricing model based on the temporal price elasticity perceptron model is proposed. It can develop consumer's time-varying demand response potential. This potential is first defined in this paper to measure what potentials of shifting/curtailing energy during a period a consumer has. By the pricing, the consumer could be incented to engage in demand response. The numerical studies validate the feasibility and superiority of the proposed methods, meanwhile price risks from the price change can be hedged effectively.
更多查看译文
关键词
Customized dynamic pricing,demand response,graph deep learning,temporal price elasticity perceptron
AI 理解论文
溯源树
样例
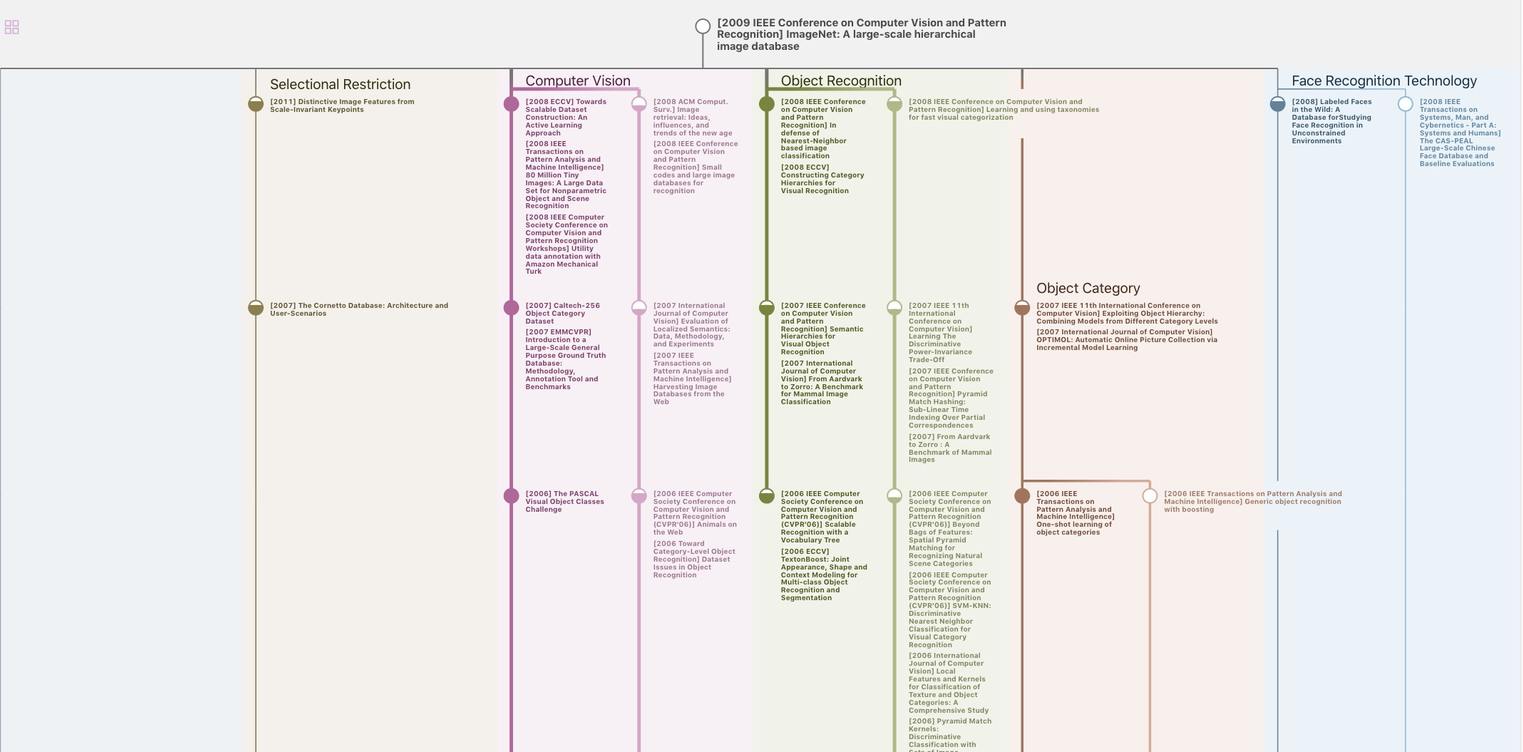
生成溯源树,研究论文发展脉络
Chat Paper
正在生成论文摘要