Learning-based spacecraft reactive anti-hostile-rendezvous maneuver control in complex space environments
Advances in Space Research(2023)
摘要
To realize rapid, safe and optimal avoidance for spacecraft in complex threat situations and incomplete-information conditions, and considering the problems in existing hierarchical decision-making modes, a learning-based spacecraft reactive anti-hostile-rendezvous (AHR) maneuver control framework is proposed in this paper. First, a fundamental AHR action planner based on the interfered fluid dynamical system (IFDS) is proposed, in which the avoidance strategies for hostile and non-adversarial threats are appropriately integrated. An avoidance strategy matched with the IFDS is further designed by specifically considering high-intensity confrontation situations where a threat maintains persistent pursuit. Then, the “state-action” solution methods based on deep reinforcement learning (DRL) and multi-agent-DRL (MADRL) methods for orbital avoidance maneuvers are proposed to control the avoidance time and directions by optimizing the IFDS parameters. In the offline training stage, the DRL method will first simulate the interactive processes between the spacecraft and non-adversarial threats so that the spacecraft can gradually adapt to complex space environments and generate the relative primary avoidance maneuver strategies. On this basis, for hostile threats, the MADRL-based solution method of orbit game strategies is further established, in which the spacecraft and hostile threats are constructed as two types of opposite agents to simulate the game processes so that the strategy levels can be bilaterally evolved and the AHR success rates can be improved. After training, the corresponding neural networks are extracted from the agents in the DRL-based and MADRL-based methods for reactive AHR maneuver control. Moreover, a progressive agent training mechanism matched with the above DRL-based and MADRL-based solution methods is proposed, which can reduce the invalid interactions in initial training episodes, improve the training efficiency, and enhance the generalization ability of orbital game strategies. Finally, the effectiveness of the proposed framework is demonstrated by simulations.
更多查看译文
关键词
control,learning-based,anti-hostile-rendezvous
AI 理解论文
溯源树
样例
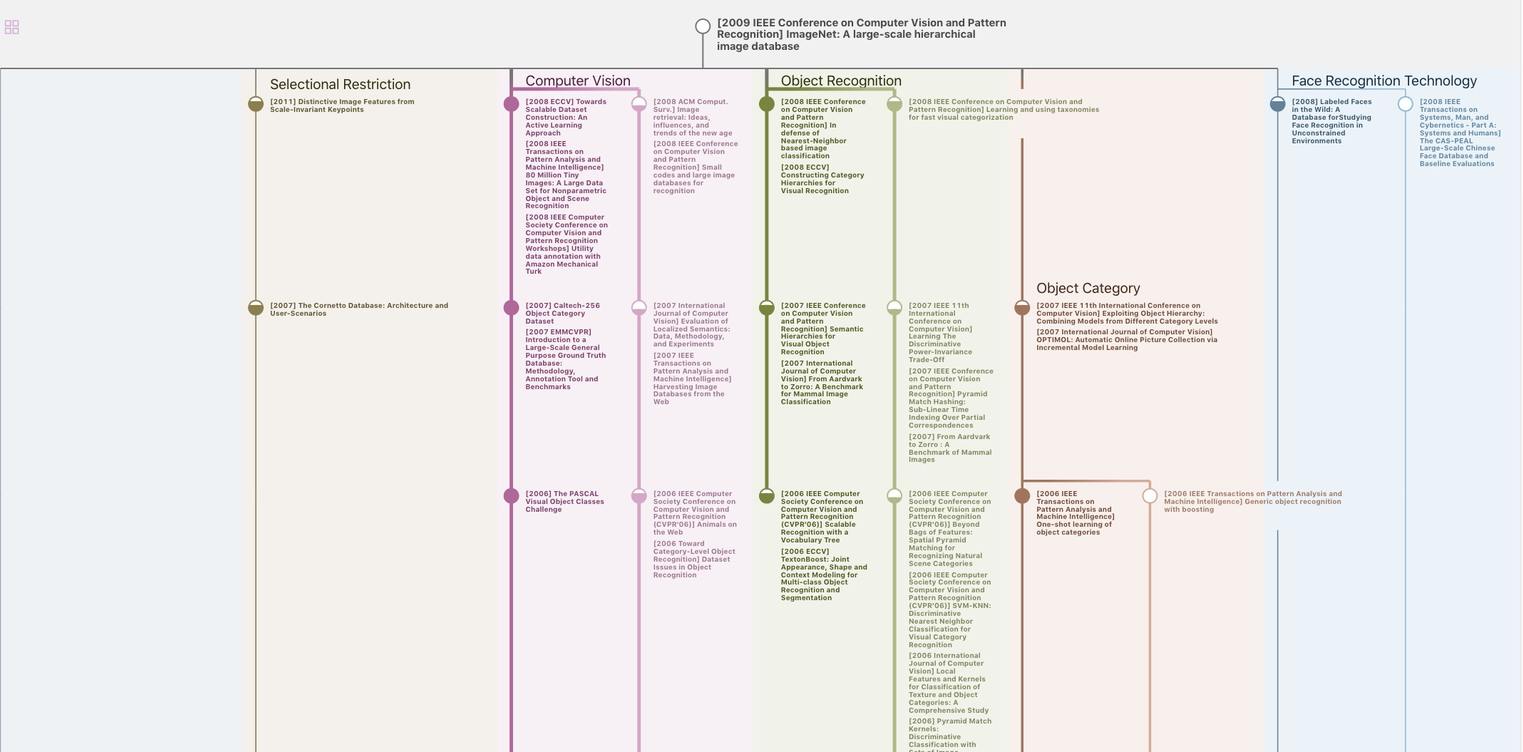
生成溯源树,研究论文发展脉络
Chat Paper
正在生成论文摘要