Boundary dependent physics-informed neural network for solving neutron transport equation
ANNALS OF NUCLEAR ENERGY(2024)
摘要
The boundary dependent physics-informed neural network (BDPINN) is proposed for solving the neutron transport equation (NTE). Developed based on physics-informed neural network (PINN), BDPINN eliminates errors caused by boundary conditions, effectively avoids the curse of dimensionality and reduces ray effects caused by solid angle discretization. Original constructions of trial functions are proposed for common boundary conditions in NTE to improve calculation accuracy. Three techniques are introduced to improve BDPINN: thirdorder tensor transformation, rearranging the training set, and result reconstruction in high order. These techniques reduce computational cost and improve accuracy. In addition, two existing techniques, area decomposition and multi-group iteration are applied to solve multi-group and complex area problems. The accuracy of BDPINN is verified through benchmark comparisons with a nodal method and the mechanism for reducing the ray effect is analyzed. This work provides novel perspectives and techniques for solving NTE.
更多查看译文
关键词
Neutron transport equation,Physics -informed neural network,Boundary dependent,Deep learning,Ray effect
AI 理解论文
溯源树
样例
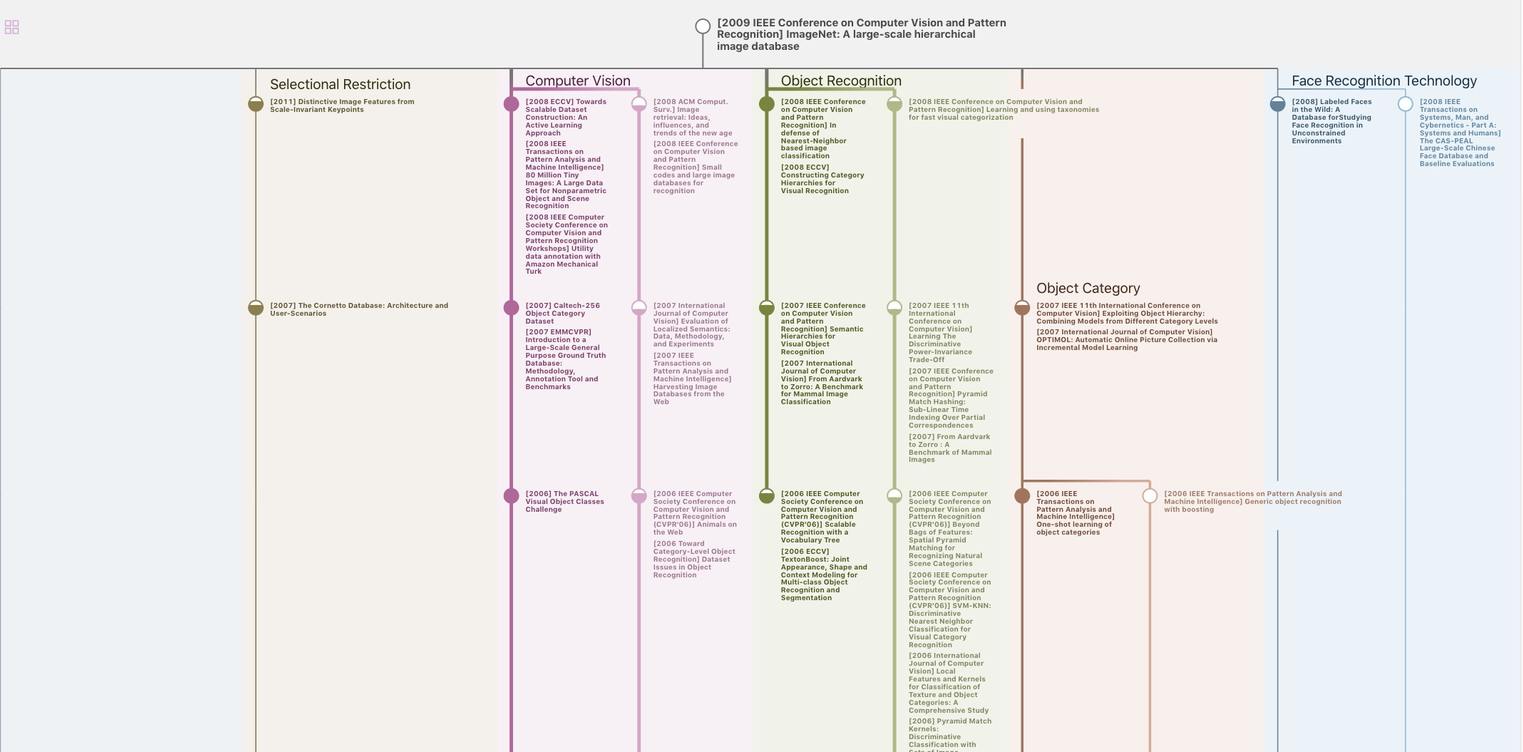
生成溯源树,研究论文发展脉络
Chat Paper
正在生成论文摘要