Online EV charging controlled by reinforcement learning with experience replay
SUSTAINABLE ENERGY GRIDS & NETWORKS(2023)
摘要
The extensive penetration of distributed energy resources (DERs), particularly electric vehicles (EVs), creates a huge challenge for the distribution grids due to the limited capacity. An approach for smart charging might alleviate this issue, but most of the optimization algorithms has been developed so far under an assumption of knowing the future, or combining it with complicated forecasting models. In this paper we propose to use reinforcement learning (RL) with replaying past experience to optimally operate an EV charger. We also introduce explorative rewards for better adjusting to environment changes. The reinforcement learning agent controls the charger's power of consumption to optimize expenses and prevent lines and transformers from being overloaded. The simulations were carried out in the IEEE 13 bus test feeder with the load profile data coming from the residential area. To simulate the real availability of data, an agent is trained with only the transformer current and the local charger's state, like state of the charge (SOC) and timestamp. Several algorithms, namely Q-learning, SARSA, Dyna-Q and Dyna-Q+ are tested to select the best one to utilize in the stochastic environment and low frequency of data streaming. (c) 2023 The Author(s). Published by Elsevier Ltd. This is an open access article under the CC BY license (http://creativecommons.org/licenses/by/4.0/).
更多查看译文
关键词
Congestion management,Distribution network,Electric vehicles,Reinforcement learning
AI 理解论文
溯源树
样例
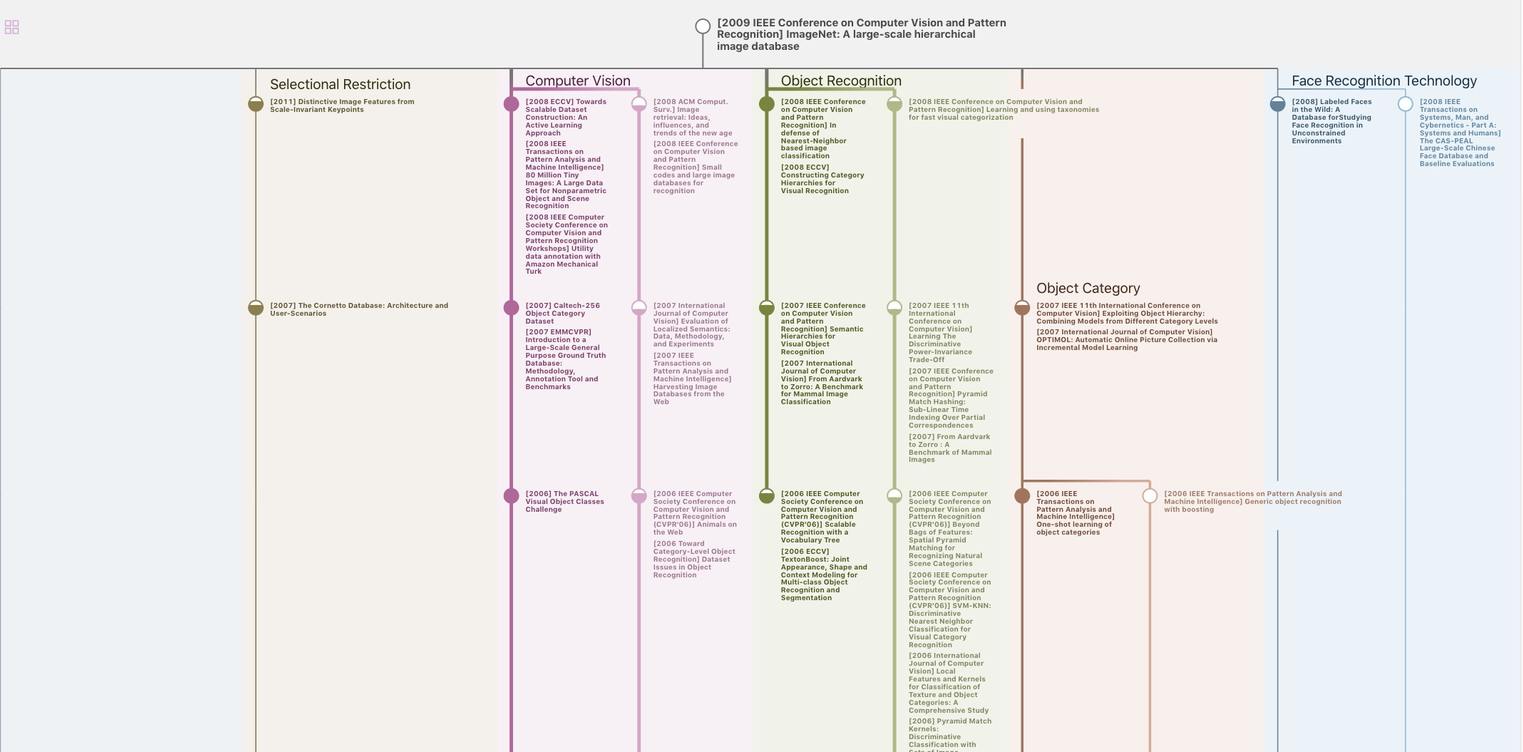
生成溯源树,研究论文发展脉络
Chat Paper
正在生成论文摘要