Wind turbine contaminant classification using machine learning techniques
SPECTROCHIMICA ACTA PART B-ATOMIC SPECTROSCOPY(2023)
摘要
It has been well established in the literature that surface contamination can adversely affect the aerodynamic performance of aerofoils and hence the efficiency with which turbines can convert wind to electrical power. Hence it is critical to ensure that turbine blades are kept as free as possible of contaminants. In this manuscript, we discuss LIBS and machine learning techniques on contaminated wind turbine blades with a view to the possibility of integrating these methods into a standoff laser ablation setup in the future. A LIBS signal will be a key step in the decision process, i.e., to determine if a wind turbine blade has been fouled in the first place. Relatedly one must also determine the point at which the laser has adequately removed contaminants from the current Area of Irradiation (AoI) before moving to the adjacent AoI, i.e., interim end-point-detection. For both steps (presence / absence of fouling) we are investigating laser-induced breakdown spectroscopy (LIBS) to discriminate clean from fouled wind turbine blade samples. In particular we have performed LIBS in both the Vacuum Ultraviolet (VUV) and Ultraviolet Visible (UV-Vis) spectral ranges. Analysis of the spectra showed only slight variations in the constituent materials between clean and contaminated blade samples. In order to address this challenge, the efficacy of a number of machine learning and statistical methods for clean versus contaminated blade classification was investigated. Four methods (Partial Least Squares Discriminant Analysis (PLS-DA), Support Vector Machines (SVM), Competitive Learning (CL), and Convolutional Neural Networks (CNN)) were evaluated. The spectral regions where machine learning algorithms were applied was determined via a volumetric ellipsoid overlap test based on Principal Component Analysis (PCA). It was found that SVMs provided the most accurate methodology for binary classification of clean vs contaminated blades whilst also yielding the shortest run time.
更多查看译文
关键词
LIBS,Wind-turbines,Machine-learning,Spectroscopy
AI 理解论文
溯源树
样例
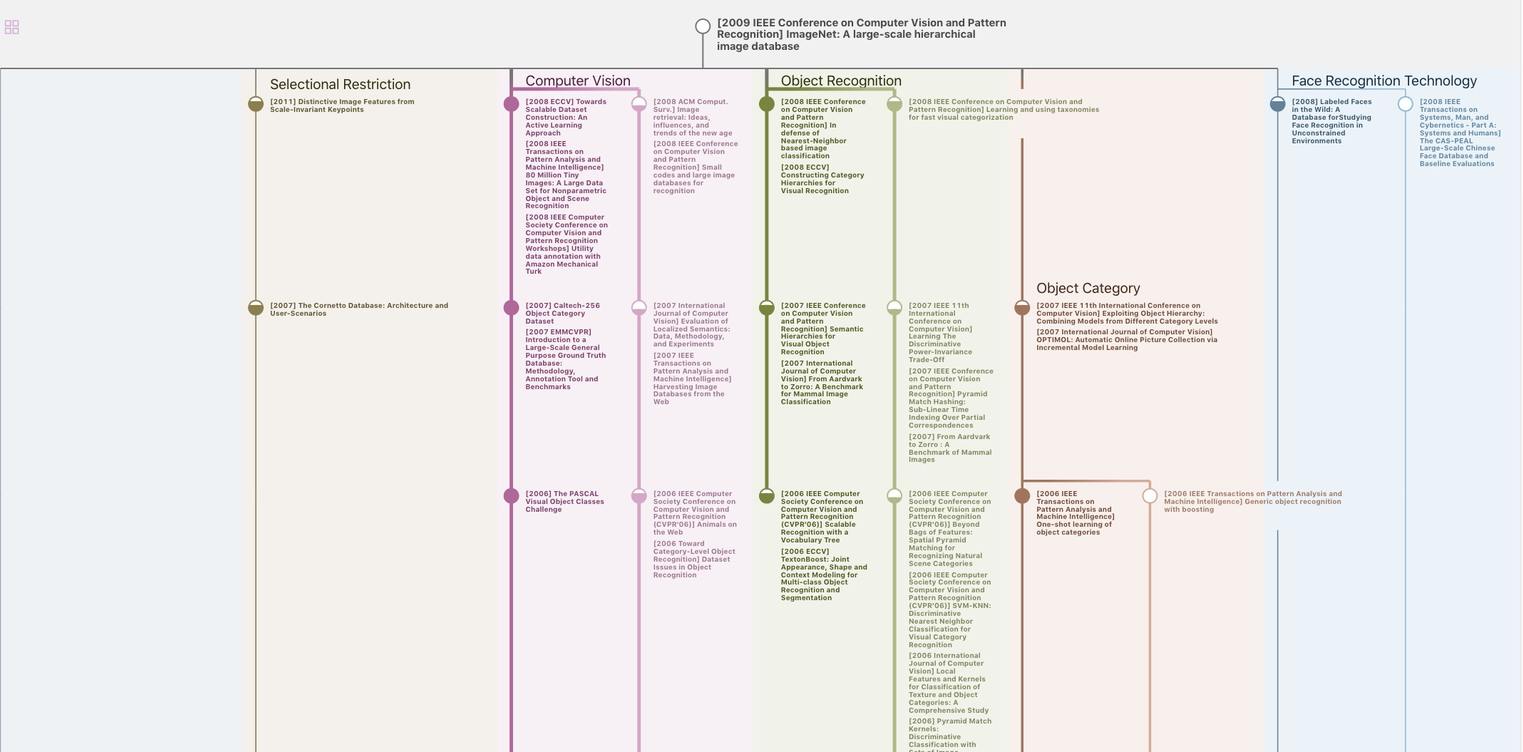
生成溯源树,研究论文发展脉络
Chat Paper
正在生成论文摘要