Pyramidal 3D feature fusion on polar grids for fast and robust traversability analysis on CPU
Robotics and Autonomous Systems(2023)
摘要
Self-driving vehicles and autonomous ground robots require a reliable and accurate method to analyze the traversability of the surrounding environment for safe navigation. This paper proposes and evaluates a real-time machine learning-based traversability analysis method that combines geometric features with a pyramid-polar space representation based on SVM classifiers. In particular, we show that by fusing geometric features with information stemming from coarser pyramid levels that account for a broader space portion, as well as integrating important implementation details, allows for a noticeable boost in performance and reliability. The main goal of this work is to demonstrate that traversability analysis is possible with effective results and in real-time even on cheaper hardware than expensive GPUs, e.g. CPU-only PCs. The proposed approach has been compared with state-of-the-art deep learning approaches on publicly available datasets of outdoor driving scenarios, running such algorithms both on GPU and CPU to compare runtimes. Our method can be fully executed on CPU and achieves results close to the best-in-class methods, runs faster, and requires fewer and less expensive hardware resources, consuming less than 30% electrical power with respect to deep learning models on embedded processing units. We release with this paper the open-source implementation of our method.
更多查看译文
关键词
Traversability analysis,3D LiDAR semantic segmentation,Autonomous driving,Machine learning
AI 理解论文
溯源树
样例
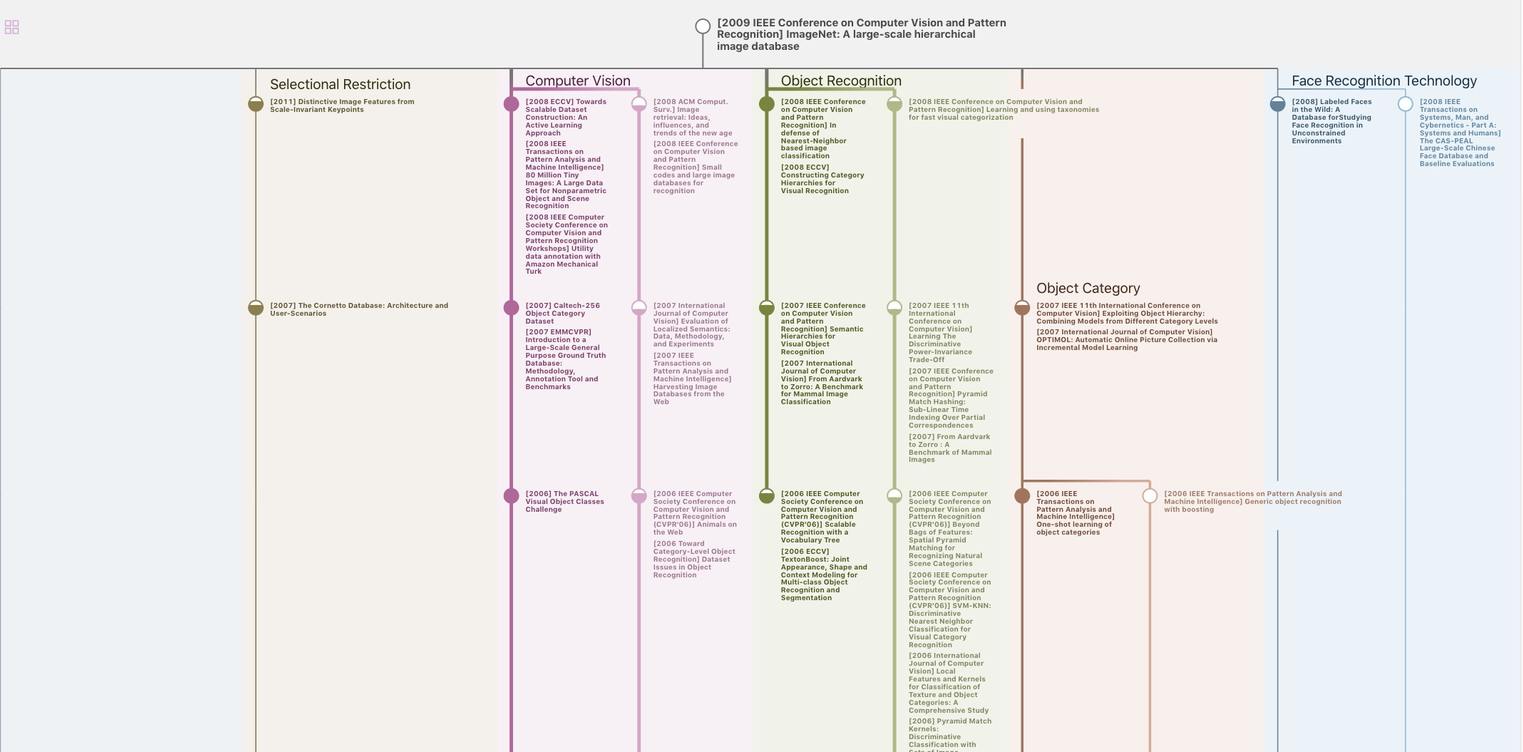
生成溯源树,研究论文发展脉络
Chat Paper
正在生成论文摘要