Uncertainty-aware trustworthy weather-driven failure risk predictor for overhead contact lines
RELIABILITY ENGINEERING & SYSTEM SAFETY(2024)
摘要
Overhead contact lines (OCLs) are electric transmission lines that power railways, which are constantly threatened by external weather and environmental factors due to their outdoor location. Hence, for the long-term functioning of railway lines, a weather-driven risk predictor is an essential tool. Current prediction methods mainly adopt a single-point estimation system with fixed weights of neural networks and therefore cannot propagate the uncertainties within the data and model, resulting in unreliable predictions. To enhance safety-risk prevention capabilities, this paper proposes an uncertainty-aware trustworthy weather-driven failure-risk approach for OCLs, in a probabilistic deep multitask learning framework. Firstly, a deep Gaussian process is employed as the backbone model to deal with imbalanced weather-related failure datasets with limited fault samples. Moreover, a multi-task learning framework is embedded to simultaneously predict the multiple weather-driven failure risks under lightning, wind and haze. Finally, the predictive uncertainty is decomposed into epistemic and aleatory uncertainties, where epistemic and aleatory uncertainties account for the uncertainty within the model and data, respectively. Extensive experiments on actual OCLs are carried out to demonstrate the effectiveness of the proposed approach, which can effectively capture the predictive uncertainty and provide trustworthy predictive decisions of mitigating operational risk for railway operators.
更多查看译文
关键词
Overhead contact lines,Weather,Multi-task learning,Imbalanced dataset,Probabilistic deep learning,Uncertainty evaluation
AI 理解论文
溯源树
样例
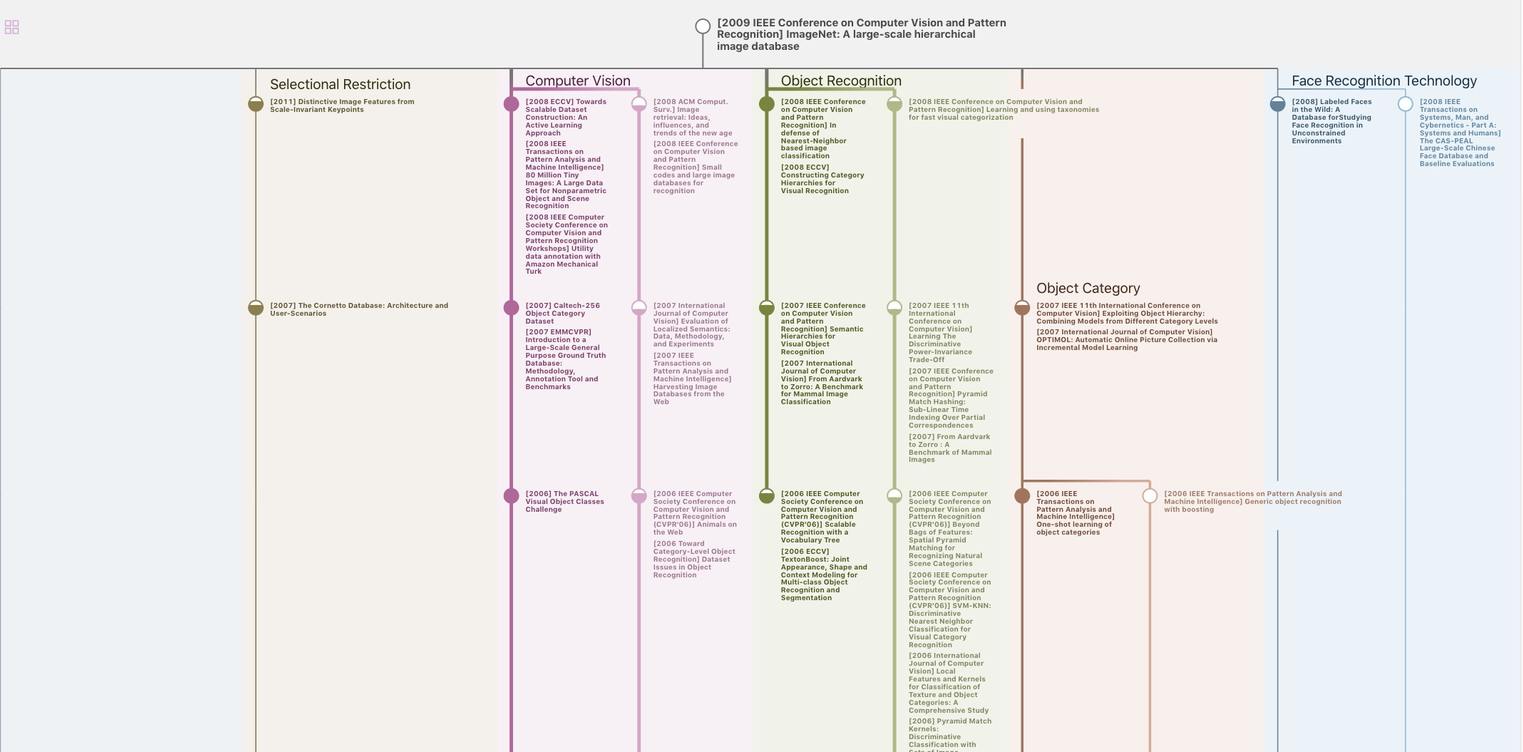
生成溯源树,研究论文发展脉络
Chat Paper
正在生成论文摘要