SSL-Net: Sparse semantic learning for identifying reliable correspondences
PATTERN RECOGNITION(2024)
摘要
Feature matching aims to identify reliable correspondences between two sets of given initial feature points, which is of considerable importance to photogrammetry and computer vision. In this study, we propose an innovative sparse semantic learning-based network, named SSL-Net, for feature matching. Specifically, SSL-Net includes a novel sparsity constraint (SC) block, which builds a sparse graph for sparse semantic learning. The SC block adopts a region-to-whole learning strategy to measure the confidence of nodes in the sparse graph. It helps the sparse graph preserve the semantic information of positive influence while rejecting unnecessary ones, thereby suppressing the negative influence of incorrect correspondences. In addition, SSL-Net also includes a channel-spatial attention feature gathering block, which gathers features along the spatial direction and channel dimension of correspondences. To mitigate the existence of label ambiguity, we incorporate the accommodation factor into the loss function of SSL-Net for feature matching. As a result, our network outperforms the state-of-the-art method by a considerable margin. Notably, SSL-Net achieves a 9.05% improvement under an error threshold of 5 degrees over the state-of-the-art method for the relative pose estimation task on the YFCC100M dataset. Our code will be available at https://github.com/guobaoxiao/SSL-Net.
更多查看译文
关键词
Feature matching,Deep learning,Outlier rejection,Attention mechanism
AI 理解论文
溯源树
样例
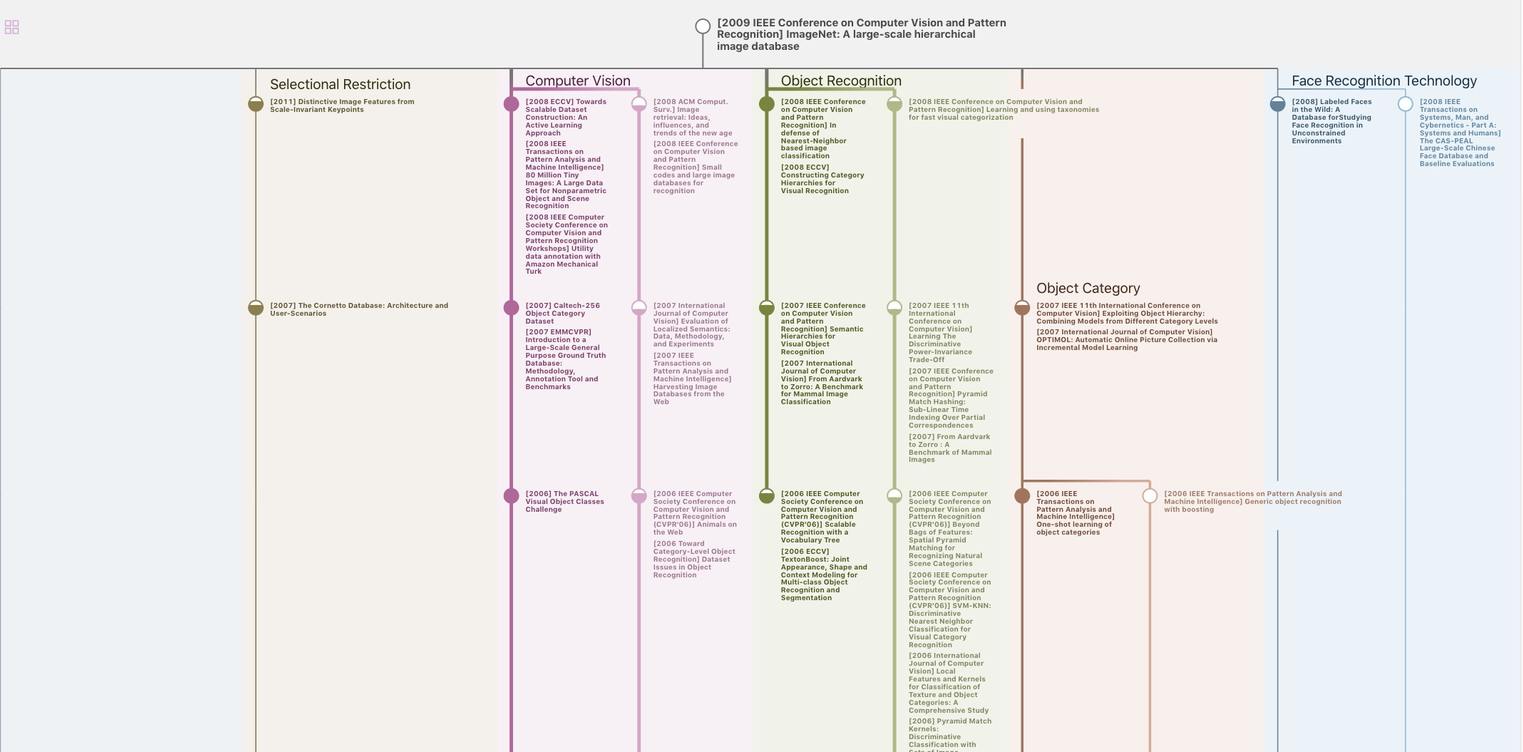
生成溯源树,研究论文发展脉络
Chat Paper
正在生成论文摘要