GITGAN: Generative inter-subject transfer for EEG motor imagery analysis
PATTERN RECOGNITION(2024)
摘要
Domain adaptation (DA) plays a crucial role in achieving subject-independent performance in Brain-Computer Interface (BCI). However, previous studies have primarily focused on developing intricate network architecture designs, neglecting the impact of source data quality and the challenges posed by the out-of-distribution target data problem. To address these limitations, we argue that a target data-centered space, augmented by a carefully selected set of high-quality source data, can significantly enhance DA. In this study, we present an unsupervised end-to-end subject adaptation approach called GITGAN, a generative inter-subject transfer for electroencephalography motor imagery analysis. We also propose a practical and effective method for selecting source data, which further enhances performance. Our approach is non-intrusive, as it does not modify the target data distribution, thus preserving its integrity for further numerical and visual analysis. Our extensive experiments with two different datasets demonstrate not only the superiority of our approach compared to existing methods, but also its phenomenal potential for practical BCI applications. The findings of this study provide valuable insights into the potential of BCI and illustrate the importance of considering source data quality in DA. The implementation is available at https://github.com/Kang1121/GITGAN.
更多查看译文
关键词
Brain-computer interface (BCI),Electroencephalogram (EEG),Unsupervised domain adaptation (UDA),Generative adversarial learning
AI 理解论文
溯源树
样例
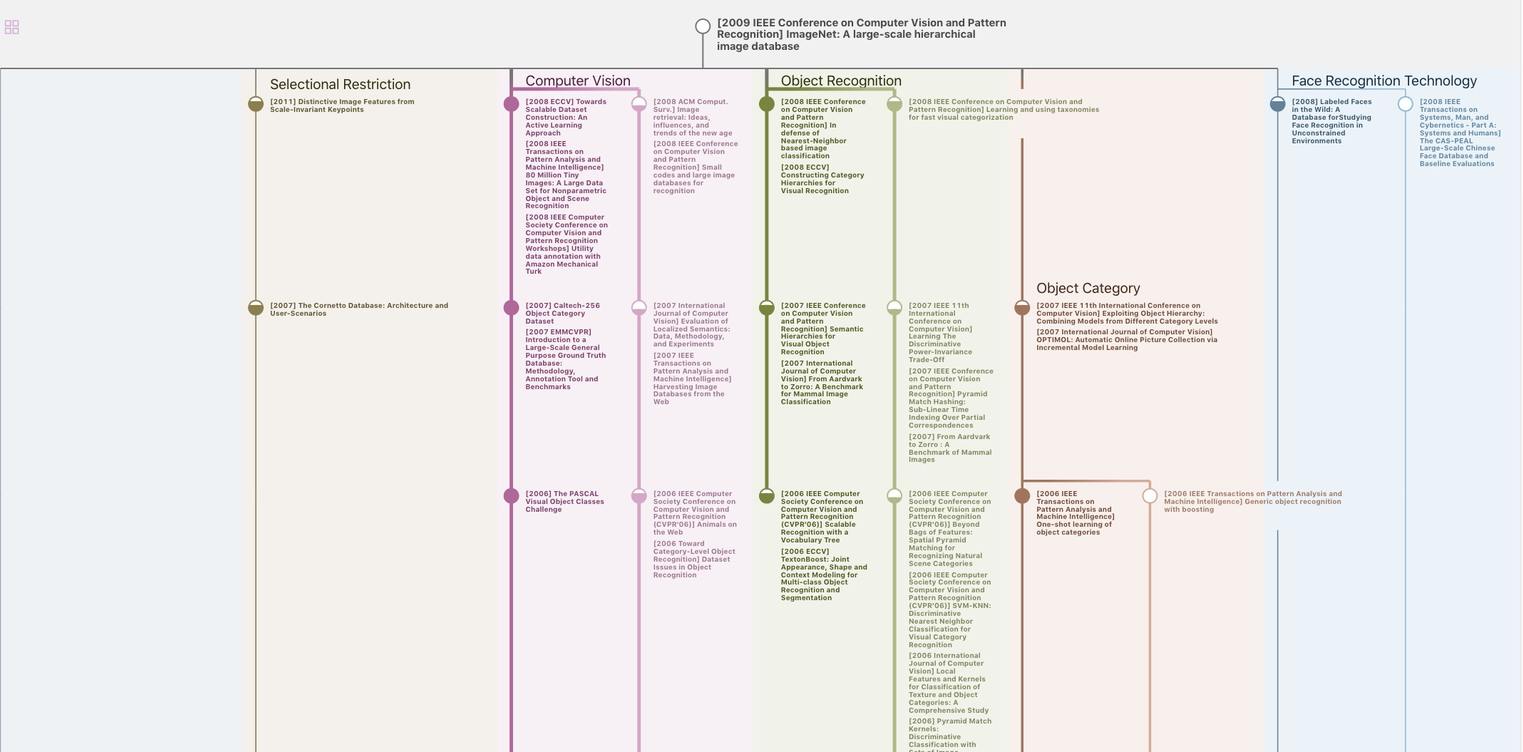
生成溯源树,研究论文发展脉络
Chat Paper
正在生成论文摘要