Multiple instance learning from similarity-confidence bags
PATTERN RECOGNITION(2024)
摘要
Multiple instance learning (MIL) is a classic weakly supervised learning approach, in which samples are grouped into bags that may contain varying numbers of instances. A bag is designated as positive if it contains at least one positive instance; otherwise, it is considered negative. Previous studies have consistently assumed that the bag labels are completely known. In fact, labeling every bag can be extremely challenging or even unfeasible due to the exorbitant expenses in terms of time and labor. Fortunately, it is much easier to obtain the similarity confidence, which represents the probability of two bags sharing the same label. How to employ it in MIL is worthy of study. Inspired by the above study, we present the first attempt to investigate MIL from similarity-confidence bags. Therefore, this paper proposes a new framework for training bag-level classifiers that adheres to the principle of empirical risk minimization. Moreover, we theoretically derive a generalization error bound to guarantee model convergence. Finally, we implement risk correction to mitigate potential over -fitting problem and provide theoretical consistency. Numerical experiments on eight datasets further validate the effectiveness of the proposed bag-level classifier.
更多查看译文
关键词
Multiple instance learning,Similarity-confidence,Empirical risk minimization
AI 理解论文
溯源树
样例
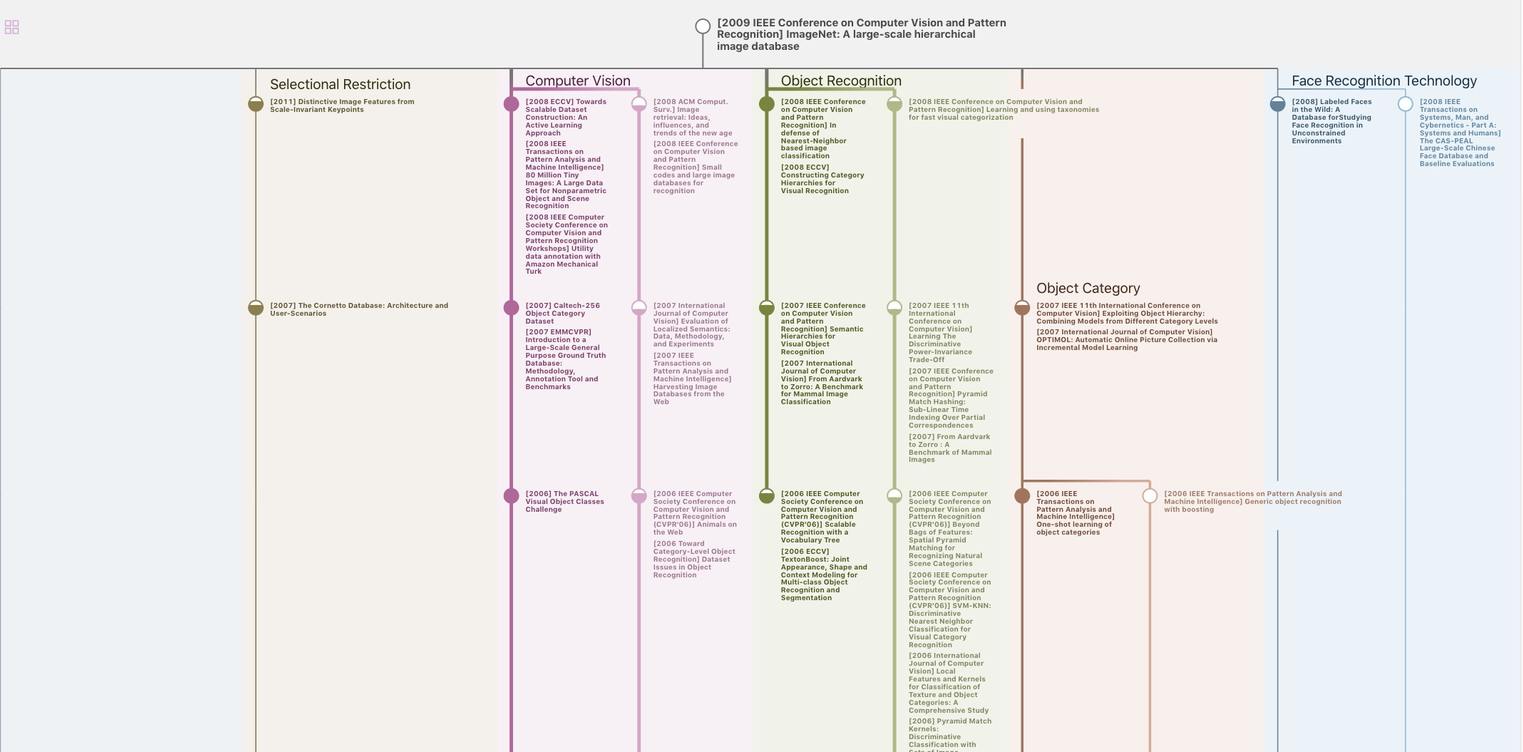
生成溯源树,研究论文发展脉络
Chat Paper
正在生成论文摘要