STF-Trans: A two-stream spatiotemporal fusion transformer for very high resolution satellites images
NEUROCOMPUTING(2024)
摘要
Spatiotemporal satellite image fusion is regarded as an effective approach to address the limitations of a single optical sensor and generate data with high spatial and temporal resolutions. Thanks to the recent advances of Deep Learning, spatiotemporal fusion has gained a significant performance improvement. However, most models require at least three inputs, including a high resolution one at a prior date, to make the prediction at the desired date. Such a requirement is not always guaranteed in practice, in particular due the high cost of high resolution image and bad atmospheric conditions. In the last few years, Transformers have achieved tremendous performance on several computer vision tasks compared to convolutional neural networks. Inspired by the new trend, we proposed an end-to-end two-stream SpatioTemporal fusion technique based on encoder-decoder transformer architecture, termed STF-Trans. To deal with a complexity and high cost of data preparation, the proposed approach aims to fuse a coarse-resolution image with high temporal resolution, and a very high resolution Google Earth image, to produce a very high resolution image at the desired date. The proposed technique employs a two-stream convolutional network to capture both temporal and spatial features from the inputs, and then the encoder-decoder transformer captures long-range dependency of the latter and generates a more efficient image representation from the shallow features. Finally, a shallow network maps the features into the desired fused image. The experimental results show that the proposed method outperforms the current state-of-the-art techniques at both quantitative and qualitative evaluations.
更多查看译文
关键词
Transformers,Satellite image fusion,Spatiotemporal fusion,Time-series
AI 理解论文
溯源树
样例
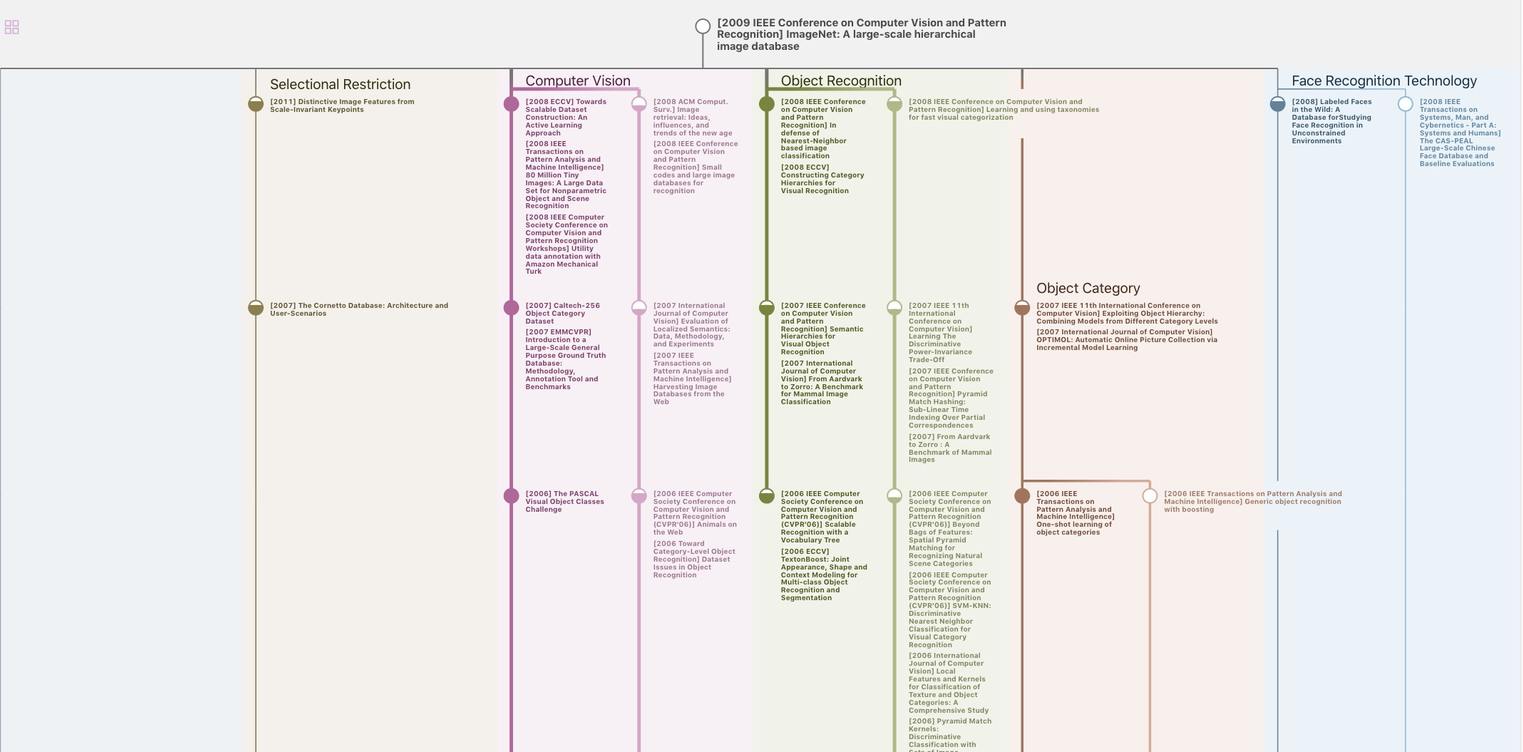
生成溯源树,研究论文发展脉络
Chat Paper
正在生成论文摘要