Enhancements for stabilizing functional in potential field inversion to recover sparse models with reasonable values and dipping structures
Journal of Applied Geophysics(2023)
摘要
Regularization theory is a powerful tool for addressing the ill-posed problem of potential field inversion. For this purpose, various types of stabilizing functionals have been applied in potential field inversion to obtain smooth or sparse models. However, the smooth constrained inversion using a quadratic stabilizer often generates results with fuzzy boundaries and underestimated values, while the results of sparse inversion depend too much on the constraint or appear to have a staircasing problem with either horizontal or vertical boundaries. To better un-derstand the problems and provide some solutions, we analyze several reweighting functions in the unifying inversion framework and propose two improvements to the stabilizing functional for sparse inversion. For the stabilizing functional of model parameters, an L0E norm stabilizing functional is adopted to increase the constraint weight of high absolute values to ameliorate the bound dependence problem. For the stabilizing functional of the model parameter gradients, a reformulated modulus constraint is used instead of the component constraint to avoid the staircasing phenomenon in the inversion results. Moreover, a combined regularization scheme is performed to integrate the benefits of the two proposed stabilizing functionals. The inversion results based on model data show that this combined method alleviates the dependence on the bound constraint and obtains sparse models with reasonable values and dipping structures. Finally, we apply this approach to real gravity gradient data collected from the Decorah region in northwestern Iowa, illustrating that this method can better recover sparse results with dipping structures.
更多查看译文
关键词
Potential field,Gravity inversion,Sparse regularization,Stabilizing functional
AI 理解论文
溯源树
样例
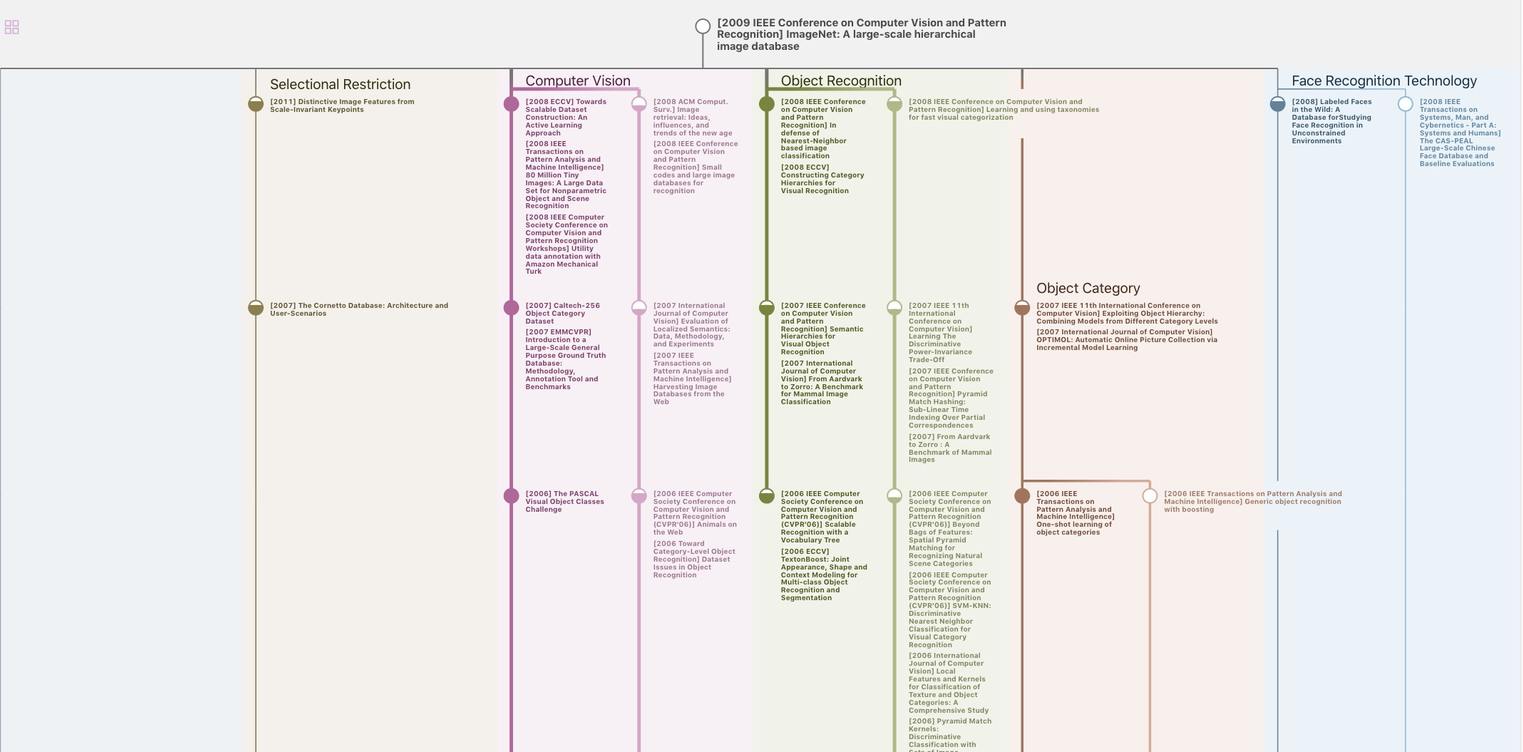
生成溯源树,研究论文发展脉络
Chat Paper
正在生成论文摘要