A learnable EEG channel selection method for MI-BCI using efficient channel attention
Frontiers in Neuroscience(2023)
摘要
IntroductionDuring electroencephalography (EEG)-based motor imagery-brain-computer interfaces (MI-BCIs) task, a large number of electrodes are commonly used, and consume much computational resources. Therefore, channel selection is crucial while ensuring classification accuracy.MethodsThis paper proposes a channel selection method by integrating the efficient channel attention (ECA) module with a convolutional neural network (CNN). During model training process, the ECA module automatically assigns the channel weights by evaluating the relative importance for BCI classification accuracy of every channel. Then a ranking of EEG channel importance can be established so as to select an appropriate number of channels to form a channel subset from the ranking. In this paper, the ECA module is embedded into a commonly used network for MI, and comparative experiments are conducted on the BCI Competition IV dataset 2a.Results and discussionThe proposed method achieved an average accuracy of 75.76% with all 22 channels and 69.52% with eight channels in a four-class classification task, outperforming other state-of-the-art EEG channel selection methods. The result demonstrates that the proposed method provides an effective channel selection approach for EEG-based MI-BCI.
更多查看译文
关键词
brain-computer interface,motor imagery,channel selection,deep learning,attention mechanism
AI 理解论文
溯源树
样例
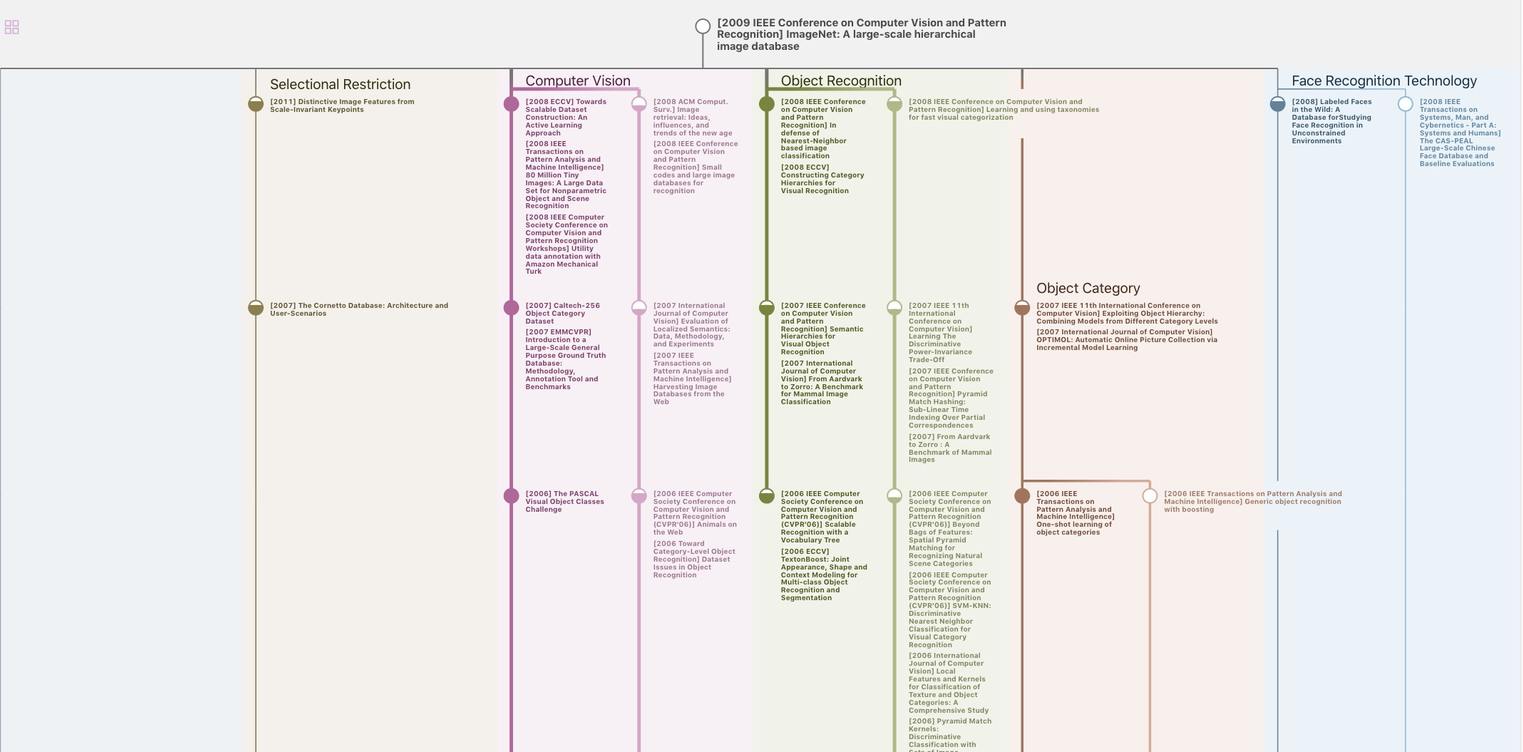
生成溯源树,研究论文发展脉络
Chat Paper
正在生成论文摘要