Hybrid unsupervised paradigm based deformable image fusion for 4D CT lung image modality
INFORMATION FUSION(2024)
摘要
Deformable image registration plays a critical role in various clinical applications (e.g., image fusion, atlas creation, and tumors targeting). In radiation therapy, especially in the context of fast registration of computed tomography (CT) lung image modalities, it is used to determine the geometric transformation by relating the anatomic points in two images. The main challenge lies in effectively addressing the nonlinear large and small deformation between the inspiration and expiration phases. In this work, we propose an unsupervised hybrid paradigm-based registration network (HPRN) for the registration of 4D CT lung images without relying on ground truth data. The proposed HPRN exhibits effective learning of multi-scale and multi-resolution features, leading to the computation of a more accurate Deformation Vector Field (DVF). Furthermore, we incorporate the regularization, image similarity and Jacobian determinant loss functions, which results in improving capability in dealing with complex large and small deformations. We evaluate the effectiveness of the proposed model on the publicly accessible DIRLab 4DCT lung image dataset, which shows the effectiveness of the proposed framework by achieving better Target Registration Error (2.04 +/- 1.42 mm) compared to other state-of-the-art unsupervised image registration algorithms.
更多查看译文
关键词
Deep learning,Image fusion,Lung 4D CT,Multi resolution,Deformation,Image registration
AI 理解论文
溯源树
样例
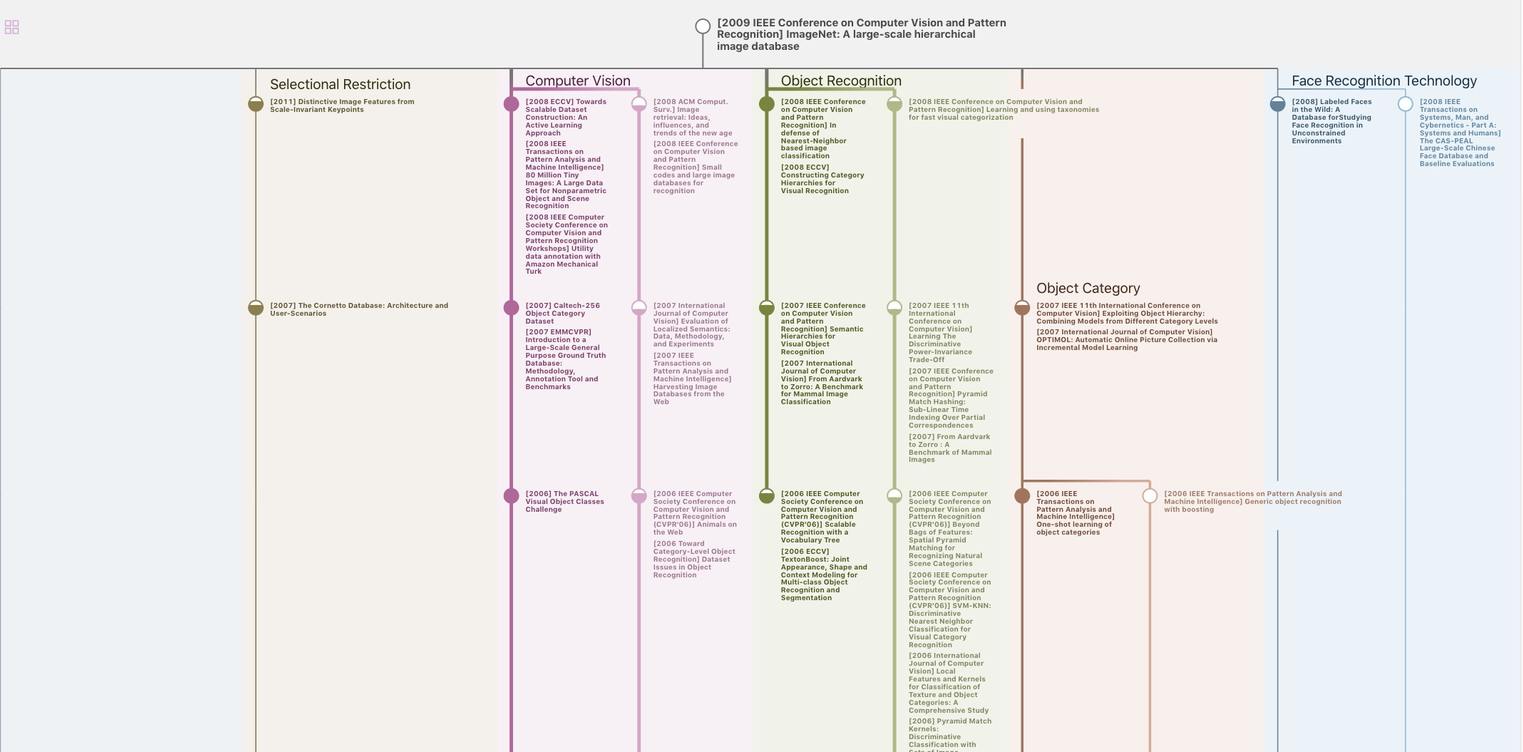
生成溯源树,研究论文发展脉络
Chat Paper
正在生成论文摘要