A multi-objective segmentation method for chest X-rays based on collaborative learning from multiple partially annotated datasets
INFORMATION FUSION(2024)
摘要
Accurate segmentation of multiple targets, such as ribs, clavicles, heart, and lung fields, from chest X-ray images is crucial for diagnosing various lung diseases. Currently, mainstream deep learning methods heavily rely on fully annotated large-scale datasets. However, annotating all objects in chest X-ray images is a labor-intensive and time-consuming task. The publicly available partially annotated chest X-ray datasets have varying annotation objects and standards, and they are seldom utilized comprehensively in existing studies. To address these challenges, we propose A Multi-objective Segmentation Method for chest X-rays based on Collaborative Learning from Multiple Partially Annotated Datasets (called: MSM-CLMPAD). Our approach first utilizes an encoder constructed with densely connected blocks to extract multi-scale features from multiple partially annotated datasets. Then, leveraging the overlapping relationships among segmentation targets, we design a dual decoder guided by the attention mechanism. The novel attention-guided decoder effectively disentangles the features corresponding to various targets. Importantly, we propose an alternating training strategy for different datasets, facilitating collaborative learning of the same network model across datasets with diverse annotation targets. The experimental results performing on four public datasets demonstrate that our method achieves superior Dice and Jaccard coefficients compared to other popular methods, particularly for overlapping targets and unclear regions. We also explore the mutual influence of different targets in chest X-rays, offering a solution for interaction among partial datasets and further alleviating the difficulties of data annotation for multi-organ segmentation tasks.
更多查看译文
关键词
Chest X-rays,Multi-object segmentation,Partially annotated data,Medical image analysis
AI 理解论文
溯源树
样例
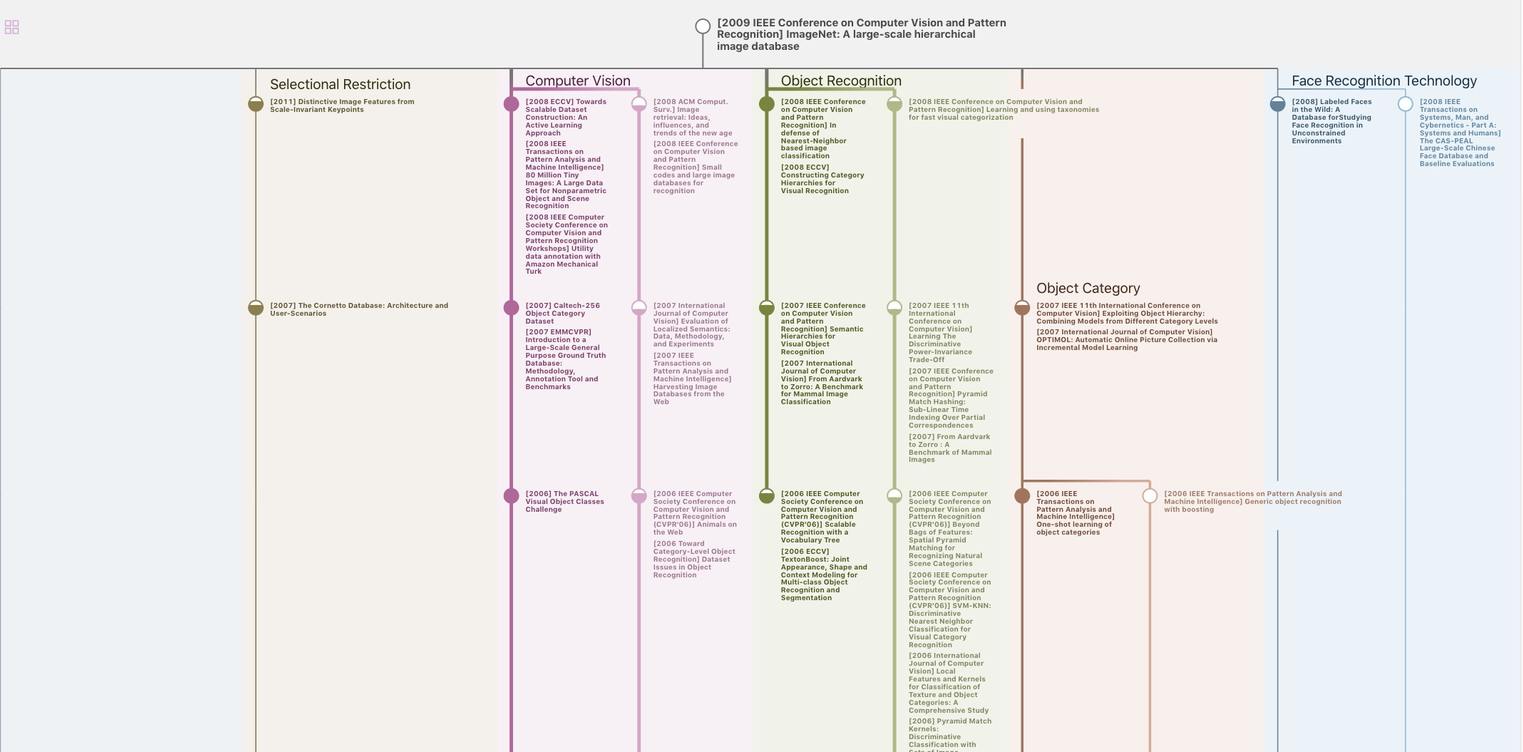
生成溯源树,研究论文发展脉络
Chat Paper
正在生成论文摘要