Hierarchical graph augmented stacked autoencoders for multi-view representation learning
INFORMATION FUSION(2024)
摘要
With recent success of deep neural networks, stacked autoencoder networks have received a lot of attention for robust unsupervised representation learning. However, recent autoencoder methods cannot make full use of multi-view information and thus fail to further improve many real-world applications by exploring the geometric structures of multi-view data. To address the above-mentioned issue, we introduce hierarchi-cal graph augmented stacked autoencoders (HGSAE) for unsupervised multi-view representation learning. Specifically, a hierarchical graph structure is first adapted to stacked autoencoders to learn view-specific representations, aiming to preserve the geometric information of multi-view data through local and non-local graph regularizations. A general or common representation can then be learned by reconstructing each single view using fully connected neural networks. By doing this, the proposed method not only preserves the geometric information in multi-view data but also automatically balances the complementarity/consistency among different views. Extensive experiments on six popular unsupervised representation learning datasets demonstrate the effectiveness of our method when compared with recent state-of-the-art autoencoder methods.
更多查看译文
关键词
Autoencoder,Graph regularized autoencoder,Multi-view representation learning,Deep learning
AI 理解论文
溯源树
样例
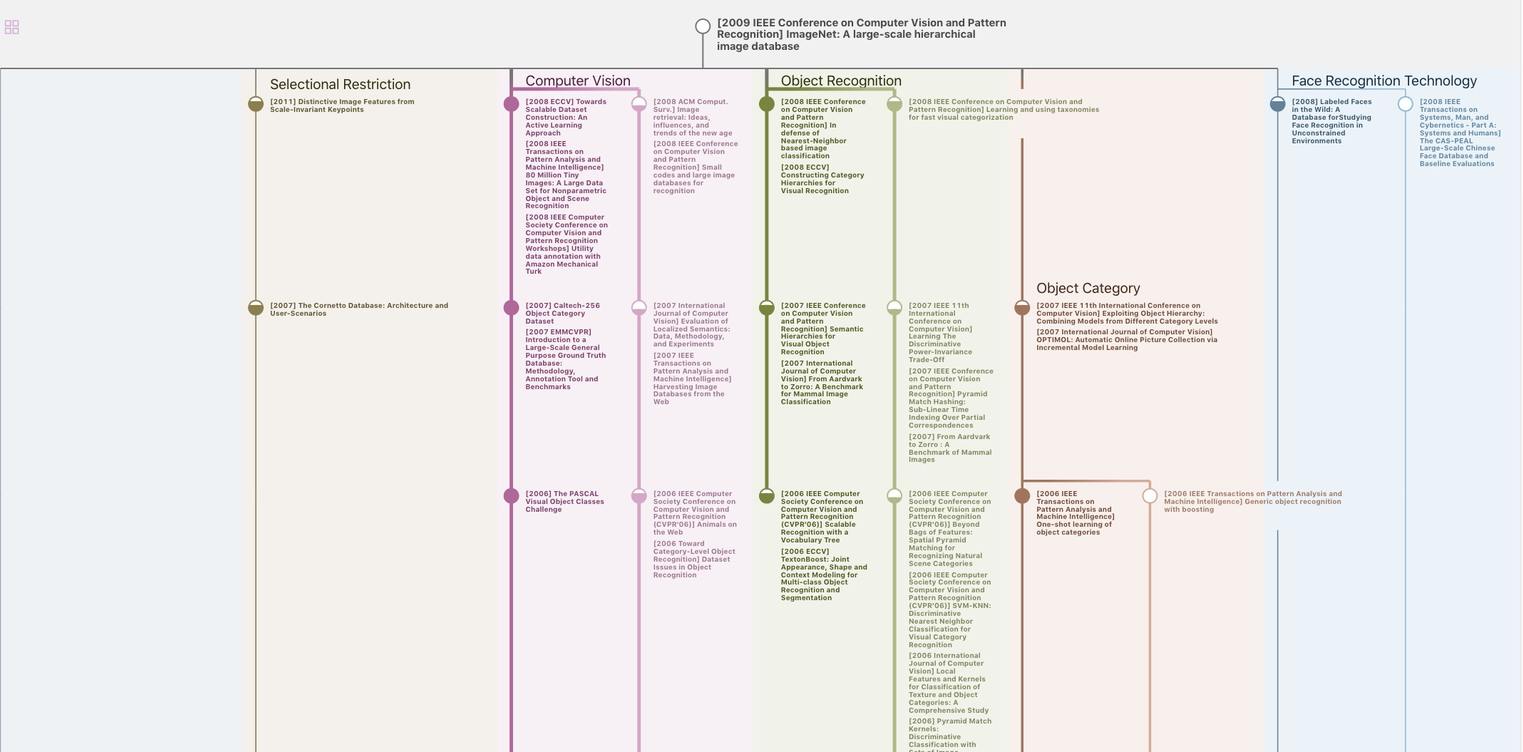
生成溯源树,研究论文发展脉络
Chat Paper
正在生成论文摘要