A generalization of multi-source fusion-based framework to stock selection
INFORMATION FUSION(2024)
摘要
Selecting outstanding technology stocks for investment is challenging. Specifically, the research of the investment for academic purposes is not mature enough due to the disarray of the publications and the overwhelming informative experience from profit-making websites. Often, some authors entitle the stock price prediction as a stock selection problem; both prediction and selection are just sub-sections of portfolio management. Moreover, stock websites provide numerous potential criteria showing various evaluations to simulate and monitor the stock market professionally, which increases the difficulty of academic studies on stock selection.The paper generalizes a novel framework with a user-interactive interface for stock selection problems based on multi-source data fusion and decision-level fusion to enhance reliability limited by the narrow criteria performance and the strength of a model overcoming the weakness of a single-performed model. This framework benefits the time-series prediction and decision-making study. Besides, we propose adopting dynamic time warping to assist a task-learning process by customizing a loss function that improves the accuracy of data prediction. The experiment shows that the proposed method reduces the prediction log error by 6.3% on average and decreases the warping cost by 5.6% on average over all cases of real-situation data. Finally, we illustrate the proposed framework by implementing it in a real-world stock data selection. The results are practical and effective, further justified through a detailed ablation study. The source code will be available at https://github.com/lingping-fuzzy.
更多查看译文
关键词
Stock selection,Multi-source fusion,Multi-criteria decision-making,Time-series prediction,Dynamic time warping
AI 理解论文
溯源树
样例
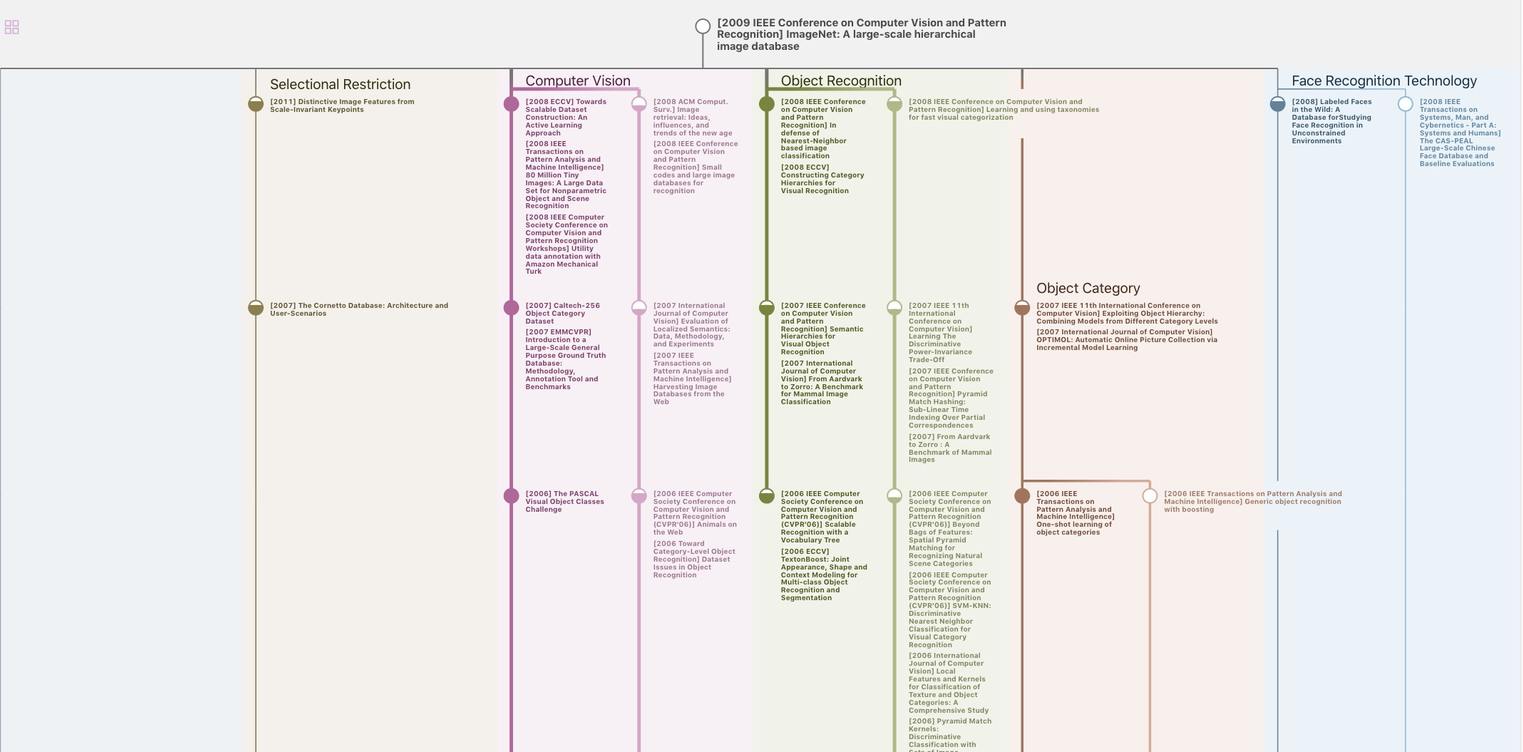
生成溯源树,研究论文发展脉络
Chat Paper
正在生成论文摘要