Geo-aware graph-augmented self-attention network for individual mobility prediction
FUTURE GENERATION COMPUTER SYSTEMS-THE INTERNATIONAL JOURNAL OF ESCIENCE(2024)
摘要
Even though some studies have found encouraging results, the sparsity of data and the complexity of mobility patterns remain significant challenges in predicting individual mobility. In order to effectively address the two challenges, we propose a novel framework called Geo-aware Graph-Augmented Self-Attention Network (GaGASAN). In GaGASAN, we construct a heterogeneous location graph consisting of a geospatial subgraph and a location transition subgraph to simultaneously model the impacts of geospatial distance and location transitions on all users. With the heterogeneous location graph, the impacts of geospatial distances and global location transitions of all users can be effectively merged, thereby mitigating the sparsity of individual mobility data. For the complex and variable mobility patterns of individuals, we employ a multi-scale time encoding technique and a self-attention mechanism to model different temporal patterns and capture long- and shortrange contexts of sequence transitions. Extensive experiments shows that GaGASAN significantly outperforms eight baseline methods on all metrics. Our implementation code is available at https://github.com/mrcwyh/GaGASAN.
更多查看译文
关键词
Mobility prediction,Mobility pattern,Self-attention mechanism,Heterogeneous Graph Convolution Network,Trajectory,Time encoding
AI 理解论文
溯源树
样例
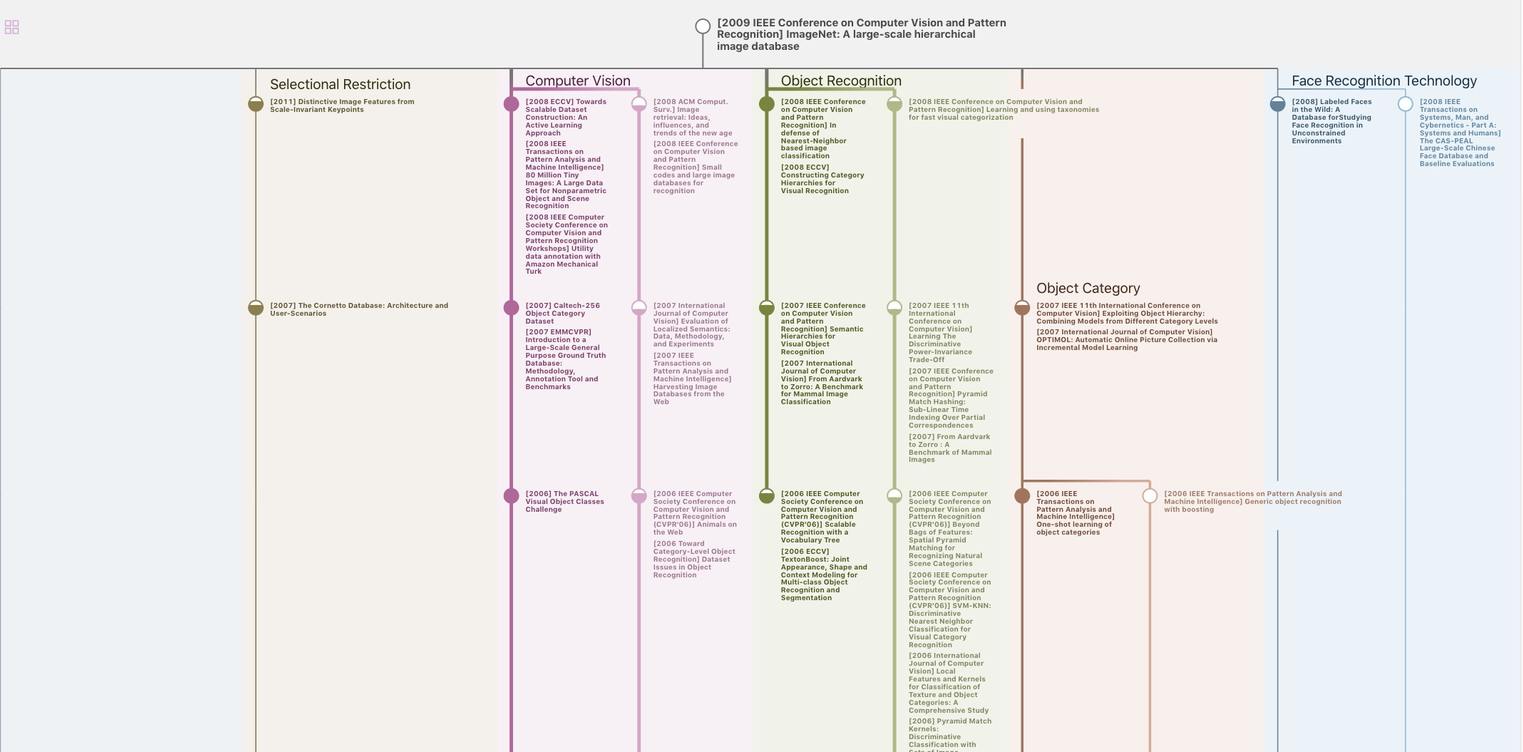
生成溯源树,研究论文发展脉络
Chat Paper
正在生成论文摘要