Attention based adaptive spatial-temporal hypergraph convolutional networks for stock trend
EXPERT SYSTEMS WITH APPLICATIONS(2024)
摘要
Stock price trend prediction is an important and challenging issue, and accurate forecasting will effectively improve investment decisions and contribute to investment returns. Improving prediction accuracy by exploring stock correlations has received much attention in recent studies. However, there are still some issues that have not been fully considered, such as the impact of invalid correlations, low sensitivity to minor price fluctuations and dependence on priors expert information. To solve the above issues, we propose a novel spatial-temporal framework, which has several characteristics: (1) the noise-aware spatial-temporal attention that dynamically filters out invalid associations from traditional spatial attention and combines temporal attention to capture the spatial-temporal patterns of different stock series; (2) the adaptive stock hypergraph generation maps the intrinsic associations of stocks into a trainable dense matrix via adaptive node embedding; (3) the adaptive graph convolution extends the graph convolution operation from static graphs to adaptive hypergraphs for exploring the dynamic correlations. (4) Multiple stacked attention-based adaptive spatial-temporal Blocks form the end-to-end prediction framework, which uses time-aware cascaded convolution to extract fine-grained temporal features. Convincing experimental results on two stock datasets, studies on the performance on various simulation investments and the model interpretability confirm the advantages of our approach.
更多查看译文
关键词
Stock price trend prediction,Spatial-temporal attention,Spatial-temporal pattern,Adaptive graph convolution,Temporal convolution
AI 理解论文
溯源树
样例
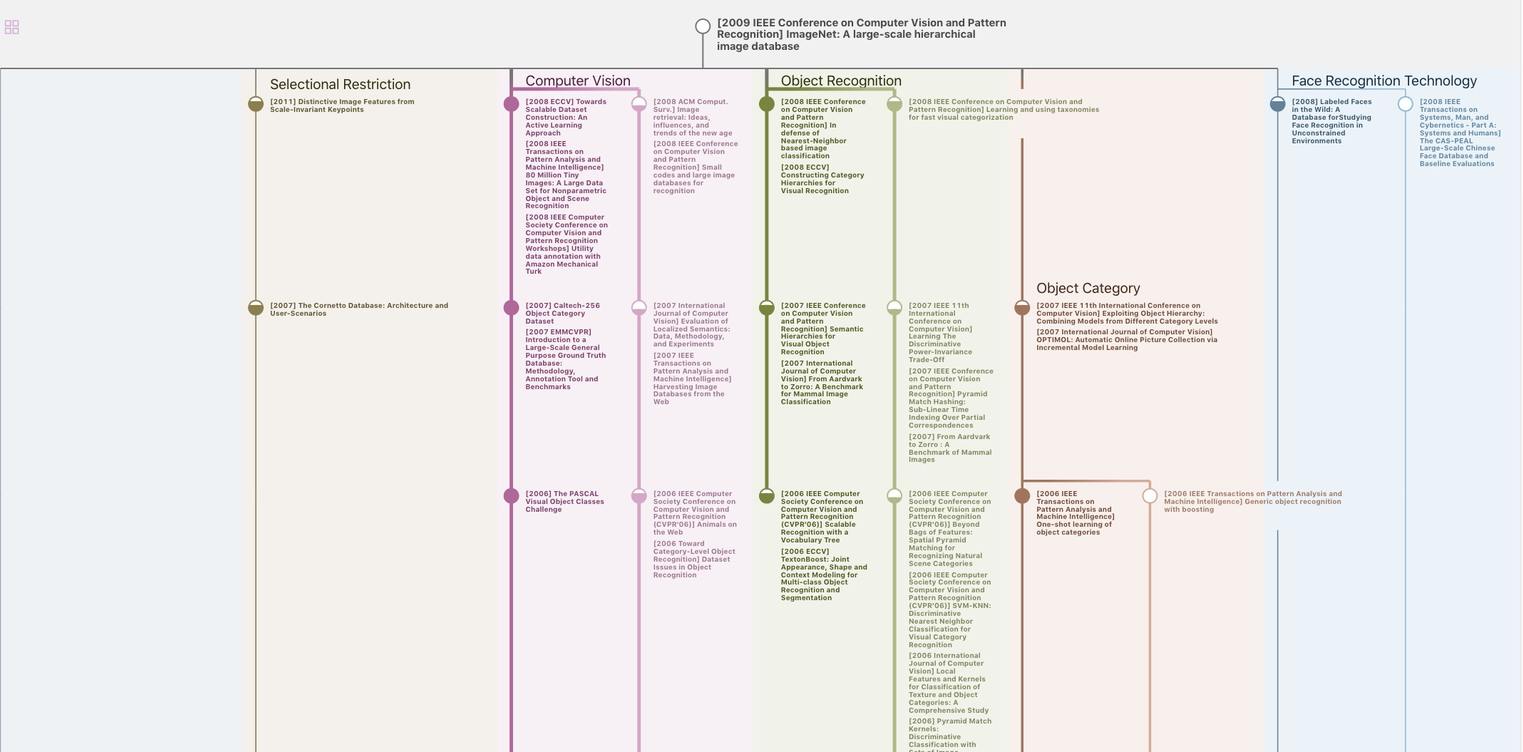
生成溯源树,研究论文发展脉络
Chat Paper
正在生成论文摘要