Image expression of time series data of wearable IMU sensor and fusion classification of gymnastics action
EXPERT SYSTEMS WITH APPLICATIONS(2024)
摘要
Human activity recognition (HAR) based on multimodal wearable motion sensors is a valuable technology, and gymnastics action recognition is one of its important application scenarios. However, this field faces challenges such as excessive sensor node deployment, hindering effective utilization of time-series sensor data, and low accuracy in action recognition. To address these issues, this paper introduces a gymnastics action recognition system comprising a hardware setup and an action recognition framework that combines a time series data imaging method with a novel three-channel convolutional model ViTGS. Firstly, a cost-effective single-node sensor system equipped with a 6-axis IMU is devised to efficiently capture gymnastics action data. Secondly, three one-dimensional time series data image encoding methods, Gramian Angular Field (GAF), Recurrence Plot (RP), and Markov Transition Field (MTF), are employed to capture the temporal features, phase space features, and state transition features of the data respectively. Finally, the ViTGS model is proposed, which uses the ViT_B/16 network to extract the features of each channel image, and uses Gated Fusion Network (GFN) to realize the effective fusion of different channel features and uses SVM as the recognition layer to further improve the classification accuracy. The experimental results show that using single-node (left wrist) sensor data, an accuracy of 98.56% is obtained on the acceleration dataset, and an accuracy of 99.04% is obtained on the angular velocity dataset. The method of this paper is not only applicable to gymnastics action recognition but also can be generalized to other human action recognition fields.
更多查看译文
关键词
Human Activity Recognition (HAR),Wearable motion sensors,Image encoding,Time series data,Gymnastics action recognition
AI 理解论文
溯源树
样例
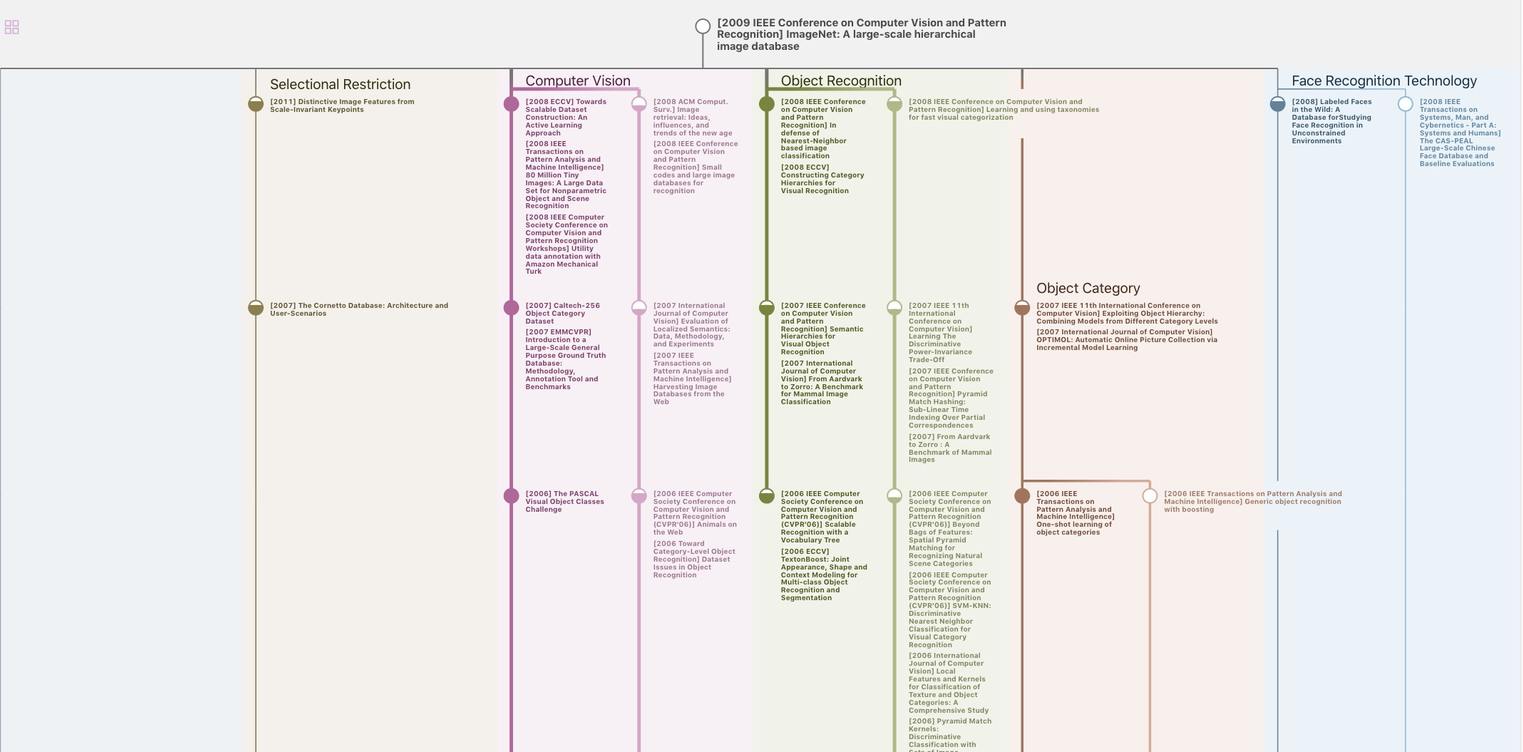
生成溯源树,研究论文发展脉络
Chat Paper
正在生成论文摘要