Inter- and intra-uncertainty based feature aggregation model for semi-supervised histopathology image segmentation
EXPERT SYSTEMS WITH APPLICATIONS(2024)
摘要
Acquiring pixel-level annotations is often limited in applications such as histology studies that require domain expertise. Various semi-supervised learning approaches have been developed to work with limited ground truth annotations, such as the popular teacher-student models. However, hierarchical prediction uncertainty within the student model (intra-uncertainty) and image prediction uncertainty (inter-uncertainty) have not been fully utilized by existing methods. To address these issues, we first propose a novel inter-and intra-uncertainty regularization method to measure and constrain both inter-and intra-inconsistencies in the teacher-student architecture. We also propose a new two-stage network with pseudo-mask guided feature aggregation (PG-FANet) as the segmentation model. The two-stage structure complements with the uncertainty regularization strategy to avoid introducing extra modules in solving uncertainties and the aggregation mechanisms enable multi-scale and multi-stage feature integration. Comprehensive experimental results over the MoNuSeg and CRAG datasets show that our PG-FANet outperforms other state-of-the-art methods and our semi-supervised learning framework yields competitive performance with a limited amount of labeled data.
更多查看译文
关键词
Semi-supervised learning,Feature aggregation,Uncertainty regularization,Histopathology image segmentation
AI 理解论文
溯源树
样例
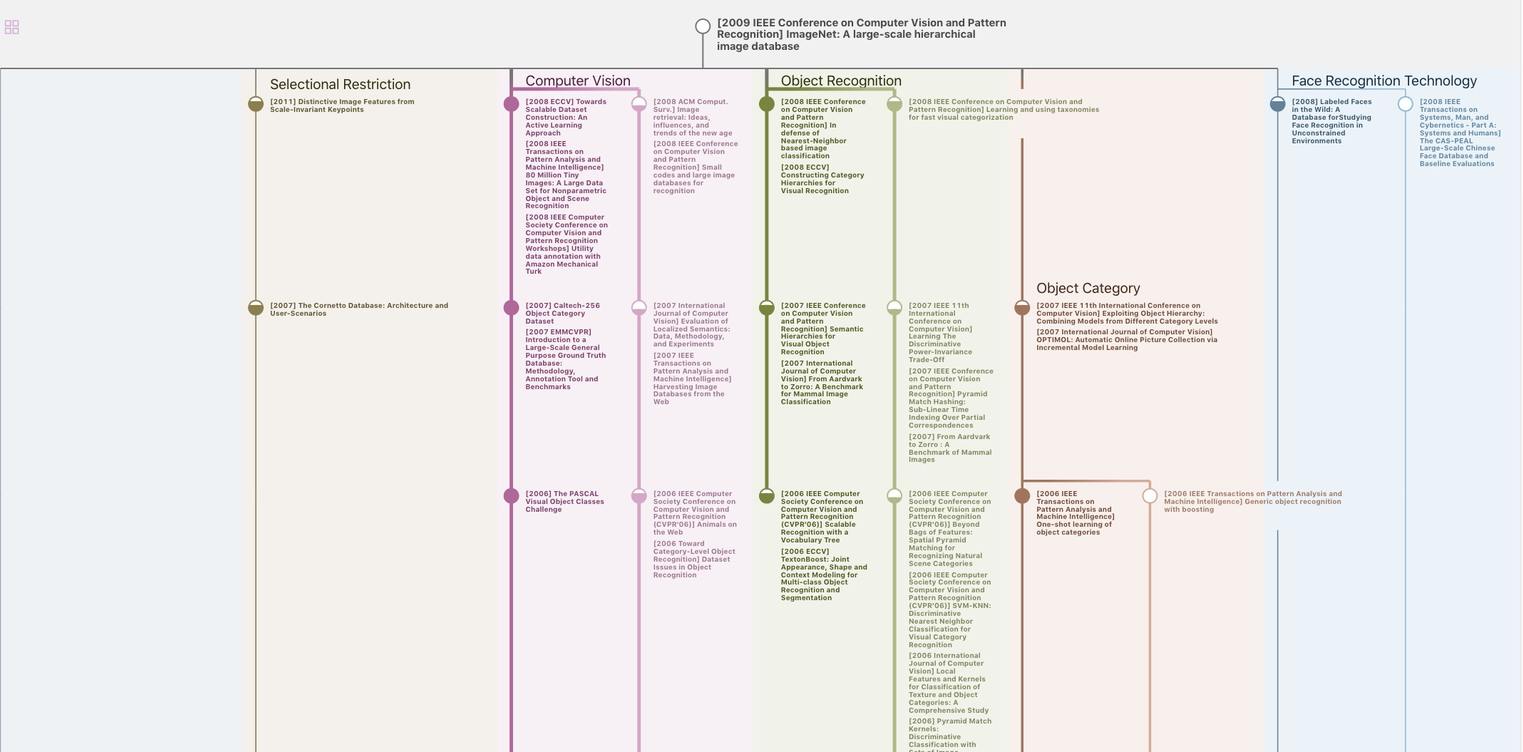
生成溯源树,研究论文发展脉络
Chat Paper
正在生成论文摘要