Chinese engineering geological named entity recognition by fusing multi-features and data enhancement using deep learning
EXPERT SYSTEMS WITH APPLICATIONS(2024)
摘要
The engineering geology report serves as a comprehensive portrayal of the geological conditions and information within a surveyed region, making it highly valuable for extracting and mining engineering geology-related knowledge. Geological Named Entity Recognition (GNER), as a pivotal technology for information extraction and knowledge discovery, aims to identify geological objects that convey significant meanings within textual data. While general NER tools and existing approaches are commonly employed for recognizing generic entities, their effectiveness is constrained by the diverse language irregularities inherent in natural language texts, including nested entities, lengthy entities, and a scarcity of domain-specific annotated corpora. Adhering to established standards and principles governing engineering geology reports, we undertake an analysis of text structures and characteristics, as well as the linguistic descriptions and data attributes. By employing an Elec-tronic Design Automation (EDA) enhancement method in conjunction with manual annotation, we construct an engineering GNER dataset. To address these linguistic irregularities, we propose a novel deep learning model that combines both the geological pre-training model (GeoBERT) and multiple features (pinyin, radical, and position vectors) to generate representations from byte sequences. These representations are subsequently fused and passed through a BiLSTM-Attention model for training. Finally, entity classification results are obtained using conditional random fields (CRF). Experimental evaluation demonstrates that the proposed model achieves an impressive F1 value of 79.60% on the constructed datasets, outperforming ten baseline models analyzed in this study.
更多查看译文
关键词
Named Entity Recognition,Engineering Geology,Pre -trained Models,Multi -feature Fusion,Deep Learning
AI 理解论文
溯源树
样例
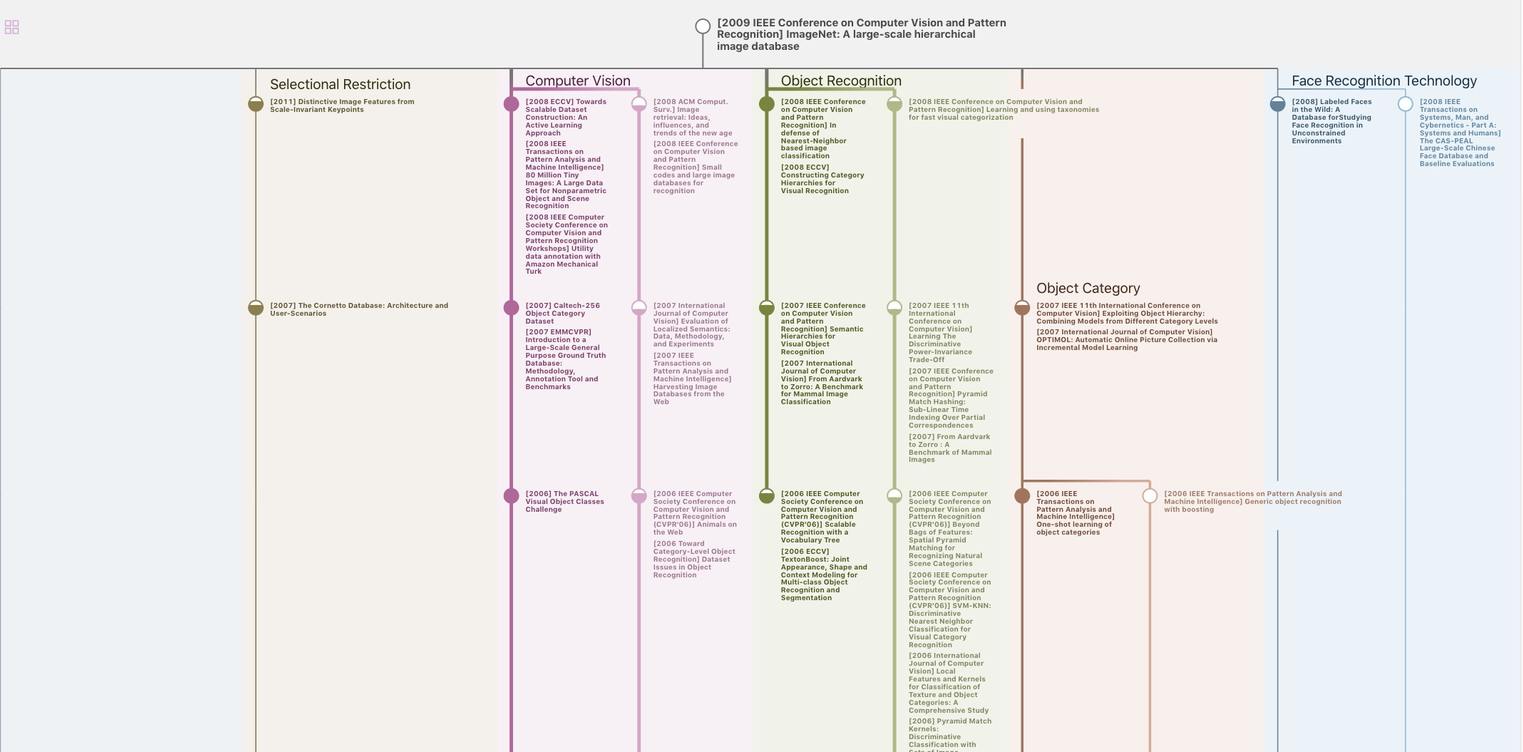
生成溯源树,研究论文发展脉络
Chat Paper
正在生成论文摘要