AdapterFusion-based multi-task learning for code-mixed and code-switched text classification
ENGINEERING APPLICATIONS OF ARTIFICIAL INTELLIGENCE(2024)
摘要
Social media text can be classified in different ways, viz sentiment analysis, humour detection, hate speech detection and hope speech detection. Multitask learning (MTL) models built on Large Language Models (LLMs) eliminate the need to build separate models for each of these tasks. However, building MTL models by fully fine-tuning the LLM has limitations such as catastrophic forgetting and requiring complete retraining to add a new task. AdapterFusion was introduced to address these limitations. However, existing AdapterFusion techniques have not been experimented with code-mixed or code-switched text. Moreover, they only considered task-based AdapterFusion on monolingual LLMs. However, using monolingual LLMs is suboptimal in classifying code-mixed or code-switched text. A better alternative is multilingual LLMs. In this paper, we present an MTL model that combines task AdapterFusion with language adapters on top of a multilingual LLM. We combine language adapters sequentially, in parallel, and as a fusion with task adapters to capture cross-lingual knowledge in code-mixed and code-switched text. We believe that this is the first research to introduce language-based AdapterFusion.
更多查看译文
关键词
Multi-task learning,Code-mixing,Code-switching,AdapterFusion
AI 理解论文
溯源树
样例
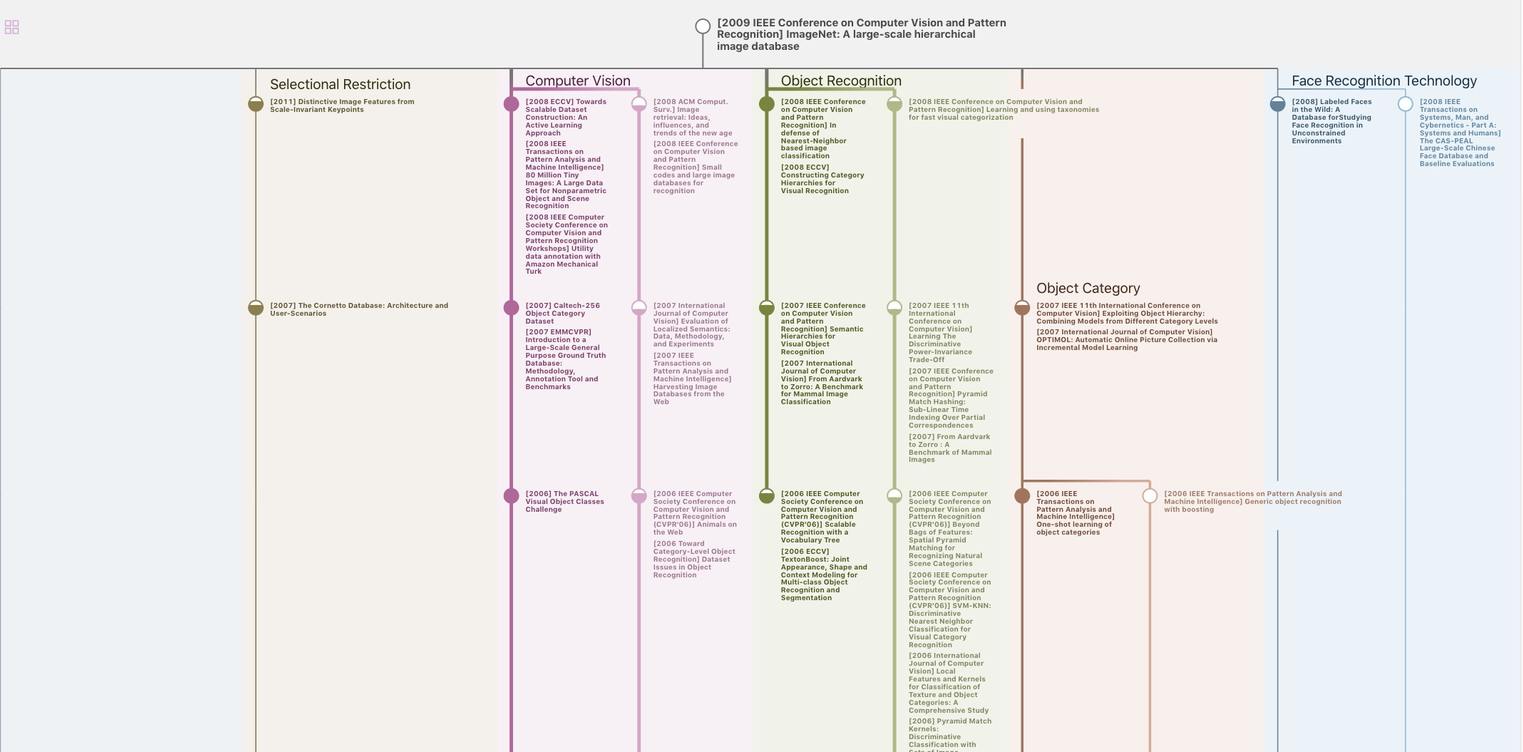
生成溯源树,研究论文发展脉络
Chat Paper
正在生成论文摘要