Quantifying the Risk of General Health and Early COVID-19 Spread in Residential Buildings with Deep Learning and Expert-augmented Machine Learning
medRxiv (Cold Spring Harbor Laboratory)(2023)
摘要
Buildings’ built environment has been linked to their occupants’ health. It remains unclear whether those elements that predisposed its residents to poor general health before the two SARS pandemics also put residents at risk of contracting COVID-19 during early outbreaks. Relevant research to uncover the associations is essential, but there lacks a systematic examination of the relative contributions of different elements in one’s built environment and other non-environmental factors, singly or jointly. Hence, the current study developed a deep-learning approach with multiple input channels to capture the hierarchical relationships among an individual’s socioecology’s demographical, medical, behavioral, psychosocial, and built-environment levels. Our findings supported that 1) deep-learning models whose inputs were structured according to the hierarchy of one’s socioecology outperformed plain models with one-layered input in predicting one’s general health outcomes, with the model whose hierarchically structured input layers included one’s built environment performed best; 2) built-environment features were more important to general health compared to features of one’s sociodemographic and their health-related quality of life, behaviors, and service utilization; 3) a composite score representing built-environment features’ statistical importance to general health significantly predicted building-level COVID-19 case counts; and 4) building configurations derived from the expert-augmented learning of granular built-environment features that were of high importance to the general health were also linked to building-level COVID-19 case counts of external samples. Specific built environments put residents at risk for poor general health and COVID-19 infections. Our machine-learning approach can benefit future quantitative research on sick buildings, health surveillance, and housing design.
Highlights
### Competing Interest Statement
The authors have declared no competing interest.
### Funding Statement
This study was funded by the Hong Kong government Strategic Public Policy Research Funding Scheme [grant number S2019.A4.015.19S].
### Author Declarations
I confirm all relevant ethical guidelines have been followed, and any necessary IRB and/or ethics committee approvals have been obtained.
Yes
The details of the IRB/oversight body that provided approval or exemption for the research described are given below:
Ethics committee/IRB of the Chinese University of Hong Kong gave ethical approval for this work
I confirm that all necessary patient/participant consent has been obtained and the appropriate institutional forms have been archived, and that any patient/participant/sample identifiers included were not known to anyone (e.g., hospital staff, patients or participants themselves) outside the research group so cannot be used to identify individuals.
Yes
I understand that all clinical trials and any other prospective interventional studies must be registered with an ICMJE-approved registry, such as ClinicalTrials.gov. I confirm that any such study reported in the manuscript has been registered and the trial registration ID is provided (note: if posting a prospective study registered retrospectively, please provide a statement in the trial ID field explaining why the study was not registered in advance).
Yes
I have followed all appropriate research reporting guidelines, such as any relevant EQUATOR Network research reporting checklist(s) and other pertinent material, if applicable.
Yes
All data produced in the present study are not available to be shared by the authors
* AUC
: area under the receiver operating curve.
CDC
: The Centers for Disease Control and Prevention of the United States.
CNN
: convolutional neural network.
COVID-19
: Coronavirus disease 2019 caused by severe acute respiratory syndrome coronavirus-2.
LSTM
: Long short-term memory.
MHHCNN
: Multi-headed hierarchical convolutional neural network. NN: neural network.
SARS
: Severe Acute Respiratory Syndrome. SES: Socio-ecological structure.
TPU
: Tertiary Planning Unit.
更多查看译文
关键词
residential buildings,deep learning,risk,machine learning,expert-augmented
AI 理解论文
溯源树
样例
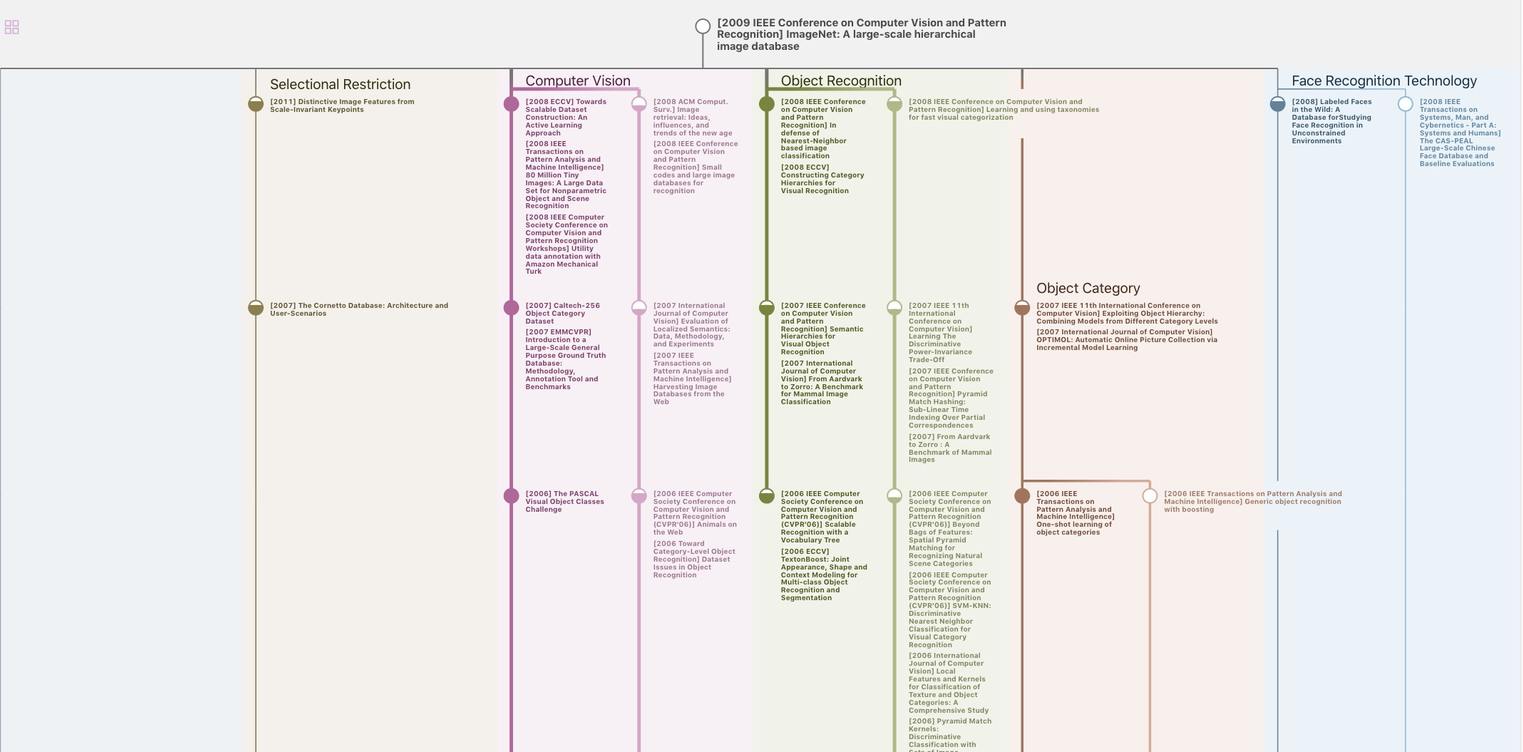
生成溯源树,研究论文发展脉络
Chat Paper
正在生成论文摘要