Phenotyping, genotyping, and prediction of abdominal pain in children using machine learning
medRxiv (Cold Spring Harbor Laboratory)(2023)
摘要
Objective Mechanisms of abdominal pain in children are not fully understood due to patient heterogeneity. We aimed to identify abdominal pain phenotypes in children to facilitate the investigation of phenotypic-genotypic associations and to determine risk factors for abdominal pain.
Design This study included 13,789 children from a large birth cohort. The comorbidities of children and mothers and single nucleotide polymorphisms in children were investigated. Machine learning (ML) was used to identify clusters of patients with homogenous characteristics; subsequently, genome-wide association studies and enrichment analyses were performed. The factors contributing to predictive models were identified using ML.
Results A total of 1,274 children experienced abdominal pain (9.2 %) (average age: 8.4 ± 1.1 years old, male/female: 615/659), who were classified into 3 clusters: cluster 1 with an allergic predisposition (n = 137), cluster 2 with mother’s comorbidities (n = 676), and cluster 3 with minimal comorbidities (n = 340). Enrichment analysis indicated that genetic pathways related to the intestinal barrier or bile acid biosynthesis were associated with abdominal pain in cluster 1; bile acid biosynthesis was also involved in cluster 2. Predictive models demonstrated modest fidelity with AUC values up to 0.65 in predicting children’s abdominal pain, showing mother’s and children’s comorbidities formed significant risk factors.
Conclusion The risk factors and phenotypes of paediatric abdominal pain are embedded within phenotype-genotype associations, which can be targeted in future studies. In particular, the link between allergy and intestinal barrier may be of mechanistic and therapeutic importance.
### Competing Interest Statement
The authors have declared no competing interest.
### Funding Statement
This study was supported by JA Niigata Kouseiren Grant (Niigata University School of Medicine).
### Author Declarations
I confirm all relevant ethical guidelines have been followed, and any necessary IRB and/or ethics committee approvals have been obtained.
Yes
The details of the IRB/oversight body that provided approval or exemption for the research described are given below:
The study used human data that are available with permission.
I confirm that all necessary patient/participant consent has been obtained and the appropriate institutional forms have been archived, and that any patient/participant/sample identifiers included were not known to anyone (e.g., hospital staff, patients or participants themselves) outside the research group so cannot be used to identify individuals.
Yes
I understand that all clinical trials and any other prospective interventional studies must be registered with an ICMJE-approved registry, such as ClinicalTrials.gov. I confirm that any such study reported in the manuscript has been registered and the trial registration ID is provided (note: if posting a prospective study registered retrospectively, please provide a statement in the trial ID field explaining why the study was not registered in advance).
Yes
I have followed all appropriate research reporting guidelines, such as any relevant EQUATOR Network research reporting checklist(s) and other pertinent material, if applicable.
Yes
All data produced in the present study are available upon reasonable request to the authors.
更多查看译文
关键词
abdominal pain,machine learning,genotyping
AI 理解论文
溯源树
样例
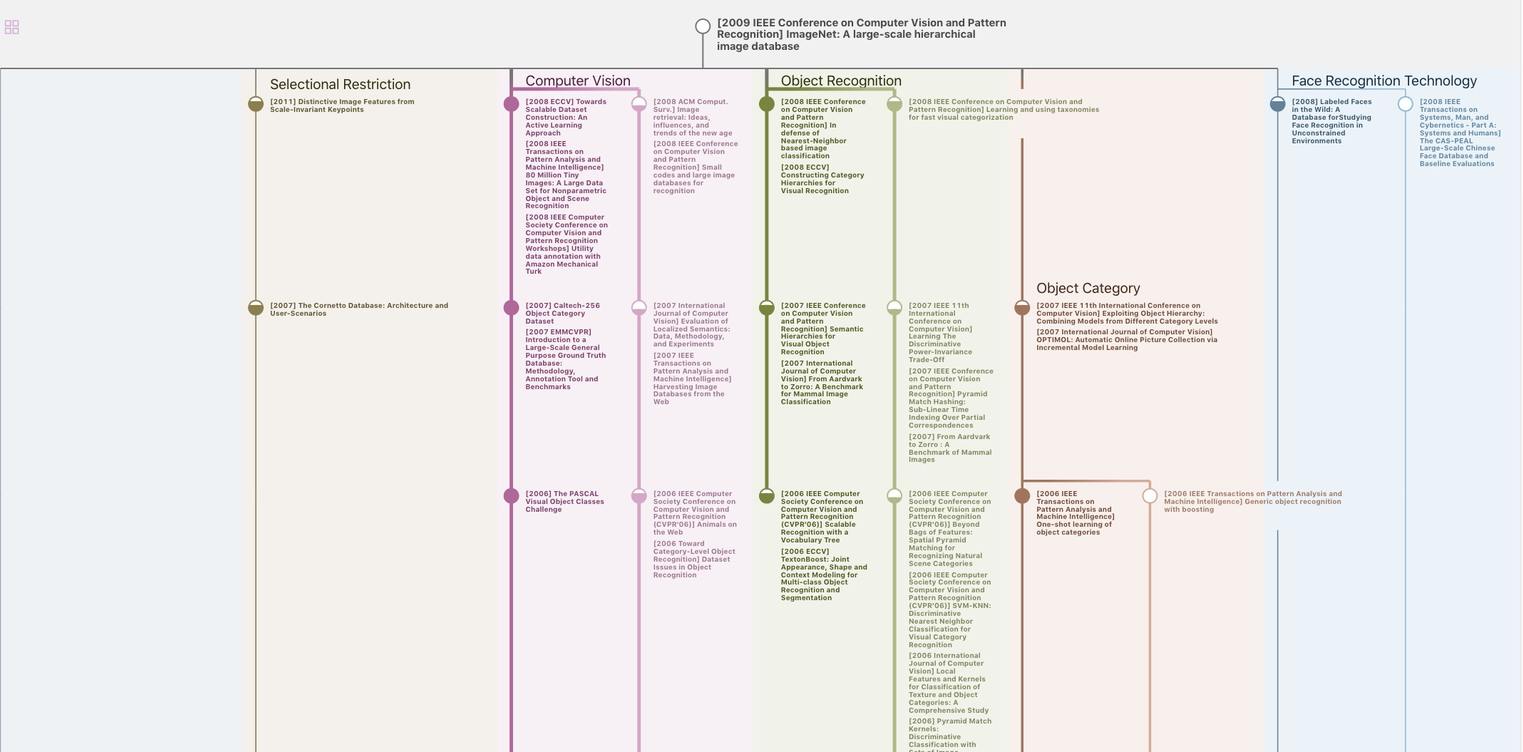
生成溯源树,研究论文发展脉络
Chat Paper
正在生成论文摘要