Simultaneous Boundary and Interior Parameterization of Planar Domains Via Deep Learning
COMPUTER-AIDED DESIGN(2024)
摘要
In isogeometric analysis (IGA), domain parameterization plays a crucial role as it significantly impacts the results of subsequent numerical analyses. Previous literature has often treated domain parameterization as two separate processes: boundary correspondence and interior parameterization. However, this division tends to decrease the quality of the final parameterization. To address this issue, this article proposes a learning based method that simultaneously parameterizes the boundary and interior of a simply connected planar computational domain using a deep neural network. In our approach, we train a neural network to represent the inverse parameterization, which maps the computational domain to the parametric domain, based on the given boundary curve of the planar computational domain. The key component of our neural network is a Multilayer Perceptron (MLP), which takes the coordinates of a point in the computational domain as input and outputs the corresponding parametric values. The loss function of our method comprises three terms. First, it considers the area distortion and angular distortion of the parameterization, which are determined by the Jacobian matrix of the parameterization. Second, it incorporates the boundary difference, quantified by the Hausdorff distance between the boundary of the parametric domain and the image of the inverse mapping of the computational boundary. By optimizing this loss function, we achieve a robust parameterization. Once the network is trained, we employ a tensor-product B-spline function to fit the mapping from the parametric domain to the computational domain. To evaluate the effectiveness of our method, we conduct experiments on the MPEG-7 dataset. The experimental results demonstrate that our approach yields parameterization results with lower distortion and higher bijectivity ratio compared to state-of-the-art approaches.
更多查看译文
关键词
Isogeometric analysis,Domain parameterization,Deep neural network
AI 理解论文
溯源树
样例
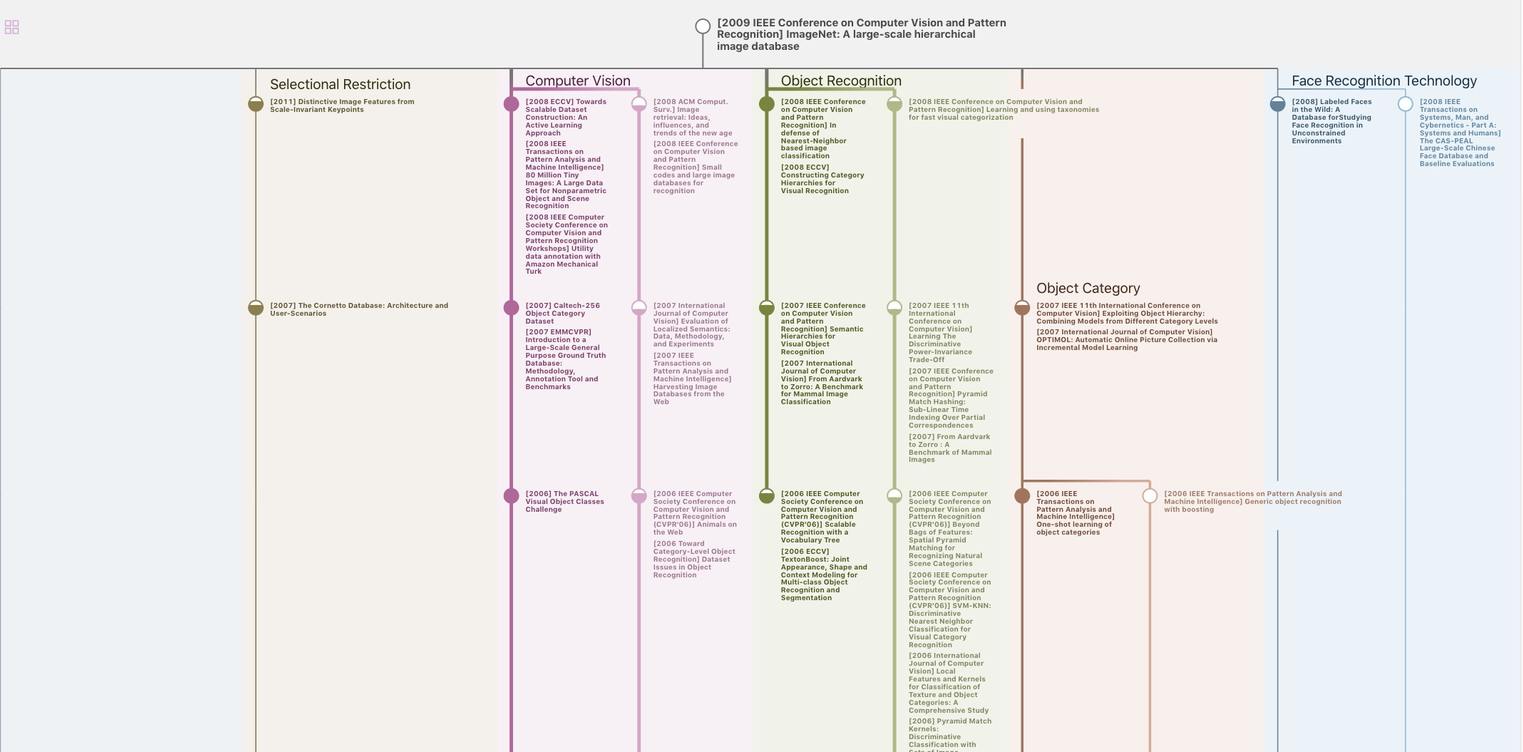
生成溯源树,研究论文发展脉络
Chat Paper
正在生成论文摘要