Learning from fluorescence: A tool for online multiparameter monitoring of a microalgae culture
Computers & Chemical Engineering(2023)
摘要
We propose a systematic approach for monitoring important productivity parameters in a Dunaliella salina cul-ture using 2D fluorescence data. For this purpose, a methodology based on Machine Learning algorithm Pro-jection to Latent Structures Regression (PLSR) coupled with variable selection strategies was used. Additionally, a robustness analysis is proposed to support the validation of the yielded models and provide a measure of their reliability. Robust (i.e., Q2 >= 0.5) and parsimonious (i.e., selecting down to 3 % of the fluorescence variables present in a 250-700 nm wavelength excitation-emission matrix) models were obtained for monitoring cell count, chlorophyll b, total carotenoids and beta-carotene culture concentration, and the ratio between total carotenoids and total chlorophylls, all of which were validated with a left-out batch performing with R2 higher than 0.7 except for beta-carotene (R2 = 0.54).
更多查看译文
关键词
2D fluorescence, Machine learning, Microalgae cultivation, Excitation-emission matrices (EEMs), Bioprocess monitoring, Projection to latent structures regression, (PLSR)
AI 理解论文
溯源树
样例
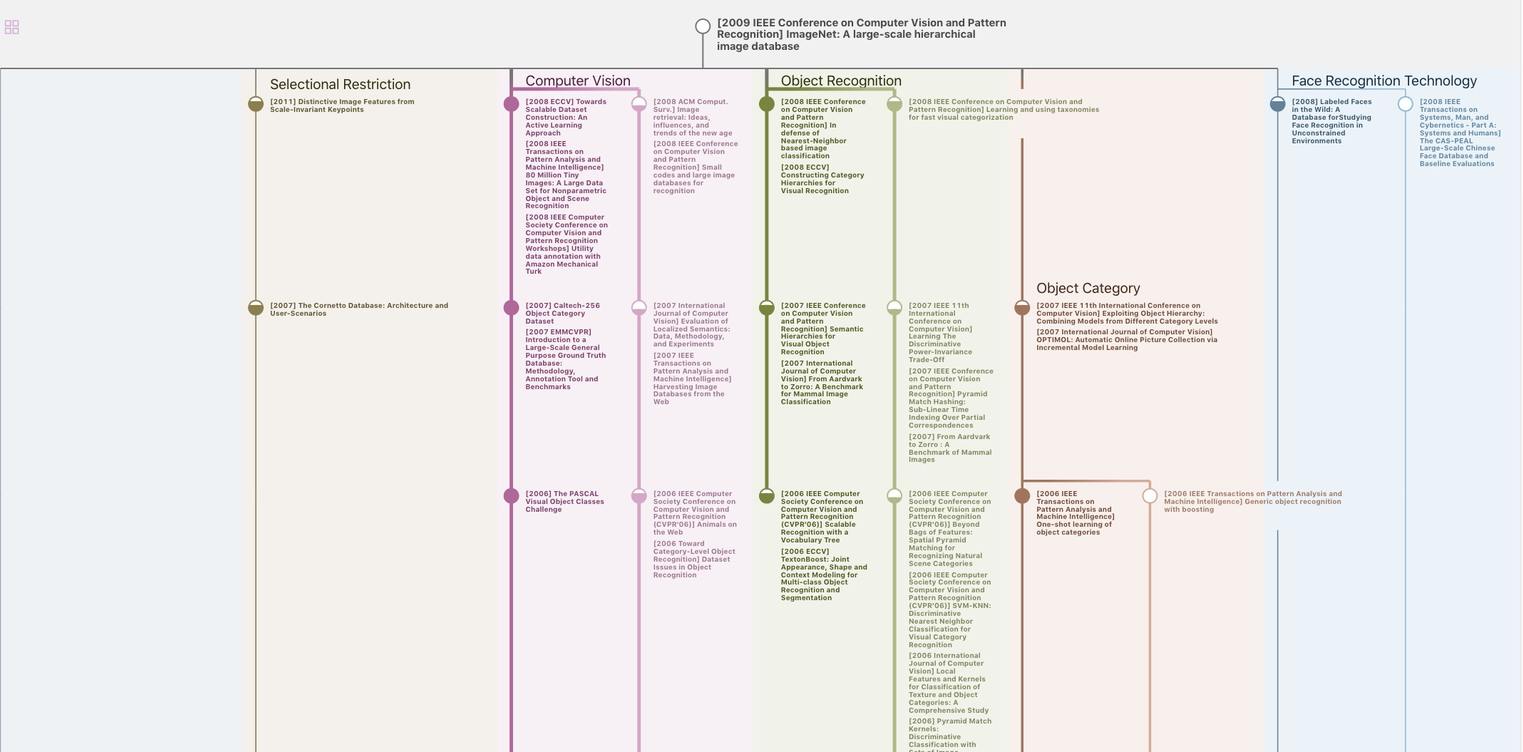
生成溯源树,研究论文发展脉络
Chat Paper
正在生成论文摘要