The emergent role of explainable artificial intelligence in the materials sciences
CELL REPORTS PHYSICAL SCIENCE(2023)
摘要
The combination of rational machine learning with creative materials science makes materials informatics a powerful way of discovering, designing, and screening new materials. However, moving from a promising prediction to a practical strategy often requires more than just an instructive structure-property relationship; understanding how a machine learning method uses the structural feature to predict the target properties becomes critical. Explainable artificial intelligence (XAI) is an emerging field in computer science based in statistics that can augment materials informatics workflows. XAI can be used as a forensic analysis to understand the consequences of data, model, and application decisions or as a model refinement method capable of distinguishing important features from nuisance variables. Here, we outline the state of the art in XAI and highlight methods most useful to the physical sciences. This practical guide focuses on characteristics of XAI methods that are relevant to materials informatics and will become increasingly important as more researchers move toward using deeper neural networks and large language models.
更多查看译文
关键词
explainable artificial intelligence,materials sciences,artificial intelligence
AI 理解论文
溯源树
样例
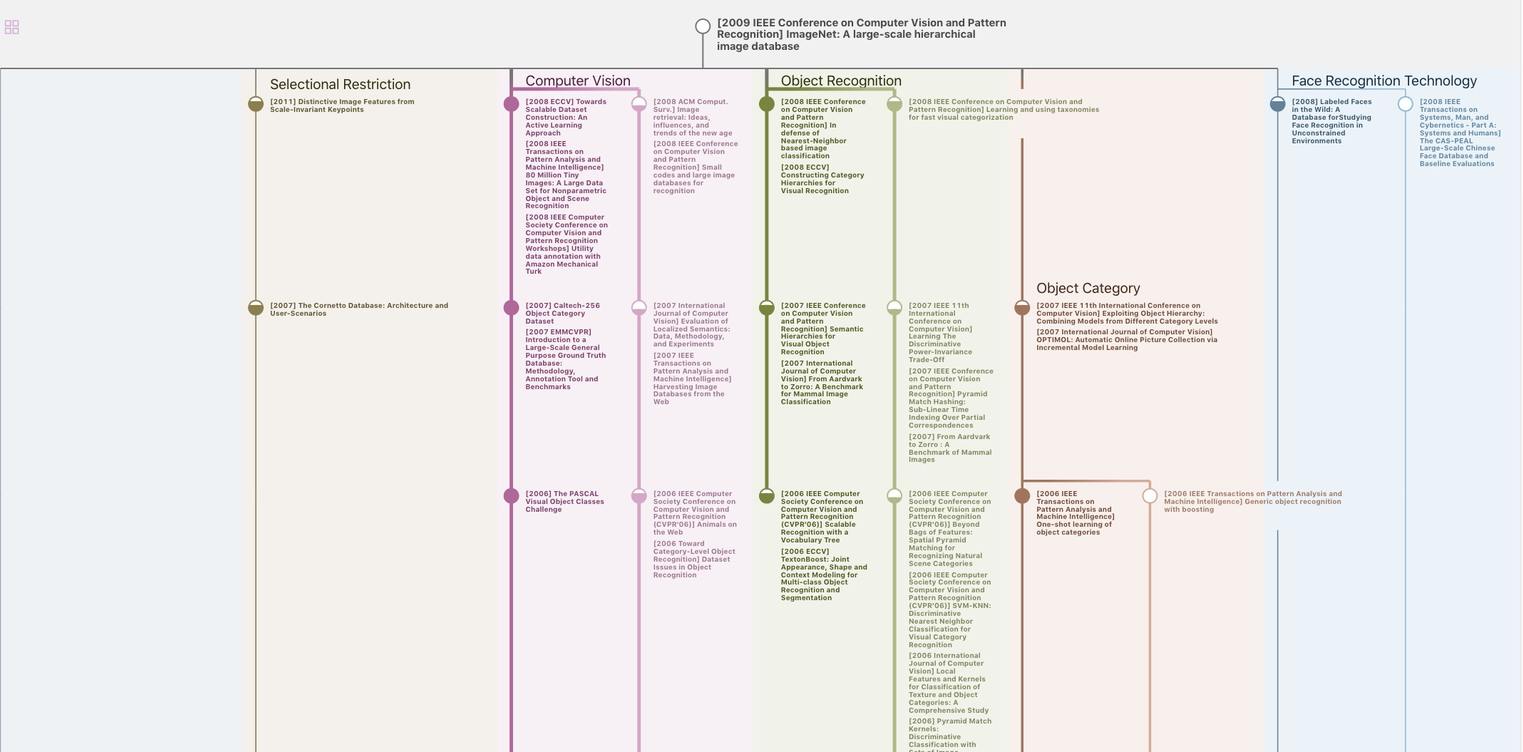
生成溯源树,研究论文发展脉络
Chat Paper
正在生成论文摘要