A novel approach for predicting the concentration of exhaled aerosols exposure among healthcare workers in the operating room
Building and Environment(2023)
摘要
The spread of respiratory infectious diseases in close proximity environments due to poor indoor air quality not only adds to the unease that exists globally, but it also presents a perplexing dilemma when assessing the harmful effects on healthcare providers performing complex surgical interventions. Knowing the exposure concentrations under different conditions is a prerequisite for healthcare providers' safety. This study aimed to develop a methodology by merging experimental data with machine learning techniques. The experiments simulated patients' breathing patterns, examining three independent variables: breathing rate, ceiling air change velocity, and breathing height. The researchers monitored aerosol concentration in six locations within healthcare workers' breathing zone. Experimental data assessed the machine learning models, indicating that the decision tree regression (DTR) was more effective than the support vector machine (SVM) in most positions. Four integrated algorithms, random forest regression (RFR), adaptive boosting (Adaboost), gradient boosting decision tree (GBDT), and extreme gradient boosting (XGboost), utilizing decision tree regression as a weak learner were studied, with RFR model showing the best overall performance. To improve results and enhance credibility, two techniques, multi-feature input and interval prediction, were utilized. The study's outcomes shed light on the potential use of machine learning in predicting aerosol concentrations and ensuring the safety of medical personnel in the operating room.
更多查看译文
关键词
Close environments, Indoor air quality, Airborne transmission, Aerosol, Machine learning, Exposure
AI 理解论文
溯源树
样例
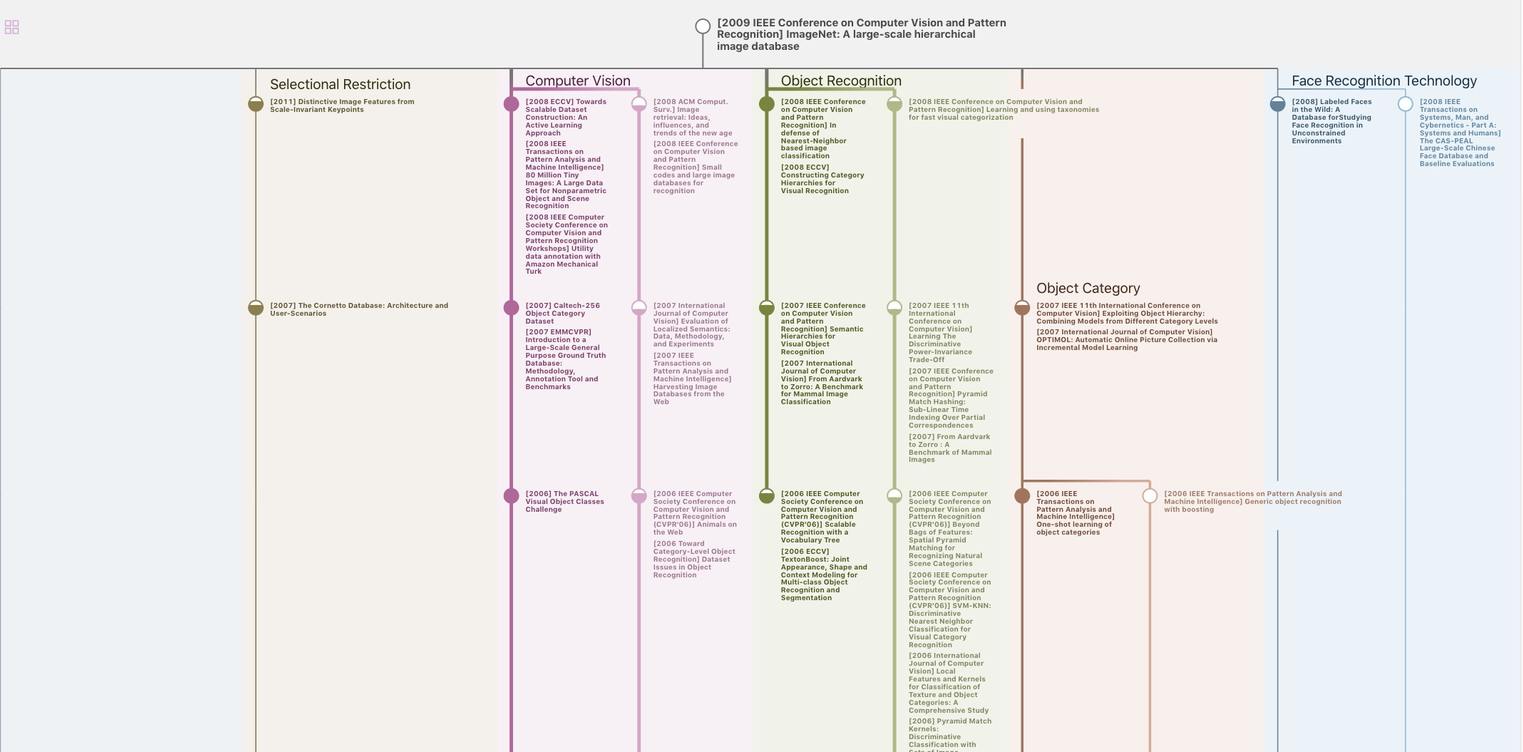
生成溯源树,研究论文发展脉络
Chat Paper
正在生成论文摘要