SCARF: Auto-Segmentation Clinical Acceptability & Reproducibility Framework for Benchmarking Essential Radiation Therapy Targets in Head and Neck Cancer
medRxiv (Cold Spring Harbor Laboratory)(2023)
摘要
Background and Purpose Auto-segmentation of organs at risk (OAR) in cancer patients is essential for enhancing radiotherapy planning efficacy and reducing inter-observer variability. Deep learning auto-segmentation models have shown promise, but their lack of transparency and reproducibility hinders their generalizability and clinical acceptability, limiting their use in clinical settings.
Materials and Methods This study introduces SCARF (auto-Segmentation Clinical Acceptability & Reproducibility Framework), a comprehensive six-stage reproducible framework designed to benchmark open-source convolutional neural networks for auto-segmentation of 19 essential OARs in head and neck cancer (HNC).
Results SCARF offers an easily implementable framework for designing and reproducibly benchmarking auto-segmentation tools, along with thorough expert assessment capabilities. Expert assessment labelled 16/19 AI-generated OAR categories as acceptable with minor revisions. Boundary distance metrics, such as 95th Percentile Hausdorff Distance (95HD), were found to be 2x more correlated to Mean Acceptability Rating (MAR) than volumetric overlap metrics (DICE).
Conclusions The introduction of SCARF, our auto-Segmentation Clinical Acceptability & Reproducibility Framework, represents a significant step forward in systematically assessing the performance of AI models for auto-segmentation in radiation therapy planning. By providing a comprehensive and reproducible framework, SCARF facilitates benchmarking and expert assessment of AI-driven auto-segmentation tools, addressing the need for transparency and reproducibility in this domain. The robust foundation laid by SCARF enables the progression towards the creation of usable AI tools in the field of radiation therapy. Through its emphasis on clinical acceptability and expert assessment, SCARF fosters the integration of AI models into clinical environments, paving the way for more randomised clinical trials to evaluate their real-world impact.
#### Highlights
Highlights
### Competing Interest Statement
Benjamin Haibe-Kains is a shareholder and paid consultant for Code Ocean Inc. There are no conflicts of interest.
### Clinical Protocols
### Funding Statement
This study was funded by Canadian Institutes of Health Research Project-Scheme for the Development and comparison of radiomics models for prognosis and monitoring (Haibe-Kains B, Hope A). Term: 02/2020-01/2023
### Author Declarations
I confirm all relevant ethical guidelines have been followed, and any necessary IRB and/or ethics committee approvals have been obtained.
Yes
The details of the IRB/oversight body that provided approval or exemption for the research described are given below:
A UHN institutional review board approved our study and waived the requirement for informed consent (REB 17-5871); we performed all experiments in accordance with relevant guidelines and ethical regulations of Princess Margaret Cancer Center. Only PM data used for network training was involved in the REB. External publicly available datasets were governed by individual REBs by its institution of origin.
I confirm that all necessary patient/participant consent has been obtained and the appropriate institutional forms have been archived, and that any patient/participant/sample identifiers included were not known to anyone (e.g., hospital staff, patients or participants themselves) outside the research group so cannot be used to identify individuals.
Yes
I understand that all clinical trials and any other prospective interventional studies must be registered with an ICMJE-approved registry, such as ClinicalTrials.gov. I confirm that any such study reported in the manuscript has been registered and the trial registration ID is provided (note: if posting a prospective study registered retrospectively, please provide a statement in the trial ID field explaining why the study was not registered in advance).
Yes
I have followed all appropriate research reporting guidelines and uploaded the relevant EQUATOR Network research reporting checklist(s) and other pertinent material as supplementary files, if applicable.
Yes
更多查看译文
关键词
essential radiation therapy targets,neck,therapy targets,cancer,auto-segmentation
AI 理解论文
溯源树
样例
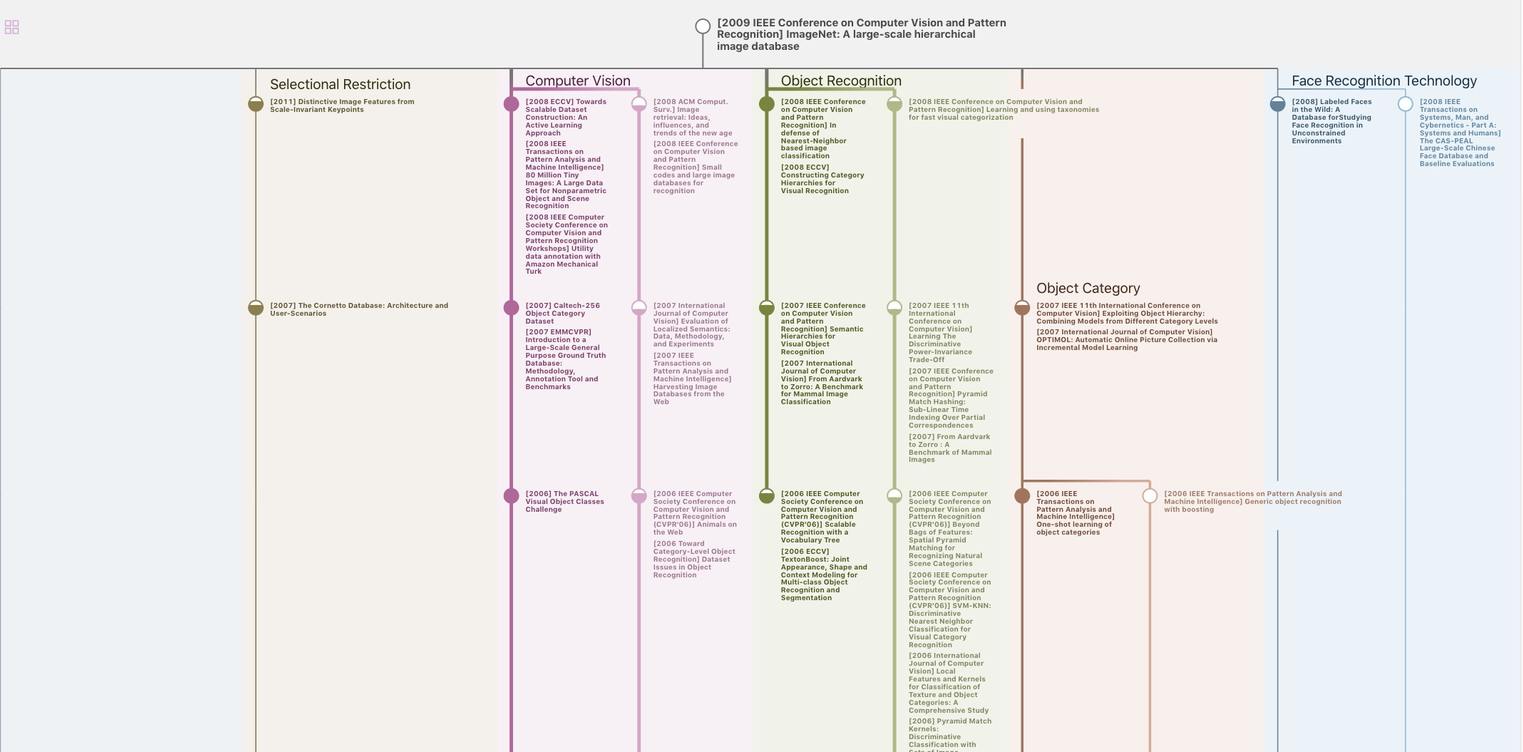
生成溯源树,研究论文发展脉络
Chat Paper
正在生成论文摘要