Relevant Region sampling strategy with adaptive heuristic for asymptotically optimal path planning
Biomimetic Intelligence and Robotics(2023)
摘要
Sampling-based planning algorithm is a powerful tool for solving planning problems in high-dimensional state spaces. In this article, we present a novel approach to sampling in the most promising regions, which significantly reduces planning time-consumption. The RRT# algorithm defines the Relevant Region based on the cost-to-come provided by the optimal forward-searching tree. However, it uses the cumulative cost of a direct connection between the current state and the goal state as the cost-to-go. To improve the path planning efficiency, we propose a batch sampling method that samples in a refined Relevant Region with a direct sampling strategy, which is defined according to the optimal cost-to-come and the adaptive cost-to-go, taking advantage of various sources of heuristic information. The proposed sampling approach allows the algorithm to build the search tree in the direction of the most promising area, resulting in a superior initial solution quality and reducing the overall computation time compared to related work. To validate the effectiveness of our method, we conducted several simulations in both SE(2) and SE(3) state spaces. And the simulation results demonstrate the superiorities of proposed algorithm.
更多查看译文
关键词
Path planning,Asymptotical optimality,Relevant Region,Adaptive heuristic
AI 理解论文
溯源树
样例
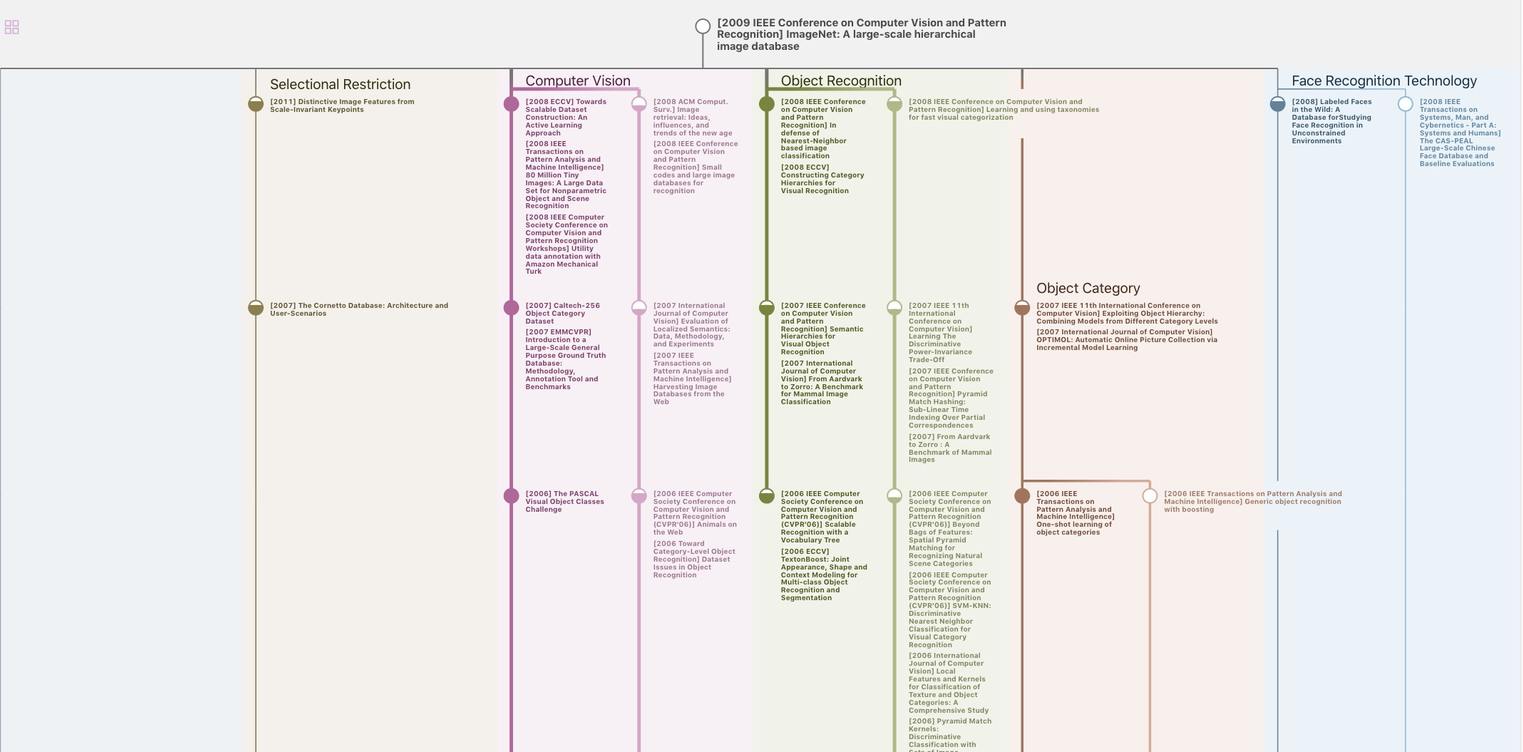
生成溯源树,研究论文发展脉络
Chat Paper
正在生成论文摘要