Towards Dynamic Fuzzy Rule Interpolation via Density-Based Rule Promotion from Interpolated Outcomes
crossref(2023)
摘要
Fuzzy Rule Interpolation (FRI) offers an innovative methodology for inferring outcomes from data points that match no established rules in a sparse fuzzy rule base. Generally, a traditional fuzzy rule-based system suffers from the inability to perform inference while facing an unmatched observation, given a sparse fuzzy rule base that is derived from insufficient data or inadequate human experience and that fails to cover parts of a specific problem domain. Fortunately, starting with a sparse rule base, Fuzzy Rule Interpolation (FRI) can help to formulate interpolated rules specifically in instances where observations fail to activate any existing rule. An interpolated rule gives a result called consequent, which is related to the unmatched observation so that the inference system will not fail to generate an outcome corresponding to the observation as the output. Conventionally, these interpolated rules will be discarded after obtaining outcomes. However, valuable information may be potentially embedded within those discarded rules addressing the limitation regarding the coverage of the original sparse knowledge space. Moreover, without exploiting the potential value of the interpolated rules, the fuzzy inference system based on a sparse rule base will never improve its efficiency and robustness in the long run. Therefore, this underscores the necessity for Dynamic Fuzzy Rule Interpolation (D-FRI), which aims to collate interpolated rules that cover particular knowledge spaces absent in the sparse rule base. Intuitively, those frequently appeared interpolated rules are considered valuable because future observations are more likely to hit the respective problem areas. Promoting selected interpolated rules to the sparse rule base can enhance the overall coverage of the knowledge space and increase the inference efficiency over time. This paper introduces a framework for Dynamic Fuzzy Rule Interpolation that integrates the widely used Transformation-based Fuzzy Rule Interpolation (T-FRI) with an effective form of clustering of interpolated results. Experimental findings validate the effectiveness of this approach.
更多查看译文
AI 理解论文
溯源树
样例
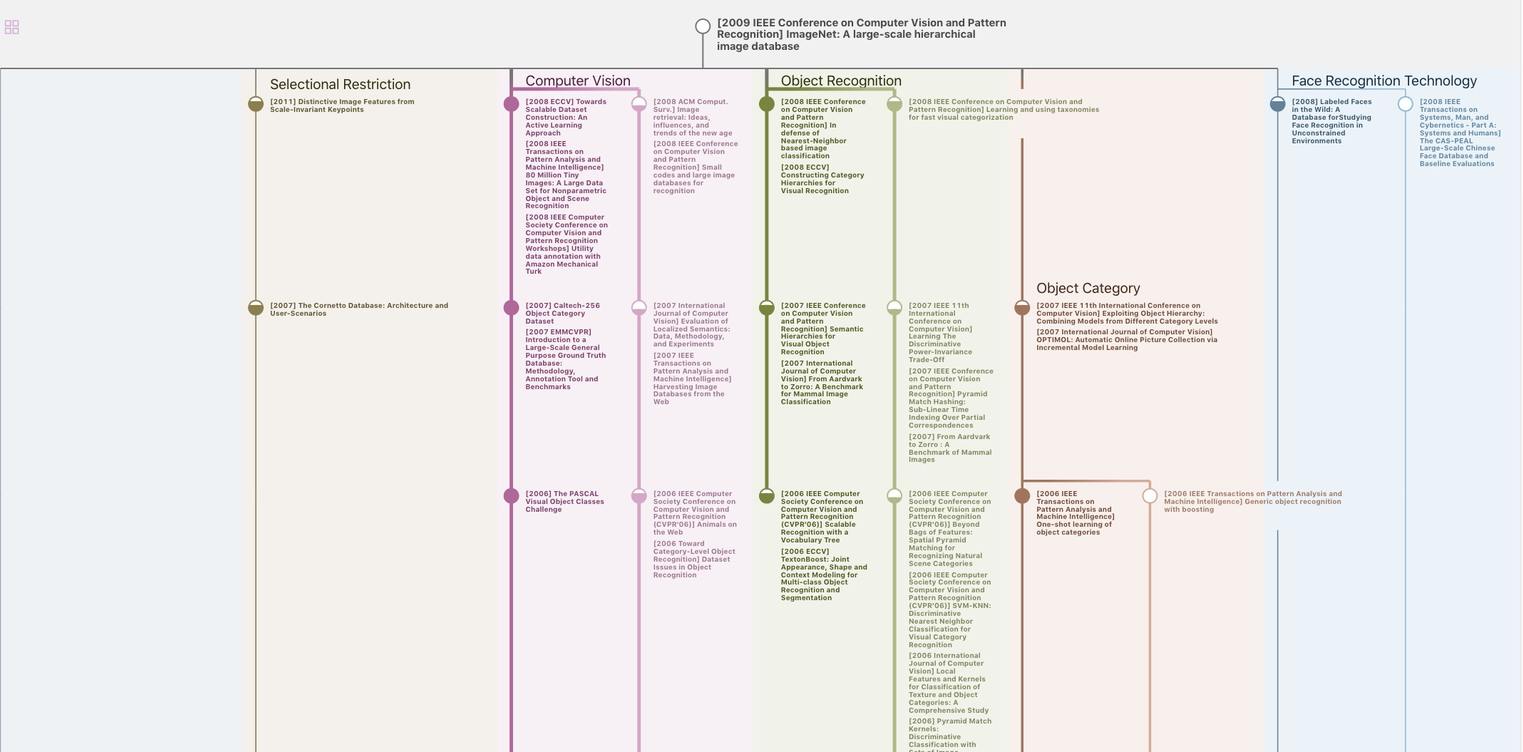
生成溯源树,研究论文发展脉络
Chat Paper
正在生成论文摘要