Identifying Incarceration Status in the Electronic Health Record Using Natural Language Processing in Emergency Department Settings
medRxiv (Cold Spring Harbor Laboratory)(2023)
摘要
Background Incarceration is a highly prevalent social determinant of health associated with high rates of morbidity and mortality and racialized health inequities. Despite this, incarceration status is largely invisible to health services research due to poor electronic health record capture within clinical settings. Our primary objective is to develop and assess natural language processing (NLP) techniques for identifying incarceration status from clinical notes to improve clinical sciences and delivery of care for millions of individuals impacted by incarceration.
Methods We annotated 1,000 unstructured clinical notes randomly selected from the emergency department for incarceration history. Of these annotated notes, 80% were used to train the Longformer-based and RoBERTa NLP models. The remaining 20% served as the test set. Model performance was evaluated using accuracy, sensitivity, specificity, precision, F1 score and Shapley values.
Results Of annotated notes, 55.9% contained evidence for incarceration history by manual annotation. ICD-10 code identification demonstrated accuracy of 46.1%, sensitivity of 4.8%, specificity of 99.1%, precision of 87.1%, and F1 score of 0.09. RoBERTa NLP demonstrated an accuracy of 77.0%, sensitivity of 78.6%, specificity of 73.3%, precision of 80.0%, and F1 score of 0.79. Longformer NLP demonstrated an accuracy of 91.5%, sensitivity of 94.6%, specificity of 87.5%, precision of 90.6%, and F1 score of 0.93.
Conclusion The Longformer-based NLP model was effective in identifying patients’ exposure to incarceration and has potential to help address health disparities by enabling use of electronic health records to study quality of care for this patient population and identify potential areas for improvement.
### Competing Interest Statement
The authors have declared no competing interest.
### Funding Statement
YCCI Doris Duke Charitable Foundation: Fund to Retain Clinical Scientists Fellowship for Medical Student Research from the Yale School of Medicine Office of Student Research.
### Author Declarations
I confirm all relevant ethical guidelines have been followed, and any necessary IRB and/or ethics committee approvals have been obtained.
Yes
The details of the IRB/oversight body that provided approval or exemption for the research described are given below:
This study was approved by the Yale University Institutional Review Board, which waived the need for informed consent. HIC#: 1602017249
I confirm that all necessary patient/participant consent has been obtained and the appropriate institutional forms have been archived, and that any patient/participant/sample identifiers included were not known to anyone (e.g., hospital staff, patients or participants themselves) outside the research group so cannot be used to identify individuals.
Yes
I understand that all clinical trials and any other prospective interventional studies must be registered with an ICMJE-approved registry, such as ClinicalTrials.gov. I confirm that any such study reported in the manuscript has been registered and the trial registration ID is provided (note: if posting a prospective study registered retrospectively, please provide a statement in the trial ID field explaining why the study was not registered in advance).
Yes
I have followed all appropriate research reporting guidelines, such as any relevant EQUATOR Network research reporting checklist(s) and other pertinent material, if applicable.
Yes
All data produced in the present study are available upon reasonable request to the authors.
更多查看译文
关键词
incarceration status,natural language processing,electronic health record,emergency department
AI 理解论文
溯源树
样例
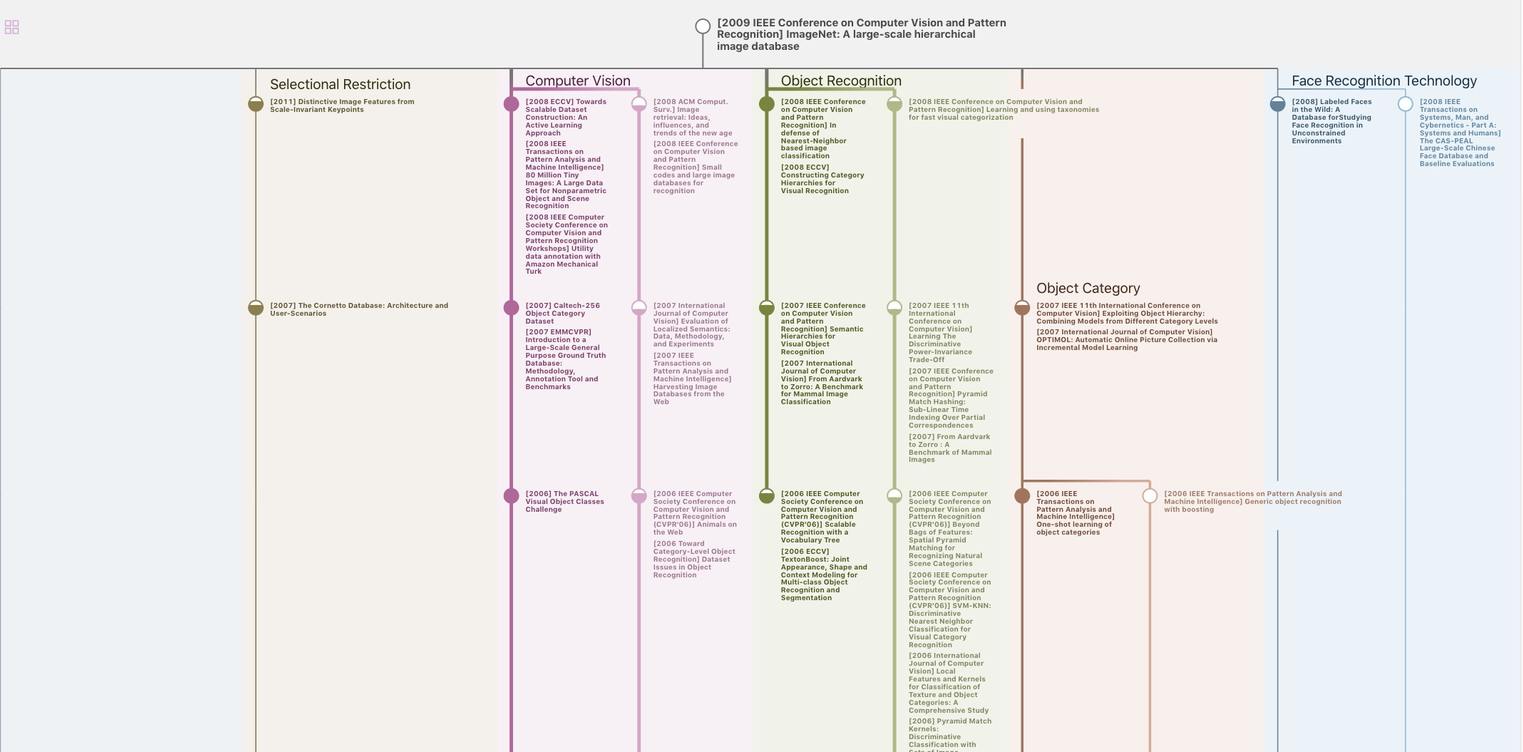
生成溯源树,研究论文发展脉络
Chat Paper
正在生成论文摘要