PAC Prediction Sets Under Label Shift
arXiv (Cornell University)(2023)
摘要
Prediction sets capture uncertainty by predicting sets of labels rather than individual labels, enabling downstream decisions to conservatively account for all plausible outcomes. Conformal inference algorithms construct prediction sets guaranteed to contain the true label with high probability. These guarantees fail to hold in the face of distribution shift, which is precisely when reliable uncertainty quantification can be most useful. We propose a novel algorithm for constructing prediction sets with PAC guarantees in the label shift setting. This method estimates the predicted probabilities of the classes in a target domain, as well as the confusion matrix, then propagates uncertainty in these estimates through a Gaussian elimination algorithm to compute confidence intervals for importance weights. Finally, it uses these intervals to construct prediction sets. We evaluate our approach on five datasets: the CIFAR-10, ChestX-Ray and Entity-13 image datasets, the tabular CDC Heart dataset, and the AGNews text dataset. Our algorithm satisfies the PAC guarantee while producing smaller, more informative, prediction sets compared to several baselines.
更多查看译文
关键词
label,prediction,shift
AI 理解论文
溯源树
样例
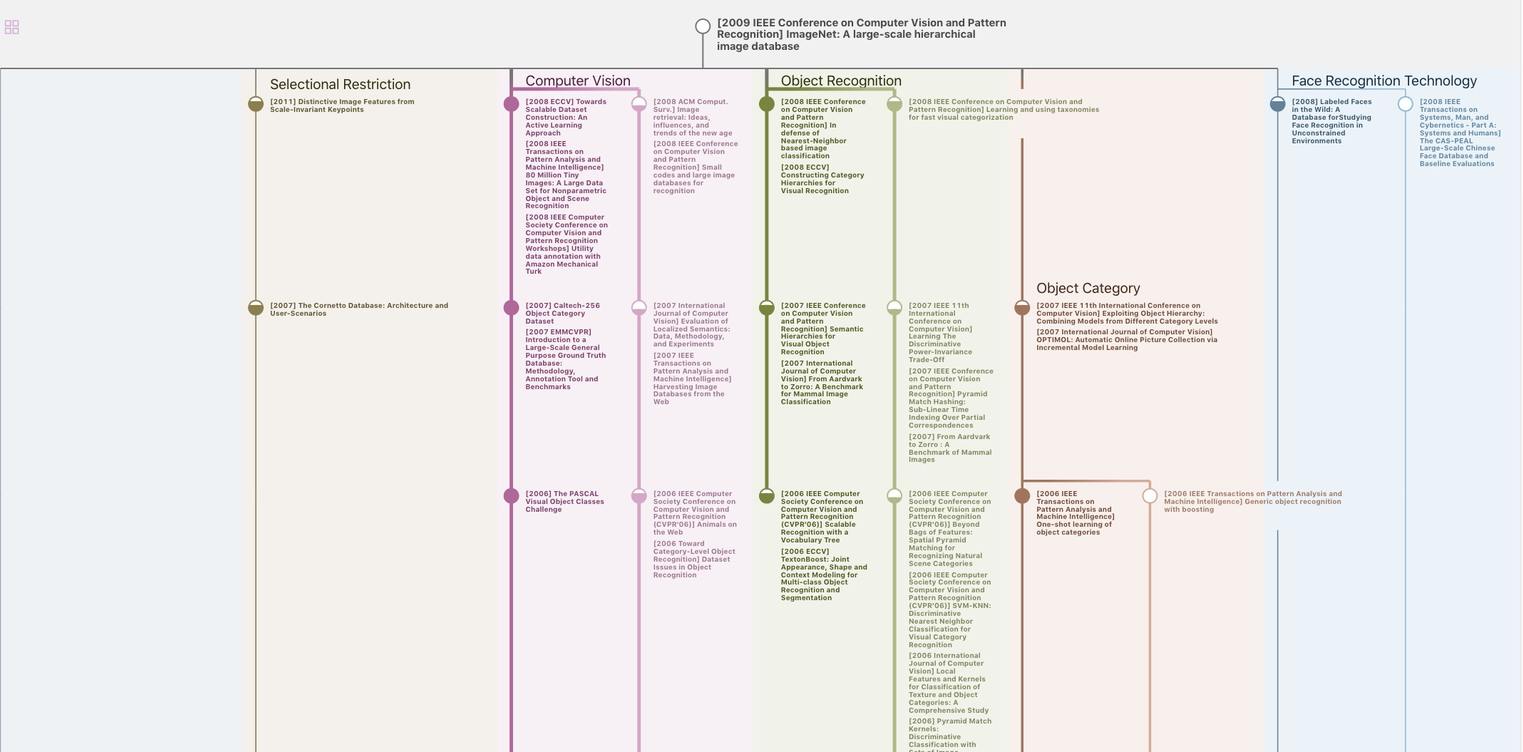
生成溯源树,研究论文发展脉络
Chat Paper
正在生成论文摘要